Understanding AI Assistants: The Foundation
In today’s rapidly evolving digital landscape, artificial intelligence assistants have become indispensable tools for businesses and individuals alike. Creating your own AI assistant may seem daunting at first, but with the right approach and understanding, it’s more accessible than ever before. AI assistants are essentially software applications that use natural language processing (NLP), machine learning algorithms, and other AI technologies to understand and respond to human queries. These digital helpers can automate tasks, provide information, and even engage in conversational interactions that feel remarkably human-like. Before diving into the development process, it’s crucial to understand that AI assistants vary in complexity – from simple rule-based chatbots to sophisticated systems like conversational AI for medical offices or virtual receptionists. According to a Stanford University study on AI index, the adoption of AI assistants has increased by 25% annually across various industries, highlighting their growing importance in our digital ecosystem.
Defining Your AI Assistant’s Purpose and Functionality
The first step in creating an effective AI assistant is clearly defining its purpose and scope. Ask yourself: What specific problems will your AI assistant solve? Will it handle AI phone calls for customer service, schedule appointments, answer FAQs, or assist with sales? For instance, if you’re building an AI assistant for a medical practice, you might want it to handle appointment scheduling, prescription refill requests, and basic patient inquiries. If you’re creating an assistant for a retail business, it might focus on product recommendations, order tracking, and customer support. Organizations like Twilio have revolutionized how businesses implement AI assistants by providing platforms that allow for customized functionality. The more precisely you define your assistant’s purpose, the more effectively you can design its features, train its algorithms, and measure its success. This foundational work will guide all subsequent development decisions and help you avoid the common pitfall of creating an assistant that tries to do too much but excels at nothing.
Selecting the Right AI Technologies and Platforms
Choosing the appropriate technology stack is crucial for your AI assistant’s capabilities. For beginners, leveraging existing platforms and APIs is often the most efficient approach rather than building from scratch. Companies like OpenAI, Google Cloud AI, and Microsoft Azure Cognitive Services offer powerful tools and pre-trained models that can be integrated into your assistant. For voice-based assistants, platforms such as ElevenLabs provide advanced text-to-speech capabilities with natural-sounding voices. If you’re looking to implement phone capabilities, Twilio’s conversational AI solutions offer robust frameworks for building voice-enabled assistants. The choice between open-source solutions like Rasa or TensorFlow and commercial platforms depends on your technical expertise, budget constraints, and specific requirements. Open-source options provide greater flexibility and customization potential but require more technical knowledge, while commercial platforms offer streamlined development experiences with professional support. Your selection should align with both your immediate needs and your long-term vision for scaling and enhancing your assistant.
Designing Natural Language Understanding Capabilities
The heart of any effective AI assistant lies in its ability to understand human language in all its nuanced complexity. Natural Language Understanding (NLU) enables your assistant to interpret user inputs, discern intent, and extract meaningful information from conversations. This involves implementing intent recognition systems that can identify what users are trying to accomplish, entity extraction algorithms that recognize specific pieces of information (like dates, names, or locations), and sentiment analysis to gauge emotional context. Tools like Hugging Face’s transformers library provide pre-trained models that excel at these tasks, while platforms such as DialogFlow offer user-friendly interfaces for designing conversational flows. When developing an AI voice assistant for FAQ handling, for instance, you’ll need to anticipate various ways users might phrase the same question and train your system accordingly. The quality of your NLU implementation directly impacts how intuitive and helpful your assistant feels to users, making this one of the most critical aspects of development to invest time and resources in perfecting.
Crafting Engaging Conversational Experiences
Creating natural-sounding dialogues is essential for user engagement with your AI assistant. Effective conversational design involves more than just accurate responses; it requires personality development, appropriate tone setting, and conversational flow management. When designing your assistant’s personality, consider your brand voice and target audience—should it be formal, casual, humorous, or compassionate? For medical applications, a calm and professional tone might be appropriate, while a retail assistant might adopt a more enthusiastic approach. Implement context management to maintain conversational coherence, allowing your assistant to remember earlier parts of the conversation and refer back to them naturally. For example, in AI voice conversations, the ability to maintain context throughout a call significantly enhances user experience. Incorporate mechanisms for handling misunderstandings gracefully, such as clarification requests and fallback responses. Resources like Nielsen Norman Group’s conversational design guidelines provide valuable insights into creating engaging dialogue flows. Remember that conversations should feel efficient yet natural, striking a balance between getting to the point and maintaining a human-like interaction style.
