The Rising Tide of AI in the SaaS Ecosystem
The software-as-a-service (SaaS) industry is witnessing a fundamental shift as artificial intelligence becomes deeply integrated into its framework. White label SaaS AI solutions are emerging as game-changers, allowing businesses to harness sophisticated AI capabilities without building them from scratch. This transformation isn’t just a minor upgrade to existing software delivery models—it represents a complete reimagining of what’s possible in the SaaS space. Companies that previously lacked the technical expertise or resources to develop AI solutions can now offer cutting-edge AI functionality under their own brand. According to recent industry research by Gartner, the AI software market is projected to reach $135 billion by 2025, with white label solutions driving significant portions of this growth. The intersection of white label business models with AI technologies is creating unprecedented opportunities for SaaS providers to expand their service offerings while maintaining brand consistency and customer trust.
Decoding White Label SaaS AI: Core Concepts and Benefits
At its foundation, white label SaaS AI refers to ready-made artificial intelligence solutions that companies can rebrand and sell as their own. Think of it as purchasing a fully-functional AI engine that you can put your logo on and integrate into your existing business infrastructure. These solutions encompass everything from conversational AI for medical offices to sophisticated AI call centers. The primary advantages include dramatically reduced time-to-market, eliminated R&D costs, and access to enterprise-grade AI capabilities that would otherwise require teams of data scientists and engineers. For instance, a customer service software provider can integrate white label AI voice agents to handle call routing and basic customer inquiries, enhancing their product without developing the technology internally. This arrangement creates a win-win scenario where SaaS vendors can focus on their core competencies while still delivering cutting-edge AI features that meet increasingly sophisticated customer expectations.
The Economic Case: Why Businesses Are Flocking to White Label AI
The financial benefits of white label SaaS AI are compelling and multifaceted. Development cost reduction stands as perhaps the most immediate advantage—building proprietary AI systems typically requires millions in investment for talent acquisition, infrastructure, and ongoing maintenance. With white label solutions, these costs shift to a predictable subscription model. A mid-size marketing agency, for example, might pay $10,000-30,000 monthly for a white label AI sales solution they can resell to clients for $2,000-5,000 per client, creating healthy profit margins without upfront development investment. Additionally, time-to-market acceleration provides competitive advantages—while custom AI development typically takes 12-18 months, white label solutions can be implemented in weeks, allowing businesses to capitalize on market opportunities before competitors. This economic equation is particularly attractive for AI calling agencies and service providers looking to expand their technological capabilities without corresponding increases in operational complexity or capital expenditure.
Transformative Applications: Voice AI Revolutionizing Customer Interactions
Voice-based AI represents one of the most transformative white label applications in the current SaaS landscape. AI voice agents are fundamentally changing how businesses handle customer communications by providing natural-sounding, intelligent conversation capabilities that can be deployed across multiple channels. According to industry studies, businesses implementing AI phone services report average cost reductions of 25-40% in their customer service operations while simultaneously improving response times and satisfaction metrics. These solutions go far beyond basic chatbots, offering sophisticated capabilities like sentiment analysis, context awareness, and true conversational intelligence. For example, a real estate agency utilizing AI calling agents for real estate can automatically qualify leads, schedule property showings, and answer common questions—all while maintaining the agency’s brand voice. The technology behind these voice solutions has reached a level of sophistication where many callers cannot distinguish between AI agents and human representatives, creating seamless customer experiences while dramatically reducing operational costs.
Behind the Scenes: The Technical Architecture of White Label AI SaaS
The technological foundation supporting white label AI SaaS solutions is a sophisticated stack comprising several interconnected layers. At its core, most platforms utilize large language models (LLMs) similar to those powering ChatGPT and other generative AI systems, but specifically tuned for particular business domains. These models are typically wrapped in APIs and microservices architecture that allows for seamless integration with existing business systems. Voice-based solutions additionally incorporate advanced speech recognition and text-to-speech technologies such as those from Elevenlabs or Play.ht. The integration layer often includes pre-built connectors to popular CRM systems, calendaring applications, and business intelligence tools. For telephony functionality, many solutions leverage providers like Twilio, though many businesses are now exploring Twilio cheaper alternatives and custom SIP trunking options. This complex architecture is precisely what makes white label solutions valuable—they encapsulate years of development work in ready-to-deploy packages that businesses can implement without needing to understand the underlying technical complexity.
