Understanding the White Label AI SaaS Revolution
White label AI SaaS represents a transformative business opportunity in today’s digital landscape, allowing companies to offer sophisticated artificial intelligence solutions under their own branding. This approach enables businesses to leverage pre-built AI infrastructure without the substantial investment in development resources that building proprietary solutions would require. As the AI market continues its exponential growth—projected to reach $407 billion by 2027 according to Grand View Research—white label solutions have become increasingly attractive for entrepreneurs and established businesses alike. The strategic advantage lies in the ability to rapidly deploy market-ready AI products while maintaining brand consistency and focusing on customer relationships rather than technical development. Companies like Callin.io have pioneered this space by offering white label AI voice agents that transform how businesses handle customer communications.
The Strategic Advantages of White Label AI Solutions
Implementing white label AI SaaS offers multiple competitive advantages for businesses looking to expand their service portfolio. Cost efficiency stands as perhaps the most compelling benefit, as organizations can circumvent the substantial expenses associated with in-house AI development—including specialized talent acquisition, infrastructure investment, and ongoing maintenance. Time-to-market acceleration represents another critical advantage, allowing companies to introduce sophisticated AI capabilities in weeks rather than the months or years required for custom development. This rapid deployment capability enables businesses to respond swiftly to market demands and capitalize on emerging opportunities before competitors. Additionally, scalability becomes significantly more manageable with white label solutions, as providers like Callin.io handle the technical infrastructure required to support growing usage volumes. For businesses considering this approach, exploring AI calling business opportunities can provide valuable insights into implementation strategies.
Key Components of Effective White Label AI Platforms
A robust white label AI SaaS solution encompasses several essential components that determine its effectiveness for resellers and end-users alike. At its foundation, the platform must feature comprehensive customization capabilities that extend beyond simple logo placement to include interface adjustments, workflow configurations, and output formatting that aligns with the reseller’s brand identity. Equally important is an intuitive administrative dashboard that empowers resellers to manage user accounts, monitor system performance, and access detailed analytics without technical assistance. The most successful white label platforms also integrate seamless API connectivity to facilitate integration with existing business systems like CRM software, communication tools, and data repositories. For customer-facing applications, conversational AI capabilities have become particularly valuable, enabling natural language interactions that enhance user experience. Looking at industry examples such as Twilio’s AI assistants provides insight into how these components work together in practice.
Selecting the Right White Label AI Partner
Choosing an appropriate white label AI provider represents a critical business decision that requires thorough evaluation across multiple dimensions. Technical reliability should be prioritized, including assessment of the provider’s infrastructure redundancy, uptime guarantees, and disaster recovery protocols. Beyond basic functionality, evaluate the sophistication of the AI technology itself—examining factors like language understanding capabilities, contextual awareness, and learning algorithms that improve performance over time. The provider’s roadmap for future development deserves careful consideration, ensuring their innovation trajectory aligns with your business objectives and market demands. Additionally, assess customization flexibility, ensuring the solution can be thoroughly branded and tailored to your specific requirements. Companies like Callin.io offer white label AI receptionists that exemplify the balance between sophistication and customizability. For comparative analysis, resources like Vapi AI white label alternatives can help businesses understand the competitive landscape.
Revenue Models for White Label AI Businesses
Establishing a profitable white label AI SaaS business requires careful consideration of revenue structure and pricing strategies. The subscription-based model has emerged as the predominant approach, offering predictable recurring revenue while providing clients with manageable operational expenses rather than substantial capital investments. These subscriptions typically feature tiered pricing based on usage metrics such as API calls, processing volume, or user seats. Value-based pricing represents another effective strategy, where fees correlate directly with measurable business outcomes like cost savings, revenue generation, or productivity improvements. Some white label providers also implement hybrid models incorporating setup fees for initial customization and implementation, followed by ongoing subscription payments. For businesses exploring this space, examining AI reseller opportunities can provide valuable insights into prevailing revenue models and market expectations. The AI calling agency market demonstrates how these models are being successfully deployed in practice.
