Understanding the Financial Landscape of Healthcare AI
The financial commitment required for AI implementation in healthcare systems goes far beyond simple software purchases. Healthcare organizations considering AI adoption face a complex financial landscape that encompasses initial investment, ongoing maintenance, staff training, and technological infrastructure upgrades. According to a report by Accenture, the healthcare AI market is projected to reach $6.6 billion by 2025, highlighting the substantial financial stakes involved. This growth reflects both the enormous potential and significant costs associated with integrating AI solutions into clinical workflows. Organizations must carefully evaluate their specific needs and financial capabilities before embarking on AI transformation journeys, as discussed in our guide to conversational AI for medical offices.
Initial Investment Requirements
The upfront costs of healthcare AI implementation typically include software licensing, hardware requirements, data infrastructure, and initial consultation fees. Depending on the scope and complexity of the AI solution, these initial investments can range from $50,000 for small clinics to several million dollars for large hospital networks. A critical component often overlooked is the cost of data preparation and cleaning, which can constitute up to 60% of initial project costs. Healthcare data is notoriously fragmented and inconsistent, requiring substantial work before AI systems can effectively utilize it. Organizations must also consider the expense of creating secure environments for sensitive patient information, including AI voice assistants for FAQ handling that maintain strict HIPAA compliance.
Infrastructure Updates and Technical Requirements
Most healthcare facilities require significant infrastructure upgrades to support AI implementation. Legacy systems common in healthcare settings often lack the computing power, storage capacity, and network bandwidth necessary for AI operations. Building a suitable technical foundation may involve investments in cloud services, data warehouses, enhanced security systems, and high-performance computing resources. The Healthcare Information and Management Systems Society (HIMSS) estimates that infrastructure modernization can represent 30-40% of total AI implementation costs. These upgrades aren’t merely supporting expenses—they’re fundamental prerequisites for successful AI deployment that enable seamless integration of systems like AI calling bots for health clinics.
Staff Training and Change Management
The human component of AI implementation represents a substantial financial commitment. Healthcare organizations must invest in comprehensive training programs for clinical and administrative staff to ensure effective AI utilization. According to a study in the Journal of the American Medical Informatics Association, successful AI integration requires allocating 15-20% of the total project budget to training and change management initiatives. This includes formal education sessions, hands-on workshops, and ongoing support resources. Organizations that underinvest in this area often experience poor adoption rates and fail to realize the full potential of their AI investments. Effective change management must address not only technical skills but also cultural adaptation to new workflows enhanced by tools like AI call assistants.
Data Acquisition and Management Costs
Healthcare AI solutions thrive on data, and acquiring, managing, and maintaining this data introduces significant ongoing expenses. Organizations must budget for data storage, quality assurance, annotation, and governance throughout the AI system’s lifecycle. High-quality training data is particularly valuable and expensive, with specialized medical data sets potentially costing hundreds of thousands of dollars to develop or acquire. Additionally, healthcare providers must establish robust data governance frameworks to ensure regulatory compliance and maintain data quality over time. These ongoing data management responsibilities can represent 25-35% of annual AI operating costs, particularly for systems like conversational AI platforms that require continuous improvement through data learning.
Integration Expenses with Existing Systems
One of the most challenging and costly aspects of healthcare AI implementation involves integrating new AI solutions with existing electronic health records (EHRs) and clinical systems. These integration projects typically require specialized IT consultants, custom development work, and extensive testing. A survey by KLAS Research found that integration costs averaged $150,000-$750,000 per AI application, depending on complexity and the number of systems requiring connection. Healthcare organizations must carefully evaluate vendor claims about "seamless integration" and budget realistically for these technical challenges. Effective integration is crucial for solutions like AI appointment scheduling systems to function properly within complex healthcare IT environments.
Regulatory Compliance and Legal Considerations
Healthcare organizations must allocate substantial resources to ensure their AI implementations meet regulatory requirements and legal standards. This includes compliance with HIPAA, FDA regulations for medical devices, and various state privacy laws. The cost of regulatory consultants, legal reviews, documentation, and compliance monitoring can add 10-15% to overall implementation expenses. Additionally, organizations must consider potential liability insurance adjustments when deploying AI systems that influence clinical decisions. The evolving nature of healthcare AI regulation means that compliance requirements—and their associated costs—will continue to change, requiring ongoing attention and investment in areas like HIPAA-compliant AI phone services.
