Defining the Fundamentals of AI Communication
At first glance, conversational agents and chatbots might seem like interchangeable terms, but they represent distinctly different approaches to automated communication. Conversational agents are sophisticated AI systems designed to engage in natural, human-like dialogue across multiple topics and contexts. Unlike their simpler counterparts, these advanced tools can maintain conversation continuity, remember previous interactions, and adapt their responses based on evolving dialogue patterns. According to research from the MIT Technology Review, conversational agents typically employ complex neural networks and natural language understanding frameworks that enable them to process context, emotion, and intent. On the other hand, chatbots generally operate within predetermined response patterns, following scripted pathways that limit their conversational flexibility. This fundamental distinction shapes how businesses implement these technologies for customer engagement, a subject explored in depth in our guide to AI voice conversations.
The Technical Architecture Behind Each Solution
The underlying architecture of conversational agents and chatbots reveals substantial differences in their capabilities. Conversational agents rely on sophisticated large language models (LLMs) combined with natural language understanding (NLU) and natural language generation (NLG) components to process and produce human-like text. These agents typically incorporate multiple AI technologies, including sentiment analysis, entity recognition, and contextual memory systems. By contrast, chatbots often utilize simpler rule-based systems or basic machine learning algorithms focused on pattern matching and keyword recognition. The comparative complexity becomes evident when examining use cases like AI for call centers, where conversational agents can handle nuanced customer inquiries with greater flexibility than traditional chatbots. The technical sophistication of conversational agents allows them to operate across various communication channels while maintaining conversation coherence and contextual relevance.
Conversational Depth and Context Management
One of the most striking differences between these AI communication tools lies in their conversational depth and context handling abilities. Conversational agents excel at managing complex, multi-turn dialogues while maintaining contextual awareness throughout extended interactions. These advanced systems can reference earlier points in the conversation, ask clarifying questions when needed, and build comprehensive user context profiles over time. This capability proves especially valuable in scenarios requiring detailed information exchange, such as AI phone consultations for businesses. Chatbots typically struggle with maintaining context beyond a few exchanges and often fail to connect related information across different parts of a conversation. The Stanford Human-Centered AI Institute has documented how conversational agents demonstrate up to 78% better context retention compared to standard chatbots, particularly in customer support scenarios handling complex product inquiries or multi-step troubleshooting processes.
Learning and Adaptation Capabilities
The learning mechanisms embedded within these AI communication tools represent another significant point of divergence. Conversational agents incorporate sophisticated machine learning techniques that enable them to improve through continuous interaction. These systems analyze conversation patterns, user feedback, and outcome data to refine their response strategies and conversational abilities over time. Many advanced conversational agents, like those used in AI calling agencies, employ reinforcement learning from human feedback (RLHF) techniques to align their responses more closely with human preferences and expectations. Traditional chatbots, however, typically require manual updates to their response libraries and decision trees. Without explicit reprogramming, most chatbots remain static in their conversational capabilities, limited to the patterns and responses initially configured by their developers.
User Experience and Conversation Flow
The user experience delivered by these technologies differs dramatically in terms of conversation flow and natural interaction. Conversational agents create more fluid dialogue experiences, with smoother transitions between topics and fewer conversational dead-ends. These systems can handle unexpected user inputs, topic changes, and ambiguous questions while maintaining coherent conversation flow. This capability proves particularly valuable in phone-based interactions, as detailed in our guide to AI phone services. Users engaging with conversational agents report higher satisfaction rates, with studies by Gartner Research indicating a 35% increase in positive experience ratings compared to traditional chatbot interactions. Chatbots typically offer more structured and predictable conversational paths, which can feel rigid and mechanical to users. When faced with queries outside their programmed parameters, chatbots often default to fallback responses that disrupt the natural flow of communication.
Implementation Complexity and Resource Requirements
The implementation pathway for each solution reflects significant differences in complexity and organizational commitment. Deploying conversational agents typically requires substantial investment in AI infrastructure, training data, and specialized expertise. Organizations must consider factors such as model selection, training methodologies, and integration with existing systems when implementing these advanced solutions, as outlined in our guide to creating your own LLM. Conversational agents also demand ongoing maintenance and optimization to ensure continued performance improvement. Chatbots, conversely, offer a more accessible entry point for organizations with limited AI expertise or budget constraints. Many platforms provide no-code or low-code chatbot development environments that simplify deployment without requiring deep technical knowledge of AI systems. This implementation difference creates distinct adoption pathways based on organizational resources, technical capabilities, and strategic priorities.