Implementing Voice and Speech Technologies
For assistants that communicate through voice, integrating high-quality speech technologies is crucial. This involves both speech recognition (converting spoken language to text) and speech synthesis (converting text to spoken language). Modern speech recognition systems like Google’s Speech-to-Text API or Amazon Transcribe offer impressive accuracy across multiple languages and accents. For speech synthesis, services like Play.ht enable your assistant to respond with natural-sounding voices that can convey appropriate emotion and emphasis. When implementing voice capabilities, consider factors such as noise handling, speaker identification, and multilingual support based on your target users’ needs. Voice latency—the delay between user input and assistant response—should be minimized for a fluid conversation experience. For comprehensive voice integration, platforms like Callin.io’s AI voice agent provide end-to-end solutions that handle both incoming and outgoing calls with natural conversational capabilities. According to research from MIT’s Media Lab, voice interactions can create stronger emotional connections with users than text-based interfaces, making this investment particularly valuable for customer-facing applications.
Building Knowledge Bases and Information Resources
An AI assistant is only as helpful as the information it can access. Developing comprehensive knowledge bases equips your assistant to provide accurate, relevant responses to user queries. Start by identifying authoritative sources related to your assistant’s domain—whether that’s product specifications, company policies, industry regulations, or general information. Structure this information in a way that’s easily retrievable, using knowledge graphs, databases, or vector embeddings to represent relationships between concepts. For specialized applications like AI calling agents for real estate, include domain-specific information about properties, market trends, and common buyer questions. Implement regular update mechanisms to ensure your knowledge base stays current, particularly for domains where information changes frequently. Consider leveraging existing knowledge sources through APIs for general information—services like Wolfram Alpha, Wikipedia, or industry-specific databases can supplement your custom knowledge base. The best AI assistants combine proprietary information with broader knowledge resources, creating a seamless experience where users don’t need to distinguish between different information sources.
Integrating with External Systems and APIs
To maximize utility, your AI assistant should connect seamlessly with other systems and services. API integration allows your assistant to perform actions beyond conversation, such as checking inventory, processing payments, updating records, or scheduling appointments. For a complete AI appointment scheduler, integration with calendar systems like Google Calendar or Microsoft Outlook is essential. Similarly, AI calling bots for health clinics need secure connections to patient management systems. When planning integrations, prioritize systems that support your core use cases, then expand to enhance functionality over time. Implement proper authentication and security measures, particularly when handling sensitive data like personal health information or payment details. Use webhook capabilities to enable real-time notifications and updates between systems. For telephony functions, integration with providers like Twilio or alternatives such as SIP trunking services creates powerful voice capabilities. Well-executed integrations transform your assistant from a simple conversational interface into a powerful automation tool that can take meaningful actions on behalf of users, significantly increasing its practical value.
Designing User Interfaces and Interaction Channels
Although conversational interfaces are central to AI assistants, the channels through which users interact with your assistant require careful consideration. Whether implementing your assistant as a chatbot on your website, a voice system accessible by phone, a mobile app, or integrated into messaging platforms like WhatsApp or Slack, each channel has unique design requirements. For web interfaces, ensure responsive design that works across devices and consider adding visual elements like typing indicators to create a more engaging experience. For AI phone services, design clear voice menus and natural interaction patterns that guide users effectively. Multi-channel assistants should maintain consistent personality and capabilities across platforms while optimizing for each channel’s strengths. Tools like Callin.io provide white-label solutions that can be customized to match your brand identity across various interaction channels. According to the International Journal of Human-Computer Studies, interface design significantly impacts user trust and satisfaction with AI assistants, making this an essential investment rather than an afterthought.
Training Your AI Assistant with Quality Data
The performance of your AI assistant depends largely on the quality and quantity of training data used to develop its understanding. For supervised learning approaches, curate diverse datasets of example conversations, questions, and responses relevant to your domain. These datasets should represent the full spectrum of user intents, phrasing variations, and edge cases your assistant might encounter. For specialized assistants like AI sales representatives, include training examples that reflect effective sales techniques and common customer objections. Implement a feedback loop where real user interactions continuously improve your assistant’s capabilities through techniques like reinforcement learning from human feedback (RLHF). Tools like Prodigy facilitate efficient data annotation and labeling. Consider synthetic data generation to augment your training sets, particularly for uncommon scenarios that are important to handle correctly. Be vigilant about potential biases in your training data that could lead to unfair or inappropriate responses—platforms like IBM’s AI Fairness 360 can help identify and mitigate such issues. Remember that training is an ongoing process rather than a one-time effort, with performance improvements directly tied to continued data refinement.