Customization Capabilities: Balancing Standardization and Unique Needs
A common misconception about white label SaaS AI is that it offers limited customization. In reality, today’s solutions provide extensive configuration options that enable businesses to tailor the AI’s behavior, knowledge base, and interaction patterns to match their specific requirements. Most white label providers offer tiered customization capabilities, from simple branding and script adjustments to deep integrations with proprietary systems. For example, businesses using AI voice assistants for FAQ handling can configure knowledge bases with company-specific information, adjust response patterns, and even modify the AI’s personality characteristics to align with brand voice. More sophisticated customization might involve training the AI on proprietary data sets or implementing custom decision trees for specific business workflows. Prompt engineering has emerged as a critical skill in this context, allowing businesses to fine-tune AI behavior without programming expertise. This balance between out-of-the-box functionality and customization flexibility represents one of the key value propositions of white label SaaS AI solutions.
Industry-Specific AI Solutions: From Healthcare to Real Estate
White label SaaS AI is proving particularly valuable in industries with specialized knowledge requirements and repetitive customer interactions. In healthcare, providers are implementing AI bots for medical clinics to handle appointment scheduling, insurance verifications, and preliminary patient information gathering—reducing administrative burdens while improving patient accessibility. Real estate agencies leverage AI appointment setters to qualify leads, schedule property viewings, and provide basic property information, allowing agents to focus on high-value activities. The financial services sector has adopted white label AI for initial client screening, basic advisory services, and routine transaction processing. What makes these industry-specific implementations particularly effective is their ability to incorporate domain knowledge—understanding medical terminology, real estate concepts, or financial regulations—while maintaining the flexibility to adapt to each business’s unique processes. This specialization trend is accelerating as white label providers recognize the value of creating vertical-specific solutions rather than generic AI platforms.
The Reseller Opportunity: Building New Business Models Around AI
A particularly interesting dimension of white label SaaS AI is the emergence of a vibrant reseller ecosystem that’s creating entirely new business models. Entrepreneurs and existing service providers are discovering lucrative opportunities in packaging and reselling white label AI solutions to end clients. For instance, marketing agencies are adding AI sales representatives to their service offerings, while IT consultancies are becoming distributors of AI call center solutions. This trend is supported by platforms like Synthflow AI Whitelabel and Air AI Whitelabel that explicitly design their business models to support multi-tier distribution. The economics can be compelling—resellers typically mark up services by 30-100% while providing valuable configuration, integration, and support services that enhance the core AI offering. For technology consultancies and digital agencies, these AI reseller opportunities represent a way to add high-margin recurring revenue streams with relatively low delivery costs once the initial implementation is complete.
Integration Challenges and Solutions in the White Label Ecosystem
While white label SaaS AI offers numerous advantages, integration complexity remains a significant consideration for businesses adopting these solutions. Connecting AI systems with existing technology stacks—particularly legacy systems—requires careful planning and sometimes custom development work. Common integration points include CRM systems, ERP platforms, communication tools, and data warehouses. Successful implementations typically follow a phased approach, beginning with standalone functionality and gradually increasing integration depth. For example, a business might start with AI appointment scheduling as a discrete function before connecting it to their calendar system, CRM, and eventually their entire customer journey. White label providers are responding to these challenges by developing more robust integration frameworks, including pre-built connectors for popular business systems, webhook support, and comprehensive APIs. Businesses considering white label solutions should carefully evaluate integration capabilities against their existing technology landscape and consider working with implementation partners who bring specific integration expertise.
Data Security and Compliance in White Label AI Implementations
As businesses adopt white label AI solutions, data security and compliance considerations take center stage, particularly for applications handling sensitive customer information. Key concerns include data sovereignty (where information is stored), encryption standards, access controls, and compliance with regulations like GDPR, HIPAA, or industry-specific requirements. White label providers are addressing these concerns through various approaches, including regional data centers, end-to-end encryption, comprehensive audit trails, and compliance certifications. For businesses in regulated industries, it’s crucial to verify that potential white label partners can meet specific compliance requirements. For example, healthcare providers implementing conversational AI for medical offices must ensure HIPAA compliance, while financial services firms need solutions that satisfy banking regulations and data protection standards. The most sophisticated white label providers now offer compliance packages specifically designed for different regulatory environments, simplifying the due diligence process for businesses operating in sensitive sectors.
The Role of Human-AI Collaboration in White Label Solutions
Rather than completely replacing human workers, the most effective white label AI implementations focus on augmenting human capabilities through thoughtful collaboration models. This "human-in-the-loop" approach assigns repetitive, structured tasks to AI systems while keeping humans involved for complex decision-making, exception handling, and relationship building. For instance, an AI call assistant might handle initial call screening and data collection before transferring complex cases to human agents who have immediate access to the information already gathered. Similarly, AI cold callers might conduct initial outreach and qualification before salespeople step in for relationship development and deal closing. Organizations that thoughtfully design these human-AI workflows report significantly higher success rates than those attempting full automation. The key lies in understanding which aspects of customer interactions benefit most from human empathy and judgment versus those where AI consistency and scalability add the most value.