Industry Applications: Transforming Customer Service
Customer service represents one of the most impactful applications for white label AI solutions, with substantial ROI potential through automation of routine inquiries and enhancement of agent capabilities. AI-powered virtual receptionists can manage incoming calls 24/7, qualifying leads, answering common questions, and routing complex issues to appropriate human representatives without the limitations of traditional IVR systems. Similarly, AI chatbots integrated with knowledge bases can resolve customer inquiries across digital channels with remarkable speed and consistency. The implementation of AI call assistants has demonstrated particular effectiveness in reducing average handling time while improving first-contact resolution rates. Organizations leveraging these technologies report significant operational benefits, including 40-70% reduction in call center costs according to Deloitte research. For businesses interested in this application, exploring call center voice AI options provides valuable implementation guidance.
Revolutionizing Sales and Lead Generation
White label AI sales solutions have emerged as powerful tools for businesses seeking to enhance revenue generation through automation and intelligence-driven approaches. AI calling agents can systematically conduct outreach campaigns at unprecedented scale, engaging potential customers through natural conversations while qualifying leads based on predetermined criteria. These systems excel at handling routine aspects of the sales process—such as appointment scheduling, product information delivery, and initial qualification—freeing human representatives to focus on relationship-building and complex negotiations. The implementation of AI cold callers has proven particularly effective for businesses with high-volume prospecting requirements. Advanced platforms also incorporate adaptive learning capabilities, continuously refining sales approaches based on interaction outcomes and success patterns. For organizations considering implementation, resources like AI sales white label solutions and AI appointment setters provide valuable insights into capabilities and deployment strategies.
Implementing White Label AI for Healthcare
The healthcare sector presents distinctive opportunities for white label AI applications, particularly in patient engagement, administrative efficiency, and preliminary assessment. AI-powered scheduling systems have demonstrated remarkable effectiveness in reducing appointment no-shows by 25-30% through intelligent reminders, confirmation automation, and easy rescheduling options. Similarly, AI voice conversation systems can conduct pre-appointment screening, gather medical history, and document symptoms before physician consultations, improving clinical efficiency and documentation quality. For administrative processes, AI solutions automate insurance verification, benefit explanation, and payment processing, substantially reducing staff workload while improving accuracy. Healthcare providers implementing these systems report significant operational improvements, including reduced wait times and enhanced patient satisfaction. For healthcare organizations exploring implementation, specialized solutions like AI calling bots for health clinics and conversational AI for medical offices offer industry-specific functionality designed to address healthcare’s unique requirements.
White Label AI for Real Estate Operations
Real estate businesses have discovered substantial efficiency gains through implementation of white label AI technologies across multiple operational areas. AI property-matching systems analyze client preferences against available inventory, intelligently prioritizing showings based on alignment with buyer requirements and behavior patterns. For property management, AI-powered virtual assistants handle tenant communications, maintenance requests, and payment reminders, delivering 24/7 responsiveness without corresponding staffing costs. The deployment of AI calling agents for real estate has proven particularly valuable for lead qualification and appointment scheduling, enabling agents to focus exclusively on high-potential interactions. These systems also excel at transaction coordination, automating document collection, deadline tracking, and closing preparation tasks that traditionally consume significant administrative time. For agencies considering implementation, white label solutions provide rapid deployment capability without the technology development burden, allowing focus on distinctive service delivery rather than software development. Resources like AI appointment booking bots offer insights into implementation approaches.
Customization Best Practices for White Label AI
Effective customization of white label AI solutions requires strategic approach to ensure the technology seamlessly represents your brand while delivering optimal performance. Brand alignment represents the first critical consideration, encompassing visual elements like color schemes, typography, and logo integration, alongside voice and communication style that reflects your organization’s personality and values. Domain-specific knowledge incorporation substantially enhances performance, requiring investment in training the AI system with industry terminology, common scenarios, and specialized information relevant to your business context. Workflow integration demands careful mapping of the AI system to existing business processes, ensuring seamless handoffs between automated and human-managed tasks. For voice-based applications, prompt engineering for AI callers plays a critical role in shaping natural, effective conversations. Organizations should establish clear success metrics before implementation, enabling objective assessment of the customized solution’s impact against business objectives like cost reduction, customer satisfaction improvement, or revenue enhancement.