Implementing Personalization and Context Awareness
Advanced AI assistants distinguish themselves by offering personalized experiences tailored to individual users. Implement user profiling systems that securely store relevant information about each user’s preferences, history, and needs. For AI call center applications, this might include customer purchase history, previous issues, and communication preferences. Context management systems should maintain conversation state across multiple interactions, allowing your assistant to reference past conversations naturally. Location awareness can be valuable for providing regionally relevant information or services. Recommendation engines can suggest products, content, or actions based on user behavior patterns and preferences. When implementing personalization, balance usefulness with privacy—always be transparent about what data you’re collecting and how it’s being used, and comply with regulations like GDPR or CCPA. Platforms like Cartesia AI offer sophisticated personalization capabilities while maintaining proper data governance. Research from Accenture indicates that personalized AI interactions can increase customer satisfaction by up to 40%, making this capability a significant competitive advantage.
Ensuring Privacy, Security, and Ethical Use
Building trust requires rigorous data protection and ethical AI practices. Implement end-to-end encryption for sensitive communications, particularly for applications like medical office conversational AI that handle protected health information. Clearly communicate your data retention policies and give users control over their personal information. Conduct regular security audits and penetration testing to identify vulnerabilities. Beyond technical security, consider broader ethical implications of your AI assistant—avoid perpetuating biases, ensure accessibility for users with disabilities, and design with transparency so users understand when they’re interacting with AI. For conversations on sensitive topics, implement content moderation systems to prevent harmful or inappropriate responses. Resources like the IEEE’s Ethically Aligned Design guidelines can help navigate complex ethical questions. Remember that privacy breaches or ethical lapses can irreparably damage user trust, so these considerations should be foundational to your development process rather than afterthoughts. Organizations like AI Now Institute provide valuable frameworks for responsible AI development that can guide your approach to these critical issues.
Testing and Quality Assurance Strategies
Thorough testing is essential before deploying your AI assistant to real users. Implement a multi-layered testing strategy that includes unit testing of individual components, integration testing of connected systems, and end-to-end testing of complete user journeys. For conversational testing, use both automated approaches and human evaluators to assess understanding accuracy, response appropriateness, and natural dialogue flow. Conduct performance testing to ensure your assistant can handle expected user loads without significant latency. For AI calling applications, test across different network conditions and phone systems to ensure reliable voice quality. Implement A/B testing to compare different conversation designs or response strategies. Tools like Botium can automate conversational testing at scale. Include diverse testers who represent your target user demographics to identify usability issues or cultural misunderstandings. Establish clear quality metrics like task completion rate, understanding accuracy, and user satisfaction to objectively measure your assistant’s performance. Remember that even after launch, ongoing monitoring and testing remain essential as user behaviors and expectations evolve over time.
Deployment and Scaling Considerations
When ready to deploy, choose infrastructure that balances performance, cost, and scalability. For cloud deployment, platforms like AWS, Google Cloud, or Microsoft Azure offer flexible resources that can scale with demand. If handling sensitive information in regulated industries, you might need dedicated or hybrid cloud solutions to meet compliance requirements. Implement proper monitoring systems to track performance metrics, error rates, and usage patterns, with automated alerts for potential issues. For voice applications like AI call centers, ensure sufficient telephony infrastructure to handle peak call volumes without degradation. Consider geographic distribution of resources to minimize latency for global users. Implement graceful degradation strategies so your assistant can continue providing basic functionality even during partial system failures. Tools like Kubernetes can help manage containerized deployments across diverse environments. For businesses exploring white-label solutions, platforms like Synthflow AI or Retell AI alternatives offer scalable infrastructure with reduced technical complexity. According to Gartner research, more than 50% of organizations underestimate the infrastructure requirements for AI projects, emphasizing the importance of proper capacity planning before deployment.