Use Cases: When to Choose Each Solution
Different business scenarios call for different AI communication approaches based on complexity, interaction depth, and customer expectations. Conversational agents shine in scenarios requiring nuanced human-like interaction, particularly in high-value customer touchpoints such as AI sales calls and complex customer service scenarios. They excel in use cases involving detailed product explanations, personalized recommendations, or multi-step processes like appointment scheduling. Our article on AI appointment schedulers demonstrates this capability in action. Chatbots typically perform best in straightforward, transaction-focused interactions with clear user intents and limited variation in request types. They’re well-suited for basic information retrieval, simple FAQs, and structured processes like order tracking or basic form completions. The choice between these tools should align with the complexity of the customer journey and the value of the interaction to your business outcomes.
Voice-Based Applications and Capabilities
The implementation of these AI technologies in voice-based applications reveals further distinctions in their capabilities and performance. Conversational agents have made remarkable strides in voice interaction, incorporating advanced text-to-speech technologies with natural-sounding prosody and emotional intonation. These systems can process complex audio inputs, understand different accents and speaking styles, and generate remarkably human-like speech outputs. For businesses interested in implementing voice-based AI, our guide to text-to-speech technology provides valuable insights. Leading voice synthesis platforms like ElevenLabs have partnered with conversational agent developers to create truly lifelike voice interactions. Traditional chatbots often struggle with voice applications, lacking the sophisticated speech recognition and natural language understanding required for fluid voice conversation, resulting in disjointed interactions that frustrate users.
Personalization and Customer Journey Integration
The ability to personalize interactions and integrate with broader customer journeys differentiates these AI communication tools in meaningful ways. Conversational agents can develop rich customer profiles based on interaction history, preferences, and behavioral patterns. These systems leverage this information to tailor conversations to individual users, reference previous interactions, and provide recommendations aligned with specific customer needs. When integrated with CRM systems and other business tools, conversational agents can deliver highly personalized experiences across the entire customer journey, as demonstrated in our article on AI voice assistants for FAQ handling. Chatbots typically offer more limited personalization capabilities, often restricted to basic information like customer names or account details. Their integration with broader customer journey touchpoints tends to be more compartmentalized, limiting their ability to provide truly cohesive cross-channel experiences.
Scalability and Performance Under Load
How these technologies perform under increasing workloads reveals important operational differences for organizations considering implementation. Conversational agents typically leverage cloud-based infrastructure designed for elastic scaling, allowing them to handle fluctuating conversation volumes without degradation in response quality or speed. Advanced systems can dynamically allocate computational resources based on conversation complexity, ensuring consistent performance even during peak demand periods. This scalability makes them particularly valuable for businesses with variable customer interaction patterns or seasonal demand spikes, as explored in our guide to AI call center solutions. Chatbots generally offer good technical scalability for handling large volumes of standardized interactions, but their effectiveness often diminishes when facing varied or complex queries at scale, resulting in higher escalation rates to human agents during high-volume periods.
Integration with Business Systems and Workflows
The integration capabilities of these AI communication tools significantly impact their value to overall business operations. Conversational agents typically offer robust API ecosystems and integration frameworks that enable seamless connection with customer relationship management systems, enterprise resource planning platforms, knowledge bases, and other business-critical applications. These integrations allow conversational agents to access real-time data, execute transactions, and update systems based on customer interactions. For organizations seeking to implement such capabilities, our article on white label AI voice agents provides valuable implementation insights. Chatbots can also integrate with business systems, but typically with more limited data exchange capabilities and fewer options for custom integration development, potentially creating information silos that reduce their overall utility within complex business environments.