Technical Integration Challenges and Solutions
Integrating white label AI solutions into existing technology ecosystems presents technical challenges that require systematic resolution approaches. Data integration complexities frequently emerge as obstacles, particularly when connecting AI systems with legacy databases or applications using proprietary formats. Implementing standardized API connections and establishing middleware layers can address these challenges by creating structured data pathways between systems. Authentication and security synchronization represents another critical concern, requiring careful implementation of unified access control and data protection measures across the integrated environment. For smaller businesses without extensive IT resources, white label providers offering comprehensive AI phone services with turnkey integration capabilities present attractive options. Organizations should also consider connectivity requirements, particularly for voice-based AI applications that depend on reliable telephony infrastructure. Resources like SIP trunking provider guides can help address these connectivity considerations. The most successful implementations typically involve phased approaches with targeted pilot programs before full-scale deployment.
Compliance and Ethical Considerations
Implementing white label AI technology demands rigorous attention to regulatory compliance and ethical implications across multiple dimensions. Privacy regulations such as GDPR, CCPA, and industry-specific requirements like HIPAA establish strict guidelines for data handling, requiring transparent collection practices, secure storage protocols, and user control mechanisms. Disclosure obligations represent another critical consideration, as many jurisdictions now mandate explicit notification when customers interact with AI systems rather than human representatives. Organizations must also address bias mitigation through regular auditing of AI outputs for potential discriminatory patterns and implementation of corrective measures when detected. For voice applications, recording consent requirements vary significantly by jurisdiction, requiring location-aware compliance protocols. White label providers offering AI phone numbers should provide comprehensive compliance documentation and configurable disclosure mechanisms. Organizations implementing these technologies should consider establishing ethical AI usage committees to evaluate implementation approaches against both regulatory requirements and organizational values.
Performance Monitoring and Optimization
Continuous improvement of white label AI implementations requires systematic performance monitoring across technical, operational, and business dimensions. Technical metrics should track system reliability indicators like uptime percentages, response latency, and error rates, establishing baselines and improvement targets for each. Interaction effectiveness measures—including task completion rates, escalation frequencies, and sentiment analysis—provide insight into how successfully the AI system handles its assigned functions. User satisfaction data gathered through post-interaction surveys and feedback mechanisms offers valuable qualitative insight complementing quantitative metrics. For voice-based systems, specialized analytics examining conversation flow, interruption handling, and natural language understanding accuracy help identify specific improvement opportunities. Organizations implementing AI call center solutions should establish formal review cadences examining performance metrics against business objectives, guiding iterative refinement of the system. Providers like Callin.io typically offer integrated analytics dashboards facilitating this ongoing optimization process.
Scaling Your White Label AI Business
Expanding a white label AI business requires strategic approaches to infrastructure, market development, and operational efficiency. Technical scalability represents the foundation, demanding cloud-based architecture with elastic resource allocation, distributed processing capabilities, and automated load balancing to accommodate growing transaction volumes without performance degradation. Market expansion typically follows either vertical specialization—developing deep expertise and tailored solutions for specific industries like healthcare or finance—or horizontal diversification across multiple sectors with adaptable core technology. For businesses pursuing reseller relationships, establishing structured AI reseller programs with comprehensive onboarding, technical support, and marketing resources accelerates channel partner success. Operational scalability requires implementing standardized deployment methodologies, self-service configuration tools, and knowledge base resources that reduce implementation complexity and support requirements. Organizations pursuing expansion should also develop clear differentiation strategies highlighting unique capabilities, specialized integrations, or domain expertise that distinguishes their offering in increasingly competitive markets.