Measuring Success and Continuous Improvement
Establishing clear key performance indicators (KPIs) is essential for evaluating your AI assistant’s impact and guiding improvements. Common metrics include task completion rate, conversation length, user satisfaction scores, containment rate (percentage of inquiries handled without human intervention), and business outcomes like conversion rates or cost savings. For AI sales applications, track metrics like lead qualification accuracy and sales conversion rates. Implement user feedback mechanisms—both explicit (ratings, surveys) and implicit (usage patterns, abandonment points)—to identify improvement opportunities. Analyze conversation logs to identify common failure points or user frustrations. Regular A/B testing of new features or conversation designs can provide data-driven guidance for enhancements. Consider establishing a benchmark against human performance for key tasks to measure your assistant’s progress over time. Tools like Dashbot or custom analytics dashboards can help visualize performance trends. Remember that improvement should be continuous rather than periodic—the most successful AI assistants evolve constantly based on user interactions and feedback, gradually expanding their capabilities while refining existing functions.
Advanced Features: Multilingual and Multimodal Capabilities
As your AI assistant matures, consider expanding its capabilities to serve diverse user needs. Multilingual support allows your assistant to communicate in users’ preferred languages, significantly expanding your potential user base. Services like Google Cloud Translation or DeepL API can be integrated for accurate translations. For specialized domains, language-specific training may be necessary to capture nuanced terminology correctly. Beyond language expansion, consider multimodal capabilities that allow your assistant to understand and generate content across different formats—processing images, creating visualizations, or understanding vocal emotions in addition to words. For applications like AI voice agents, the ability to detect emotional cues in speech can enable more empathetic responses. For retail or visual applications, image recognition capabilities allow users to show rather than tell what they’re looking for. These advanced features should be prioritized based on user needs rather than implemented simply because they’re technologically possible. Resources like You.com demonstrate how multimodal capabilities can create distinctively powerful user experiences when thoughtfully implemented around specific use cases.
Customization and White-Labeling Options
For businesses looking to implement AI assistants without extensive in-house development, white-label solutions offer a compelling alternative. These platforms provide pre-built AI capabilities that can be customized with your branding, knowledge base, and specific functionality requirements. Services like AIR AI white-label and VAPI AI white-label offer turnkey solutions for implementing sophisticated AI assistants without starting from scratch. When evaluating white-label options, consider customization flexibility, integration capabilities, pricing models, and available support. For specific applications like AI receptionists or AI appointment setters, look for platforms with proven success in those domains. White-label solutions typically accelerate time-to-market and reduce development costs, though they may offer less differentiation than fully custom-built assistants. For businesses entering the AI space as service providers themselves, reseller AI caller programs provide opportunities to offer AI communication services under your own brand. According to McKinsey research, white-label AI solutions can reduce implementation time by up to 60% compared to building custom solutions, making them particularly attractive for businesses seeking rapid deployment.
AI Assistant Management and Operational Best Practices
Successfully operating an AI assistant requires ongoing management and optimization. Establish clear governance structures defining who’s responsible for different aspects of your assistant’s performance—from technical maintenance to content approvals and conversation design updates. Create detailed documentation of your assistant’s capabilities, limitations, and operational procedures to facilitate knowledge transfer and consistent management. Implement proper version control for your assistant’s knowledge base and conversation flows, allowing you to roll back changes if needed. Develop escalation protocols for situations your assistant can’t handle, ensuring smooth transitions to human agents when necessary. For call center voice AI applications, integrate with human agent systems to enable seamless handoffs. Schedule regular reviews of user interactions to identify improvement opportunities and evolving user needs. Consider establishing a dedicated team responsible for your assistant’s ongoing development rather than treating it as a one-time project. Tools like DeepSeek can help analyze conversation patterns to identify optimization opportunities. According to Deloitte, organizations with formal AI governance structures report 37% higher satisfaction with their AI implementations, highlighting the importance of well-defined management practices.
Prompt Engineering for Optimal Assistant Performance
For AI assistants built on large language models (LLMs), prompt engineering is a critical skill that dramatically impacts performance. Effective prompts establish clear context, constraints, and expectations for your assistant, guiding it toward desired responses. When designing system prompts that define your assistant’s core behavior, include explicit instructions about tone, response format, and handling of sensitive topics. For specialized applications like AI cold callers, carefully crafted prompts can encode sales techniques and conversation strategies. Implement prompt templates for common scenarios to ensure consistent handling of similar situations. Test different prompt formulations against benchmark examples to identify optimal approaches, and document successful patterns for future reference. Resources like Callin.io’s prompt engineering guide provide domain-specific insights for voice applications, while general resources like OpenAI’s prompt engineering guide offer broader principles. Consider implementing prompt management systems that allow non-technical team members to modify prompts within controlled parameters. According to research from Stanford’s CRFM, well-engineered prompts can improve task performance by 20-30% without any changes to the underlying model, making this skill a high-leverage investment for AI assistant developers.