Ongoing Operational Expenses
Beyond initial implementation, healthcare organizations must budget for continuous operational costs associated with AI systems. These include software subscription fees, technical support contracts, system monitoring, regular updates, and periodic retraining of AI models. According to Gartner research, annual operational expenses typically range from 20-30% of the initial implementation cost. Organizations adopting cloud-based AI solutions may face variable expenses based on computing resources and data volume, while those with on-premises deployments must account for hardware maintenance and eventual replacement costs. Budgeting for these ongoing expenses is essential for sustainable AI adoption, particularly for systems like AI call center solutions that handle high volumes of patient interactions.
Return on Investment Timeframes
Healthcare organizations implementing AI must adopt realistic expectations about ROI timeframes. Unlike some technological investments that deliver immediate returns, AI implementations typically require 18-36 months to demonstrate significant financial benefits. This extended timeline results from the complexity of healthcare workflows, the learning curve associated with adoption, and the time needed for AI systems to accumulate sufficient data for optimization. A study in the New England Journal of Medicine Catalyst found that healthcare AI projects deliver an average ROI of 4:1 after three years of operation—impressive returns that nonetheless require patience and sustained investment. Organizations can accelerate returns by focusing initial efforts on applications with clear financial impact, such as AI voice agents for appointment scheduling that reduce administrative costs.
Hidden Costs and Common Financial Pitfalls
Many healthcare organizations encounter unexpected expenses during AI implementation. Common financial surprises include data cleaning costs exceeding initial estimates, integration challenges requiring additional consultant hours, and staff adoption issues necessitating extended training periods. Organizations frequently underbudget for essential components like thorough testing, security reviews, and performance optimization. A Deloitte Healthcare analysis found that 63% of healthcare AI projects exceeded their original budgets by 25% or more, primarily due to these hidden costs. Careful planning and comprehensive budgeting that accounts for contingencies can help avoid financial overruns that threaten project viability or delay the implementation of valuable solutions like AI cold calling systems for patient outreach.
Cost Variations by Healthcare Setting
The financial implications of AI implementation vary dramatically across different healthcare environments. Large hospital systems typically face higher absolute costs but benefit from economies of scale when deploying AI across multiple departments or facilities. Small physician practices encounter proportionally higher per-user implementation costs but may achieve faster adoption due to simpler workflows and decision-making processes. Academic medical centers often leverage research partnerships to offset certain expenses but may face higher integration costs due to complex existing systems. Rural healthcare providers typically experience higher relative costs for connectivity and infrastructure upgrades. Each setting requires customized financial planning that recognizes these unique characteristics and constraints, whether implementing AI receptionists or comprehensive clinical decision support tools.
Comparing Vendor Pricing Models
Healthcare organizations must navigate diverse AI vendor pricing structures when budgeting for implementation. Common models include perpetual licensing with maintenance fees, subscription-based Software-as-a-Service (SaaS) arrangements, usage-based pricing tied to transaction volume or data processed, and hybrid approaches combining these elements. Each model presents different financial implications for cash flow, operational budgeting, and long-term total cost of ownership. Organizations should carefully analyze these structures against their financial constraints and usage patterns. The Center for Connected Medicine recommends requesting detailed five-year cost projections from vendors to facilitate accurate comparisons, especially when evaluating specialized healthcare solutions like AI voice platforms for medical consultations.
Strategies for Cost Optimization
Healthcare organizations can employ several approaches to maximize value while controlling AI implementation costs. Phased implementation strategies allow organizations to spread investments over time while learning from early deployments. Starting with focused use cases that address specific operational pain points can demonstrate value and build momentum. Cloud-based deployments often reduce initial capital expenditures while providing scalability. Consortium approaches where multiple healthcare organizations share development and implementation costs are increasingly common, particularly among affiliated hospital systems. Open-source components can reduce certain software expenses when appropriate expertise is available. These optimization strategies help organizations balance financial constraints with the transformative potential of healthcare AI, enabling more accessible adoption of solutions like AI phone agents for customer service.
Funding Sources and Financial Support
Healthcare organizations are increasingly exploring diverse funding mechanisms for AI implementation. Beyond traditional capital budgeting, options include innovation grants from government agencies like the National Institutes of Health, partnerships with technology vendors offering favorable financing terms, and shared risk arrangements with AI companies that tie payments to achieved outcomes. Academic medical centers may leverage research funding to support dual-purpose implementations that serve both clinical and research objectives. Some healthcare systems establish innovation investment funds specifically targeting digital transformation initiatives. The Healthcare Financial Management Association documents growing availability of specialized healthcare technology financing options designed to align payment structures with realized benefits, potentially making sophisticated systems like AI call centers more financially accessible.