Measurement and Analytics Capabilities
The analytics capabilities built into these AI communication tools provide different levels of insight into customer interactions and system performance. Conversational agents typically offer comprehensive analytics suites that track not just basic metrics like completion rates and conversation duration, but also nuanced interaction patterns, sentiment trends, topic emergence, and conversation quality indicators. These advanced analytics enable organizations to continuously refine their AI communication strategy based on detailed performance data. This approach to measurement aligns with best practices detailed in our guide to using AI for sales. Chatbot analytics tend to focus on more fundamental operational metrics, such as usage volumes, abandonment rates, and escalation points. While useful for basic performance monitoring, these metrics often lack the depth needed for sophisticated conversation optimization and user experience enhancement.
Cost Structure and Return on Investment
The financial considerations surrounding these AI communication tools reflect their differing capabilities and implementation requirements. Conversational agents typically involve higher initial investment for development, training, and deployment, with costs potentially ranging from tens to hundreds of thousands of dollars for enterprise-grade solutions. However, their superior handling of complex interactions can deliver substantial ROI through reduced call center costs, improved conversion rates, and enhanced customer lifetime value. For organizations seeking cost-effective implementation approaches, our guide to Twilio cheaper alternatives offers valuable insights. Chatbots generally require lower upfront investment, making them accessible to organizations with limited AI budgets. While they provide positive ROI for simple, high-volume interactions, their limited capabilities often mean diminishing returns when applied to complex customer engagement scenarios that require sophisticated conversation management.
Security and Compliance Considerations
Security and regulatory compliance requirements present distinct challenges for different AI communication implementations. Conversational agents typically incorporate advanced security frameworks including data encryption, access controls, and privacy by design principles to protect sensitive customer information exchanged during interactions. These systems can be configured to maintain compliance with industry regulations such as GDPR, HIPAA, and PCI DSS through features like automated data anonymization, retention policies, and consent management. For regulated industries like healthcare, our article on AI calling bots for health clinics addresses these specific compliance considerations. While chatbots also implement security measures, their typically more limited architecture may offer fewer options for customized security configurations and compliance controls, potentially creating challenges for organizations in highly regulated industries or those handling particularly sensitive customer data.
The Role of Human Oversight and Intervention
The relationship between these AI technologies and human agents represents another key differentiator in implementation strategy. Conversational agents increasingly employ sophisticated human-in-the-loop frameworks that enable seamless transitions between AI and human agents when conversations exceed the system’s capabilities. These hybrid approaches allow organizations to maximize automation benefits while ensuring complex issues receive appropriate human attention. Advanced systems can provide human agents with conversation context, sentiment analysis, and suggested responses when transfers occur, as detailed in our guide to AI call assistants. Traditional chatbots typically utilize simpler escalation protocols, often lacking the contextual handoff capabilities that preserve conversation continuity during transfers to human agents. This limitation can create frustrating customer experiences where users must repeat information or restart their inquiry process when chatbot interactions are elevated to human support.
Customization and Brand Alignment
The extent to which these AI communication tools can be customized to reflect unique brand identities and communication styles varies significantly. Conversational agents offer extensive customization options including personality design, vocabulary selection, response tone calibration, and conversation flow architecture. These capabilities enable organizations to create distinctive AI personalities that authentically represent their brand voice and values across customer interactions. For businesses interested in maintaining this level of brand control, our article on white label AI bots explores implementation options. Chatbots typically provide more limited personality customization, often restricted to visual elements and basic response phrasing rather than fundamental conversational style or complex brand-aligned communication strategies. This limitation can create disconnects between chatbot interactions and broader brand experiences, potentially undermining brand consistency across customer touchpoints.
Multi-Channel and Omni-Channel Capabilities
The ability to maintain consistent conversation experiences across different communication channels represents a growing priority for organizations implementing AI communication strategies. Conversational agents increasingly offer true omnichannel capabilities, maintaining continuous conversation context as users move between channels like websites, mobile apps, social media platforms, and phone interactions. This seamless experience preservation aligns with contemporary customer expectations for frictionless cross-channel journeys, as explored in our guide to omnichannel communication strategies. Traditional chatbots more commonly implement multi-channel rather than true omnichannel approaches, with separate instances operating across different platforms without shared context or conversation history. This siloed approach can create disjointed customer experiences when interactions span multiple channels or devices, potentially requiring customers to restart conversations when changing communication methods.