Case Studies: Success Stories and Lessons Learned
Learning from real-world implementations can provide valuable insights for your own AI assistant development. Consider how medical offices have successfully implemented conversational AI to handle appointment scheduling and patient inquiries, reducing administrative burden while improving patient satisfaction. Analyze how companies like Bland AI have created white-label solutions that enable businesses to rapidly deploy customized AI voice agents. Study the approaches of AI phone consultants who have successfully created natural-sounding voice interactions that customers find helpful rather than frustrating. The evolution of virtual secretary services demonstrates how AI assistants can transform from simple answering services to comprehensive business operations support. Review challenges faced by early implementers, such as misaligned expectations or insufficient training data, to avoid repeating common mistakes. For sector-specific insights, examine how AI is transforming sales processes or how AI appointment booking bots are changing customer service dynamics. Case studies published by organizations like MIT Technology Review and Harvard Business Review provide well-documented examples with measured outcomes that can inform your strategy and implementation approach.
Future-Proofing Your AI Assistant
The field of artificial intelligence evolves rapidly, requiring strategic planning to ensure your assistant remains relevant and effective. Design your architecture with modularity in mind, allowing individual components to be upgraded without rebuilding the entire system. Stay informed about emerging AI technologies and standards through resources like Hugging Face, arXiv, and industry conferences. Consider implementing multimodal capabilities now, even if primarily used for text or voice initially, as user expectations are increasingly shifting toward rich, diverse interaction models. For voice applications, follow evolving standards in text-to-speech technology to maintain natural-sounding interactions. Plan for regular model updates and retraining cycles to incorporate new data and capabilities. Build flexibility into your knowledge management systems to easily incorporate new information sources or domains. Consider potential regulatory developments, particularly around privacy, transparency, and AI accountability, and design systems that can adapt to changing compliance requirements. For businesses building on platforms like Twilio, stay aware of platform evolution and alternative options like cheaper Twilio alternatives to manage costs effectively. According to PwC research, organizations that proactively adapt their AI strategies to emerging technologies realize 5-15% greater return on AI investments compared to reactive approaches.
Transforming Your Business with Intelligent Automation
The journey of building an AI assistant extends beyond technical implementation to fundamentally transforming how your business operates. When properly integrated, AI assistants can reshape customer engagement models, streamline operations, and enable new service offerings. Consider how starting an AI calling agency represents a new business model enabled by accessible AI technology. For customer service operations, AI call assistants can dramatically reduce wait times while maintaining consistent quality across all interactions. In sales contexts, AI pitch setters can qualify leads and initiate conversations that human representatives then cultivate into relationships. Look beyond obvious applications to identify unique opportunities within your business—perhaps using virtual call power to reach previously underserved market segments or implementing AI sales generators to create personalized outreach at scale. Measure both quantitative metrics like cost savings and qualitative improvements like employee satisfaction as repetitive tasks shift to AI handling. Organizations like McKinsey estimate that businesses successfully implementing AI assistants realize 3-5x return on investment through combined cost reduction and revenue enhancement. The most successful implementations treat AI assistants as partners in transformation rather than simply technological tools, using them to catalyze broader organizational innovation and evolution.
Take Your Business Communications to the Next Level
Building an AI assistant represents a significant opportunity to transform how your business engages with customers and manages operations. Whether you’re looking to implement conversational AI platforms for customer service, develop AI appointment schedulers for efficient booking, or create AI sales representatives to nurture leads, the journey begins with clear goals and thoughtful implementation. While this guide provides a comprehensive framework, each business has unique needs and challenges that require customized approaches. If you’re ready to implement AI voice technology in your business without the complexity of building from scratch, consider exploring purpose-built solutions.
If you’re looking to manage your business communications efficiently and effectively, I recommend exploring Callin.io. This platform allows you to implement AI-powered phone agents that autonomously handle inbound and outbound calls. With their innovative AI phone agent, you can automate appointments, answer frequently asked questions, and even close sales, interacting naturally with customers.
The free account on Callin.io offers an intuitive interface for setting up your AI agent, with test calls included and access to the task dashboard to monitor interactions. For those seeking advanced features, such as Google Calendar integrations and built-in CRM, subscription plans starting at $30 USD per month are available. Discover more about Callin.io and take your first step toward intelligent business communication today.