Voice Quality and Natural Language Processing: The Technical Differentiators
The perceived quality of white label voice AI solutions hinges substantially on two technical factors: voice synthesis quality and natural language understanding capabilities. Voice synthesis has advanced dramatically in recent years, moving from robotic-sounding speech to natural, expressive voices with proper intonation and even emotional nuance. Providers like Elevenlabs are setting new standards for voice quality, offering nearly indistinguishable-from-human speech synthesis. Similarly, natural language processing capabilities have evolved from simple keyword recognition to sophisticated understanding of context, intent, and even subtle linguistic nuances. These technical capabilities directly impact customer experience—higher quality voice synthesis leads to greater caller comfort, while advanced language understanding reduces frustration from misunderstandings. When evaluating white label solutions, businesses should pay particular attention to these aspects, as they significantly influence caller perception and ultimately determine whether AI implementations enhance or detract from customer experience.
Measuring ROI: Quantifying the Impact of White Label AI
Businesses implementing white label SaaS AI need robust frameworks for measuring return on investment to justify costs and guide optimization efforts. Key performance indicators typically fall into several categories: operational efficiency (call handling times, query resolution rates, staff productivity), customer experience metrics (satisfaction scores, net promoter scores, positive feedback instances), and financial outcomes (cost savings, revenue generation, conversion rate improvements). For example, a business implementing an AI receptionist might track metrics including abandoned call rates, average time to resolution, appointment booking conversion rates, and staff time saved. Leading organizations are developing increasingly sophisticated ROI models that capture both direct financial impacts and indirect benefits such as improved employee satisfaction (from reduced repetitive work) and enhanced brand perception. The most compelling case studies show multi-dimensional ROI, where white label AI implementations simultaneously reduce costs, improve customer experience, and create new revenue opportunities through expanded service capacity.
Market Players: Key White Label AI Providers and Their Unique Selling Points
The white label SaaS AI landscape features several distinctive providers, each with unique strengths and specializations. Synthflow AI positions itself as a comprehensive solution with particular strength in multi-channel customer communications, offering strong integration capabilities with existing CRM systems. VAPI.ai focuses specifically on voice-based interactions, providing exceptional natural language processing for complex conversations, though businesses seeking alternatives often explore VAPI.ai white label alternatives. Air AI emphasizes ease of implementation and intuitive configuration interfaces, making it accessible to less technical users, while competitors like Air AI white label alternatives may offer more customization depth. Bland AI delivers sophisticated multi-turn conversation capabilities with advanced context retention. And Retell AI specializes in ultra-realistic voice synthesis, though businesses often consider Retell AI whitelabel alternatives for specific use cases. When selecting a provider, businesses should consider factors including integration capabilities with existing systems, customization options, pricing models, and specific strengths relevant to their use cases.
Future Directions: Emerging Trends in White Label AI SaaS
The white label SaaS AI sector is evolving rapidly, with several exciting trends poised to shape its future. Multimodal AI capabilities are expanding beyond voice and text to include visual and interactive elements—imagine an AI agent that can not only discuss products but also show them visually during customer interactions. Emotional intelligence in AI systems is advancing through better sentiment analysis and adaptive response patterns that adjust tone and approach based on customer emotional states. Vertical specialization continues to deepen, with white label solutions increasingly designed for specific industry niches rather than general purposes. Autonomous decision-making capabilities are gradually expanding, with AI systems handling increasingly complex decisions within carefully defined parameters. Perhaps most significantly, LLM customization is becoming more accessible, allowing businesses to fine-tune large language models on proprietary data without massive computational resources, as highlighted in guides about how to create your LLM. These trends collectively point toward white label AI solutions that are increasingly capable, specialized, and autonomous while remaining accessible to non-technical business users.
Case Study: Transforming Customer Service with White Label Voice AI
To illustrate the real-world impact of white label SaaS AI, consider the experience of a mid-sized healthcare provider that implemented an AI voice assistant for their medical office. Before implementation, the practice struggled with high call volumes resulting in approximately 30% of patient calls going to voicemail and a staff primarily occupied with administrative phone tasks rather than in-person patient care. After deploying a white label voice AI solution, the practice experienced dramatic improvements: 24/7 call answering capacity eliminated missed calls, average appointment scheduling time decreased from 4.5 minutes to just 1.2 minutes, and staff reported spending 68% less time on routine phone tasks. Patient satisfaction scores for appointment scheduling increased by 22 points, while the practice realized approximately $12,000 monthly in staff efficiency gains. The implementation process took just three weeks from contract signing to full deployment, with minimal disruption to existing operations. This case exemplifies the transformative potential of white label AI to simultaneously improve operational efficiency, enhance customer experience, and redirect human resources to higher-value activities.