Case Study: Transforming Customer Support with White Label Voice AI
Financial services provider MidwestBank illustrates the transformative impact of white label AI implementation in customer support operations. Facing escalating call volumes and customer frustration with extended wait times, the institution implemented a white labeled AI voice agent handling account balance inquiries, transaction verification, and basic service requests. The system—branded as "MidwestBank Assistant" to maintain consistent customer experience—processed over 60% of incoming inquiries without human intervention within three months of deployment. Performance metrics demonstrated 24/7 availability with average response times under two seconds, compared to previous 8-minute average wait times during peak periods. Customer satisfaction surveys showed 87% positive ratings for AI interactions, with particularly favorable responses to consistent information delivery and elimination of hold times. The implementation generated 42% cost reduction in support operations while enabling reassignment of service representatives to complex customer needs requiring human judgment and relationship building. This case exemplifies how white label AI technology can deliver simultaneous improvements in operational efficiency, service accessibility, and customer experience when thoughtfully implemented.
Emerging Trends in White Label AI Technology
The white label AI landscape continues evolving rapidly, with several transformative trends reshaping solution capabilities and implementation approaches. Multimodal AI integration represents perhaps the most significant development, combining voice, text, image, and video processing capabilities within unified platforms that can engage across multiple communication channels simultaneously. Emotion recognition technologies are increasingly incorporated into conversational systems, enabling detection of customer sentiment through voice tone analysis and adaptive responses based on emotional context. For voice-based applications, advances in text-to-speech technology have dramatically improved natural delivery, with state-of-the-art systems from providers like ElevenLabs approaching human-indistinguishable quality. Federated learning approaches are addressing privacy concerns by enabling AI improvement without centralized data collection, training models across distributed datasets while maintaining information security. Organizations evaluating white label partners should assess their innovation roadmaps against these emerging capabilities to ensure selected solutions remain competitive as technology evolves.
Comparing White Label Providers: Key Differentiators
Selecting the optimal white label AI provider requires systematic evaluation across multiple capability dimensions and business considerations. Technology sophistication varies significantly between providers, with leading platforms offering advanced natural language understanding, contextual awareness, and learning capabilities that continuously improve performance through interaction analysis. Customization depth represents another critical differentiator, ranging from simple visual branding to comprehensive functional adjustment capabilities that enable precise alignment with business requirements. Integration capabilities also vary substantially, with some providers offering extensive pre-built connectors to popular business systems while others require custom development for connections. Support models differ significantly as well, from self-service documentation to dedicated implementation teams and ongoing optimization assistance. For voice-based applications, comparing providers like Vapi AI, Air AI, and Retell AI against capabilities offered by Callin.io illustrates the range of available options and distinctive approaches. Organizations should prioritize evaluation criteria based on specific business requirements and implementation objectives.
Implementation Timeline: Planning Your White Label AI Rollout
Successful deployment of white label AI solutions follows a structured implementation sequence requiring careful planning and milestone establishment. The process typically begins with a thorough requirements analysis phase (2-4 weeks) documenting specific business objectives, technical integration points, customization requirements, and success metrics that will guide the implementation. This leads to solution configuration (3-6 weeks), encompassing visual branding application, conversation flow development, knowledge base integration, and system behavior customization aligned with business processes. Integration testing (2-3 weeks) then validates connections with existing systems, data exchange processes, and user authentication mechanisms before proceeding to pilot deployment. Limited production testing with selected users or departments (4-6 weeks) provides real-world validation while allowing iterative refinement based on actual usage patterns and feedback. Full deployment typically follows successful pilot completion, accompanied by user training, support process establishment, and monitoring system activation. For organizations implementing AI call center solutions, phased implementation approaching one department or function at a time often proves most successful.