Measuring Success and Financial Impact
Establishing robust frameworks for measuring financial impact is essential for healthcare AI implementations. Key financial metrics typically include direct cost savings from automation, revenue improvements from enhanced scheduling efficiency, reductions in denied claims through improved documentation, and staff productivity increases. Organizations should establish clear baselines before implementation and implement systematic measurement processes throughout the project lifecycle. According to McKinsey & Company research, healthcare organizations that implement rigorous measurement frameworks are 65% more likely to achieve positive financial returns from AI investments. These measurement systems should encompass both quantitative financial metrics and qualitative indicators like patient satisfaction improvements facilitated by technology such as AI voice conversations.
Case Studies: Cost Analysis of Successful Implementations
Examining real-world implementation examples provides valuable financial insights for healthcare organizations considering AI adoption. Boston Medical Center implemented an AI-powered patient triage system with an initial investment of $2.1 million that generated annual savings of $1.4 million through reduced unnecessary emergency department visits and optimized staffing. Providence St. Joseph Health deployed an AI appointment scheduling solution at $850,000 that reduced no-show rates by 28%, resulting in approximately $3.2 million in recovered revenue annually. Banner Health’s $1.7 million investment in an AI-powered documentation assistant for physicians produced $5.3 million in annual savings through improved coding accuracy and reduced physician burnout. These case studies demonstrate both the substantial investments required and the significant financial returns possible from strategically implemented healthcare AI, similar to the benefits organizations can achieve with AI appointment booking bots.
Balancing Cost Concerns with Patient Care Benefits
Healthcare organizations must consider the broader value proposition of AI beyond direct financial returns. While cost considerations are crucial, the ultimate metric for success involves improvements in patient outcomes, safety, and experience. Investments that enhance clinical decision quality may not generate immediate revenue but can significantly reduce costs associated with preventable complications or readmissions. According to a study in Health Affairs, AI implementations that prioritize patient benefit ultimately demonstrate stronger long-term financial performance than those focusing exclusively on operational efficiency. This balanced approach requires developing evaluation frameworks that incorporate both financial metrics and patient-centered outcomes when assessing the value of investments in technologies like AI phone agents.
Future Cost Trends in Healthcare AI
Several factors will influence the financial landscape for healthcare AI in coming years. Increasing competition among AI vendors is likely to moderate software licensing costs, while advances in cloud computing may reduce infrastructure expenses. Conversely, growing demand for healthcare AI specialists will continue to drive personnel costs upward. Regulatory requirements are expected to become more defined but potentially more stringent, affecting compliance costs. The standardization of healthcare data exchange through initiatives like FHIR (Fast Healthcare Interoperability Resources) may eventually reduce integration expenses. Organizations should monitor these trends when developing long-term AI strategies and budgeting for future implementations. The American Hospital Association projects that while initial implementation costs may decrease somewhat, the sophistication and scope of healthcare AI applications will continue to expand, driving continued investment in advanced solutions like conversational AI for medical offices.
Avoiding Common Implementation Failures
Financial distress often contributes to AI implementation failures in healthcare settings. Common pitfalls include insufficient budgeting for change management, underestimating data preparation requirements, and failing to account for integration complexity. Organizations frequently struggle when they attempt to implement too many AI applications simultaneously rather than pursuing focused, strategic deployments. According to Harvard Business Review research, nearly 70% of healthcare AI implementations encounter significant financial or operational challenges. Organizations can mitigate these risks through comprehensive planning, realistic budgeting with appropriate contingencies, phased implementation approaches, and careful vendor selection. Successful implementations often start with clearly defined problems and measurable objectives rather than broad technological aspirations, an approach recommended for all AI implementations from clinical decision support to AI phone services.
Small-Scale Starting Points for Budget-Conscious Organizations
Healthcare providers with limited initial budgets can still benefit from AI through strategic, focused implementations. Targeted applications like automated appointment reminders, simple triage chatbots, or focused revenue cycle management tools typically require investments of $25,000-$100,000 while delivering measurable returns. These entry-level implementations allow organizations to develop AI expertise, demonstrate value, and build internal support for broader initiatives. Cloud-based solutions with subscription pricing models can further reduce initial capital requirements. Many vendors now offer starter packages specifically designed for smaller organizations or limited deployments. These modest first steps can serve as valuable learning experiences and proof points for organizations beginning their AI journeys, particularly when utilizing scalable platforms like those offered by Callin.io for healthcare communication needs.
Transform Your Healthcare Communications with AI Solutions
If you’re looking to enhance patient communication while controlling costs, exploring Callin.io’s healthcare-focused solutions could be your next strategic step. Their platform allows healthcare providers to implement AI phone agents that can handle appointment scheduling, answer common patient questions, and manage routine administrative calls with natural, human-like interactions. These solutions address many of the implementation challenges discussed throughout this article by offering scalable, subscription-based options that minimize upfront investment.