Implementation Roadmap: From Selection to Deployment
Businesses considering white label SaaS AI should follow a structured implementation approach to maximize success probability. The journey typically begins with needs assessment and use case definition, identifying specific business processes where AI can add value. This is followed by vendor evaluation and selection, considering factors such as technical capabilities, integration options, customization flexibility, and pricing structures. Once a vendor is selected, the configuration and customization phase involves adapting the solution to specific business requirements, including creating dialog flows, knowledge bases, and integration connections. Pilot testing with limited scope allows for refinement before full deployment. The training and change management phase prepares staff for new workflows and system interactions. Finally, full deployment and ongoing optimization establish the solution in production while continuing to refine its performance. Throughout this process, businesses should pay particular attention to knowledge transfer from the vendor to ensure internal teams develop the capability to maintain and evolve the solution over time. Resources like guides on how to create an AI call center provide valuable implementation insights.
Ethical Considerations and Transparency in AI Deployment
As white label SaaS AI becomes more prevalent, ethical considerations increasingly influence implementation decisions. Key concerns include transparency with customers (should callers be informed they’re speaking with AI?), fairness and bias mitigation, appropriate handling of edge cases, and responsible data usage. Leading organizations are developing ethical frameworks that guide their AI implementations, including clear policies on disclosure, data retention, and exceptional situation handling. For example, many businesses implementing AI phone agents choose to disclose the automated nature of the interaction upfront, finding that transparency actually increases customer comfort rather than reducing it. Similarly, thoughtful design of escalation paths ensures that complex or sensitive situations are appropriately routed to human agents. As regulatory frameworks for AI continue to develop globally, businesses implementing white label solutions should stay informed about emerging requirements and industry best practices to ensure their deployments remain both effective and ethically sound.
Competitive Differentiation: Standing Out in a White Label World
A common concern with white label solutions is the potential for competitive homogenization—if multiple businesses use the same underlying technology, how can they differentiate themselves? Successful organizations address this challenge through several approaches. Voice and brand personality customization ensures AI interactions reflect the company’s unique tone and values, creating distinctive customer experiences despite shared underlying technology. Workflow and process integration tailors the AI’s behavior to company-specific business processes, creating unique customer journeys. Knowledge base specialization infuses the AI with company-specific expertise and information that competitors don’t possess. Human-AI collaboration models can be designed in ways that leverage the particular strengths of a company’s human team. Finally, complementary service offerings that surround and enhance the AI capability create differentiated value propositions. For example, a real estate agency using AI appointment scheduling might distinguish itself through unique property matching algorithms, virtual tour capabilities, or specialized market knowledge that competitors lack, even if they use similar scheduling technology.
The Future of Work: How White Label AI is Reshaping Business Operations
The proliferation of white label SaaS AI is fundamentally reshaping business operations and workforce composition across industries. Rather than wholesale job displacement, we’re witnessing a reallocation of human talent from repetitive, transactional tasks toward higher-value activities requiring creativity, emotional intelligence, and strategic thinking. Organizations implementing AI solutions typically report that employee satisfaction improves as mundane tasks shift to automated systems. For example, customer service representatives freed from routine inquiries through AI voice conversation systems can focus on complex problem-solving and relationship building, resulting in both higher job satisfaction and greater value creation. Forward-thinking businesses are proactively addressing this transition through reskilling initiatives, creating new roles focused on AI oversight and optimization, and developing career paths that leverage uniquely human capabilities. The most successful implementations position AI as an enabler that enhances human potential rather than a replacement technology, creating a symbiotic relationship between technological capabilities and human creativity.
Elevate Your Business with Intelligent Communication Solutions
As we’ve explored throughout this article, white label SaaS AI is transforming how businesses engage with customers and streamline operations. If you’re ready to harness this technology for your own business, Callin.io provides the perfect entry point into intelligent communication solutions. Our platform allows you to implement AI-powered phone agents that handle incoming and outgoing calls autonomously, creating seamless customer experiences while reducing operational overhead.
Callin.io’s technology enables your business to automate appointment scheduling, answer frequently asked questions, and even close sales through natural, human-like interactions. The flexibility of our platform means you can tailor the AI to represent your brand voice perfectly, ensuring consistent customer experiences across all touchpoints.