Current Limitations and Challenges
Despite their significant capabilities, both AI communication approaches face distinct challenges that organizations should consider during implementation planning. Conversational agents continue to struggle with certain complex conversation scenarios including deeply contextual cultural references, highly specialized domain knowledge, and nuanced emotional responses in sensitive situations. They may also face computational challenges when handling unusually long conversation histories or extremely complex multi-topic dialogues. Our article on prompt engineering for AI callers addresses strategies for mitigating these limitations. Traditional chatbots encounter more fundamental constraints including limited natural language understanding, poor handling of conversation deviations, and inability to resolve ambiguity through clarification questions. Both technologies continue to evolve as researchers and developers address these challenges through improved algorithms, larger training datasets, and enhanced architectural approaches.
Future Trajectory and Emerging Capabilities
The development roadmap for these AI communication technologies points toward increasing convergence and capability expansion in coming years. Conversational agents are rapidly incorporating multimodal capabilities that extend beyond text and voice to include visual understanding, sentiment analysis through facial expression recognition, and gesture interpretation. These expanded capabilities promise even more natural and context-rich human-AI interactions across physical and digital environments. For businesses interested in staying ahead of these trends, our article on AI voice assistants explores emerging capabilities. Chatbot evolution is increasingly focused on incorporating more conversational agent characteristics, with simplified versions of contextual memory, intent recognition, and adaptive conversation flows becoming standard features in next-generation chatbot platforms. This convergent evolution suggests a future where the distinction between these technologies becomes increasingly blurred as capabilities advance across both categories.
Making the Right Choice for Your Business
Selecting the appropriate AI communication technology requires careful assessment of your specific business requirements, customer expectations, and operational capabilities. Organizations should consider factors including conversation complexity, integration requirements, personalization needs, and budget constraints when evaluating these technologies. For high-value, complex customer interactions in industries like healthcare, finance, or premium retail, conversational agents typically deliver superior experiences and business outcomes despite higher implementation costs. For straightforward, transactional interactions with clear user intents, well-designed chatbots may provide sufficient capabilities with lower implementation complexity. Many organizations implement hybrid approaches, deploying conversational agents for complex, high-value interactions while using chatbots for simpler, high-volume scenarios. Our comprehensive guide to AI phone agents provides detailed implementation considerations for organizations navigating these decisions.
Real-World Success Stories and Implementation Examples
Examining real-world implementations illustrates the practical impact of these technology choices across different business contexts. Leading healthcare providers have deployed conversational agents to handle patient scheduling, medication queries, and preliminary symptom assessment, resulting in 40% reductions in administrative call volumes and 25% improvements in appointment adherence. Financial institutions using conversational agents for investment advisory services report 30% increases in client engagement and 15% growth in portfolio expansion among digital customers. These results align with case studies featured in our article on AI for medical offices. Retail organizations implementing well-designed chatbots for order tracking and basic product information have achieved 20% reductions in routine customer service inquiries while maintaining satisfaction ratings. These real-world examples demonstrate how different AI communication approaches can deliver meaningful business results when appropriately matched to specific use cases and customer needs.
Transform Your Business Communication with Callin.io’s Advanced AI Solutions
If you’re ready to elevate your business communication strategy beyond basic automation, Callin.io offers state-of-the-art conversational AI solutions designed for meaningful customer engagement. Our platform enables you to deploy sophisticated AI phone agents that can handle inbound and outbound calls with remarkable natural conversation capabilities. These AI agents can autonomously schedule appointments, answer complex customer questions, and even manage sales conversations with human-like understanding and responsiveness.
With Callin.io’s free account, you can quickly set up your customized AI agent through our intuitive interface, including test calls to experience the technology firsthand and access to our comprehensive task dashboard for monitoring interactions. For businesses requiring advanced functionality, our subscription plans starting at just $30 per month include seamless Google Calendar integration, built-in CRM capabilities, and enterprise-grade conversation analytics. Don’t let your business fall behind in the AI communication revolution—discover how Callin.io can transform your customer engagement experience today at Callin.io.