Callin.io’s free account provides access to an intuitive interface for configuring your AI agent, with test calls included and a comprehensive task dashboard for monitoring interactions. For healthcare organizations seeking advanced capabilities like Google Calendar integration and CRM connectivity, subscription plans starting at just $30 per month offer exceptional value. By reducing administrative burdens while improving patient communication, these AI solutions deliver tangible benefits that help offset implementation costs. Learn more about how Callin.io can support your healthcare organization’s unique communication needs at Callin.io.
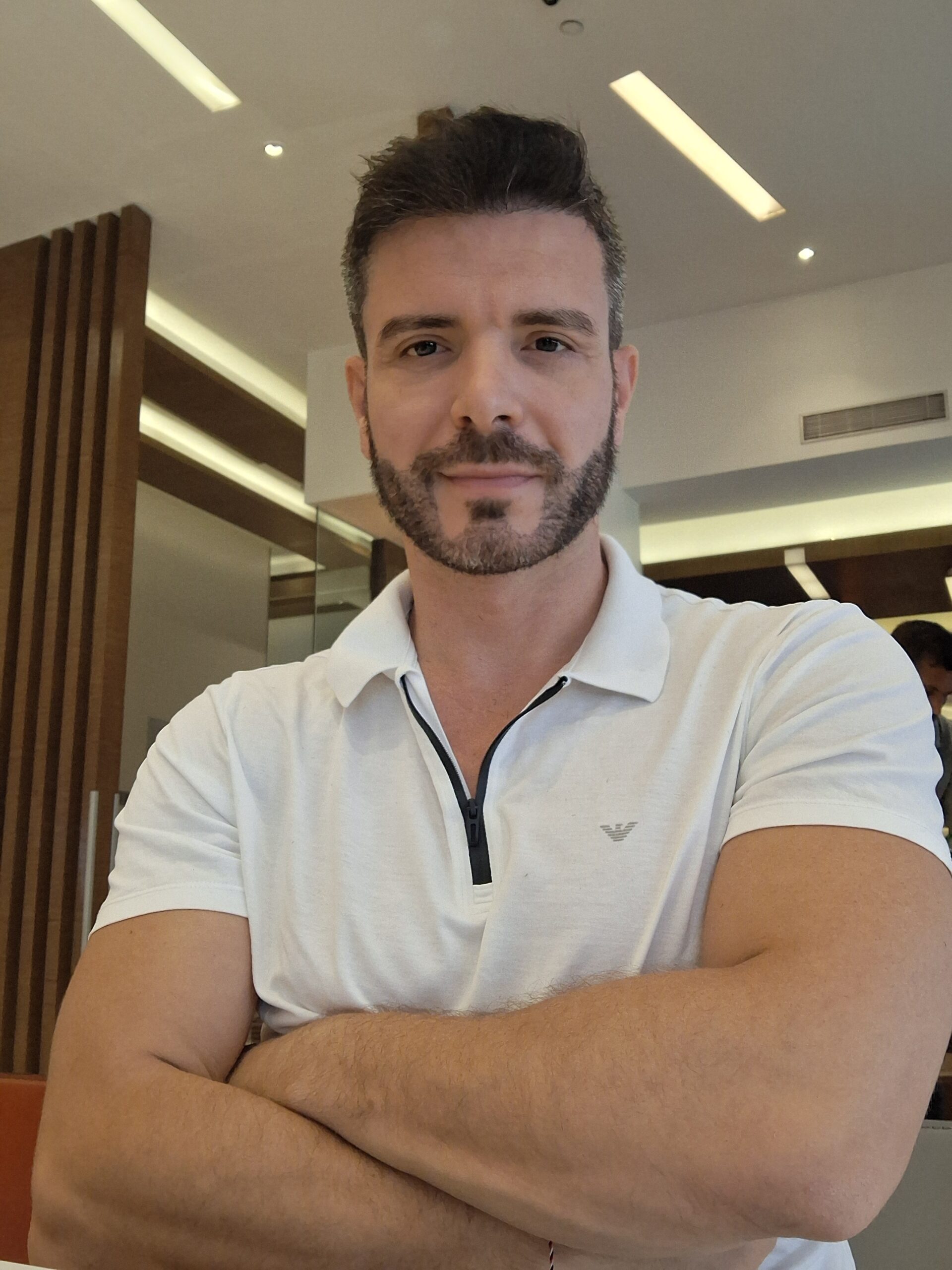
Helping businesses grow faster with AI. 🚀 At Callin.io, we make it easy for companies close more deals, engage customers more effectively, and scale their growth with smart AI voice assistants. Ready to transform your business with AI? 📅 Let’s talk!
Vincenzo Piccolo
Chief Executive Officer and Co Founder