Getting started is simple with our free account, which includes test calls and access to our intuitive task dashboard for monitoring interactions. For businesses ready to scale, our subscription plans starting at just $30 USD monthly provide advanced features like Google Calendar integration and built-in CRM functionality. The future of business communication is intelligent, automated, and personalized—and it’s available today through Callin.io.
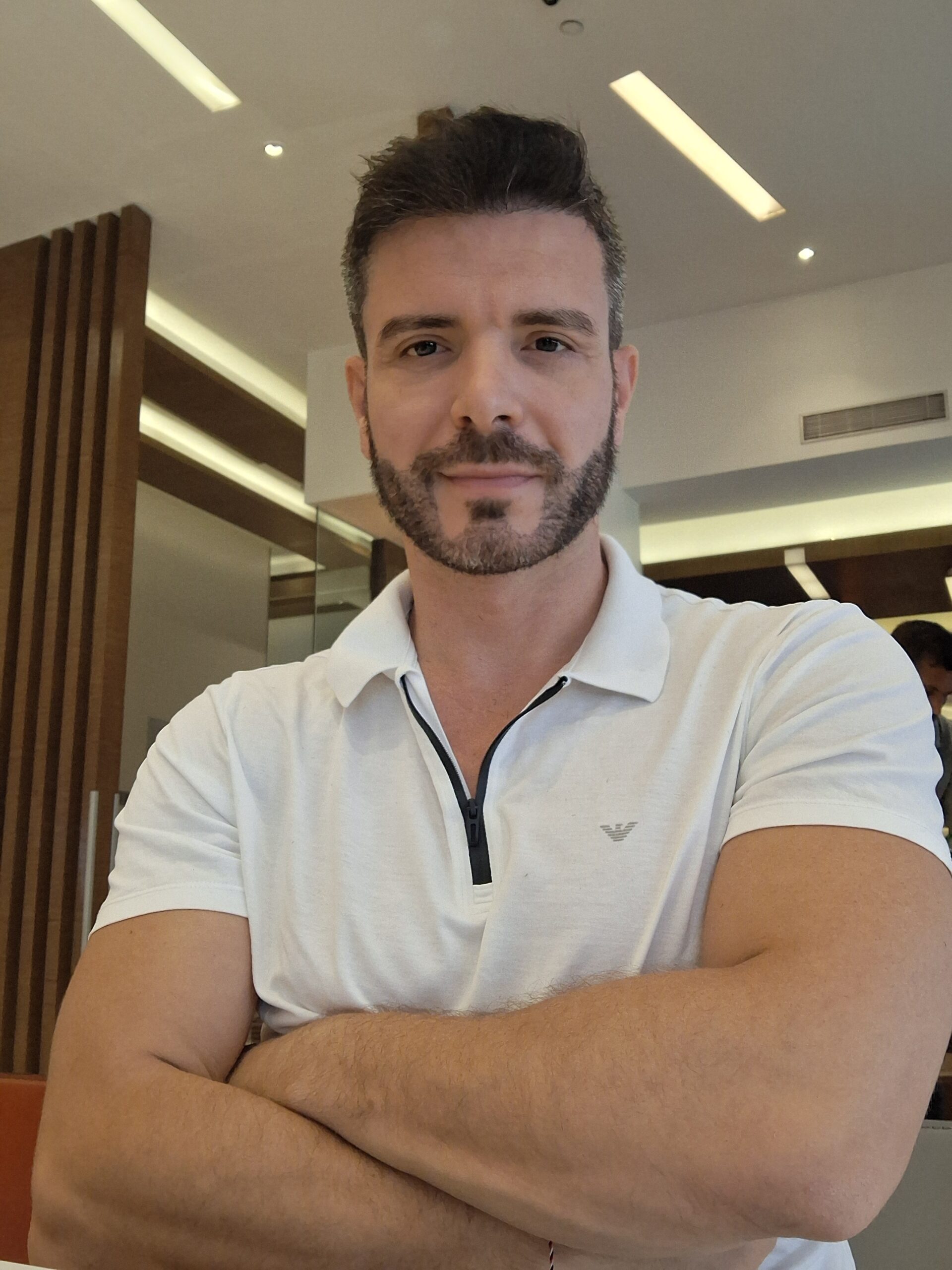
Helping businesses grow faster with AI. 🚀 At Callin.io, we make it easy for companies close more deals, engage customers more effectively, and scale their growth with smart AI voice assistants. Ready to transform your business with AI? 📅 Let’s talk!
Vincenzo Piccolo
Chief Executive Officer and Co Founder