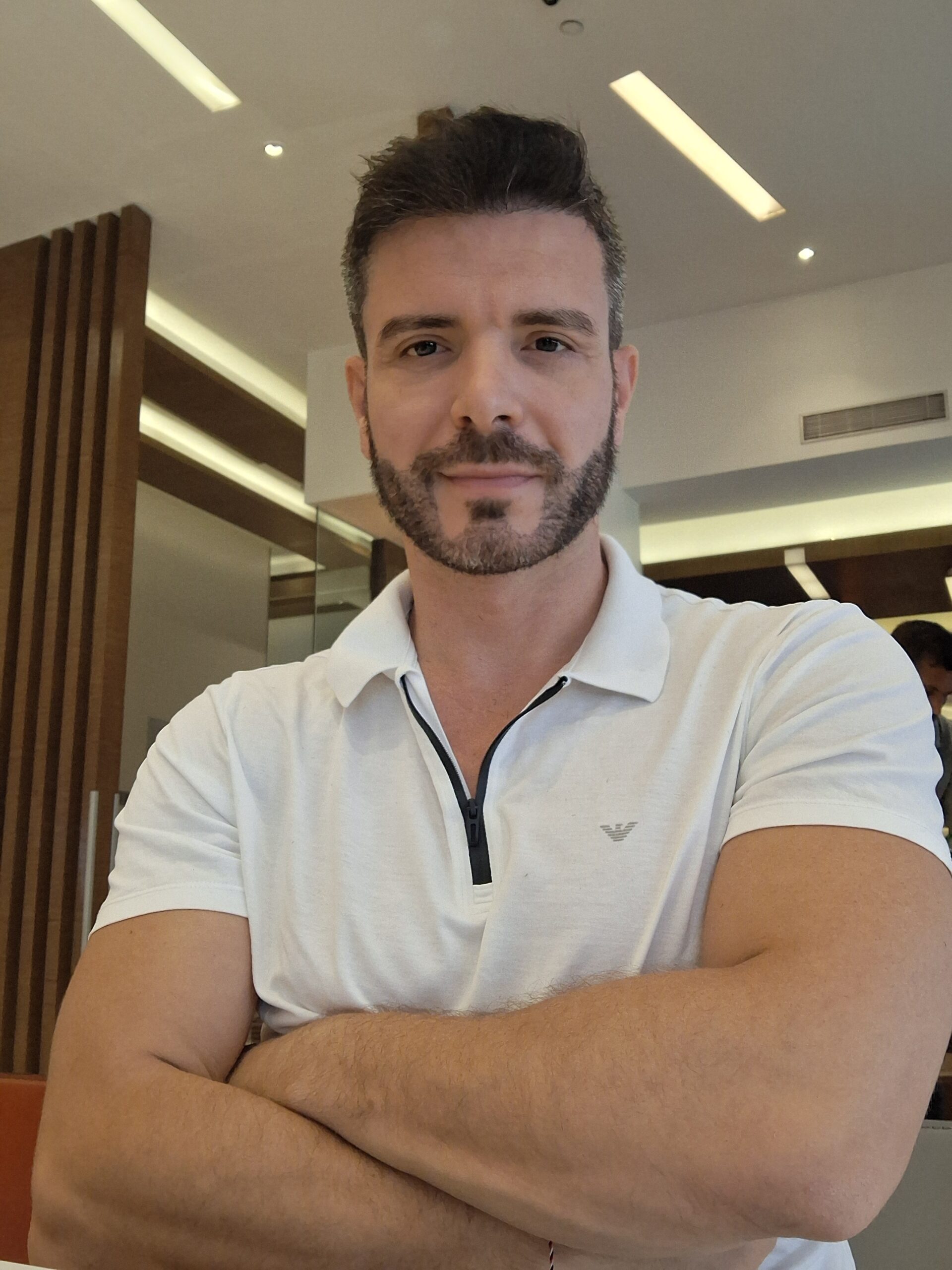
Helping businesses grow faster with AI. 🚀 At Callin.io, we make it easy for companies close more deals, engage customers more effectively, and scale their growth with smart AI voice assistants. Ready to transform your business with AI? 📅 Let’s talk!
Vincenzo Piccolo
Chief Executive Officer and Co Founder