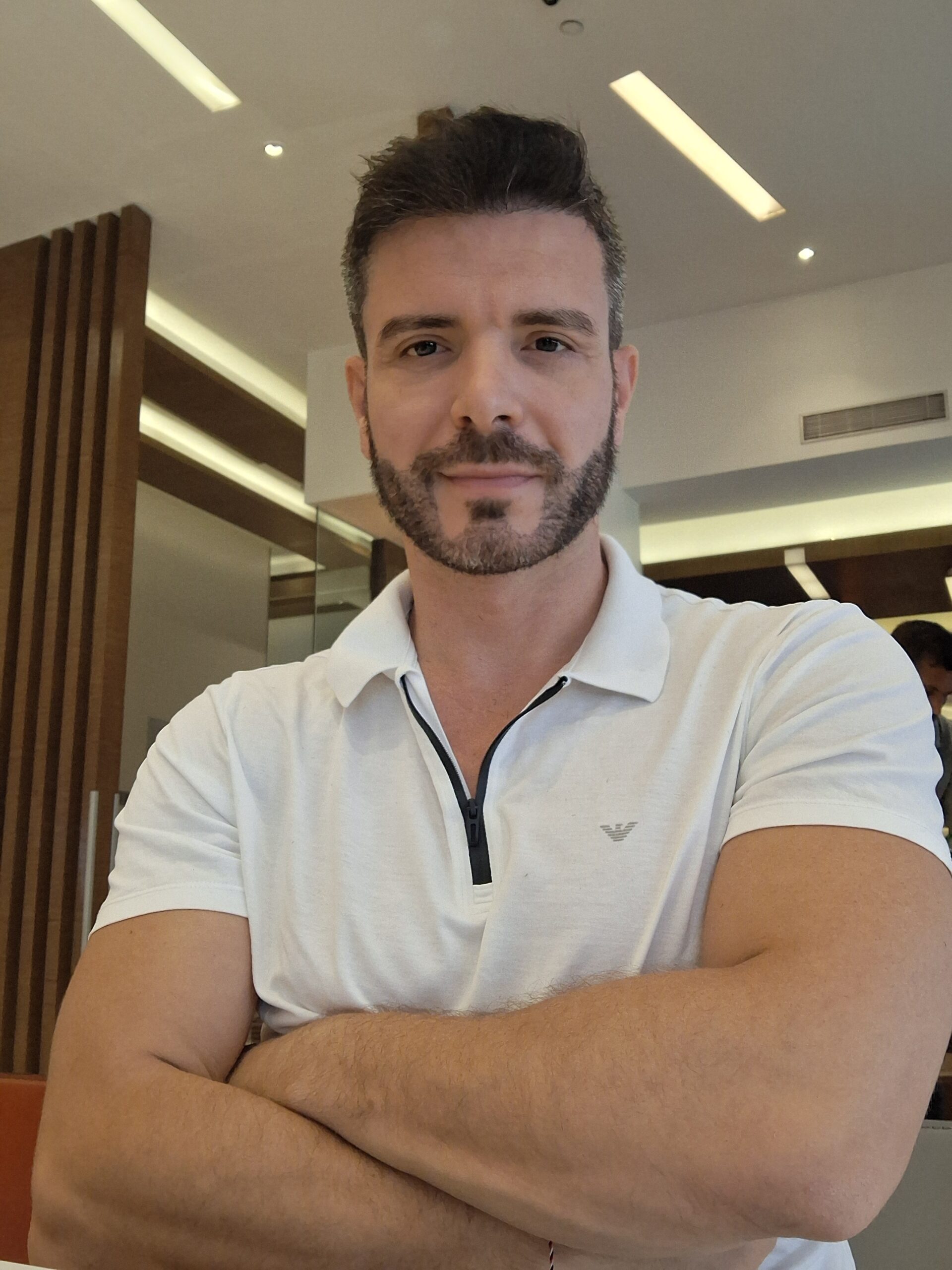
Helping businesses grow faster with AI. 🚀 At Callin.io, we make it easy for companies close more deals, engage customers more effectively, and scale their growth with smart AI voice assistants. Ready to transform your business with AI? 📅 Let’s talk!
Vincenzo Piccolo
Chief Executive Officer and Co Founder