ROI Analysis: Measuring White Label AI Business Impact
Comprehensive evaluation of white label AI investments requires multidimensional ROI assessment examining both financial returns and operational improvements. Direct cost reduction typically appears most immediately in decreased staffing requirements for routine tasks, reduced training expenses, and lower infrastructure costs compared to maintaining 24/7 human coverage. Revenue enhancement effects frequently emerge through improved lead conversion rates resulting from consistent follow-up, increased upsell opportunities identified through conversation analysis, and expanded service hours enabling business outside traditional timeframes. Customer experience improvements manifest in metrics like reduced wait times, increased first-contact resolution rates, and higher satisfaction scores, contributing to improved retention and lifetime value. For businesses implementing AI sales representatives, performance analysis should track conversion metrics against historical human-only baselines. Implementation typically demonstrates a J-curve ROI pattern, with initial investment followed by accelerating returns as the system learns and processes optimize. Organizations should establish clear measurement frameworks before implementation, including both leading indicators providing early performance insights and lagging metrics demonstrating long-term business impact.
Future-Proofing Your White Label AI Strategy
Establishing lasting competitive advantage through white label AI requires forward-looking approaches addressing technological evolution, market dynamics, and customer expectations. Architectural flexibility represents the foundation of future-proofing, favoring platforms with modular design, standards-based integration, and provider-managed updates that incorporate emerging capabilities without disruption. Continuous learning mechanisms—both technical systems that improve through interaction analysis and organizational processes capturing implementation insights—enable progressive enhancement rather than periodic replacement cycles. Data strategy plays an equally critical role, as systematically collected interaction information fuels ongoing improvement while creating proprietary assets that increase in value over time. Organizations should establish technology radar processes monitoring developments like specialized language models and advanced voice synthesis to inform update planning and competitive positioning. Partnership selection deserves particular attention, evaluating providers not only on current capabilities but also on innovation investment, enhancement frequency, and strategic roadmap alignment with your business direction. Resources like AI phone agent implementation guides can provide valuable insights into emerging practices and technology directions.
Building Customer Trust with White Label AI Solutions
Establishing and maintaining customer confidence represents perhaps the most critical success factor for white label AI implementations. Transparency forms the foundation of trust-building, requiring clear disclosure of AI system use, straightforward explanation of capabilities and limitations, and honest communication about information handling practices. Setting appropriate expectations proves equally important, avoiding overstatement of system capabilities while highlighting genuine advantages like consistent service and round-the-clock availability. Human backup availability remains essential for complex situations exceeding AI capabilities, with seamless transition paths preventing customer frustration when escalation becomes necessary. Organizations implementing AI voice assistants should develop explicit language explaining when and how human intervention occurs. Continuous quality monitoring systems should prioritize identifying potential trust-eroding interactions—such as misunderstood requests or inappropriate responses—for immediate remediation and process improvement. The most successful implementations establish governance frameworks specifically addressing trust considerations across system design, implementation approach, and operational management.
Leveraging AI Calling Technology for Your Business Growth
Transforming your business communications through white label AI calling technology represents a strategic opportunity for operational efficiency, customer experience enhancement, and competitive differentiation. When properly implemented, these systems deliver substantial advantages through automated yet natural customer interactions handling appointment scheduling, frequently asked questions, lead qualification, and routine service requests with remarkable consistency and availability. For businesses weighing implementation options, Callin.io offers a comprehensive platform combining sophisticated conversational intelligence with straightforward customization and integration capabilities. The system’s voice synthesis technology creates natural, engaging interactions while machine learning continuously improves performance through conversation analysis.
If you’re ready to revolutionize your business communications with AI technology, Callin.io provides the ideal starting point. Their platform allows you to implement AI telephone agents that autonomously handle incoming and outgoing calls. With their innovative AI phone agent, you can automate appointments, answer common questions, and even close sales through natural customer interactions.
The free Callin.io account offers an intuitive interface for configuring your AI agent, including test calls and access to the task dashboard for monitoring interactions. For those seeking advanced features like Google Calendar integrations and built-in CRM capabilities, subscription plans start at just $30 USD monthly. Discover how Callin.io can transform your business communications today.
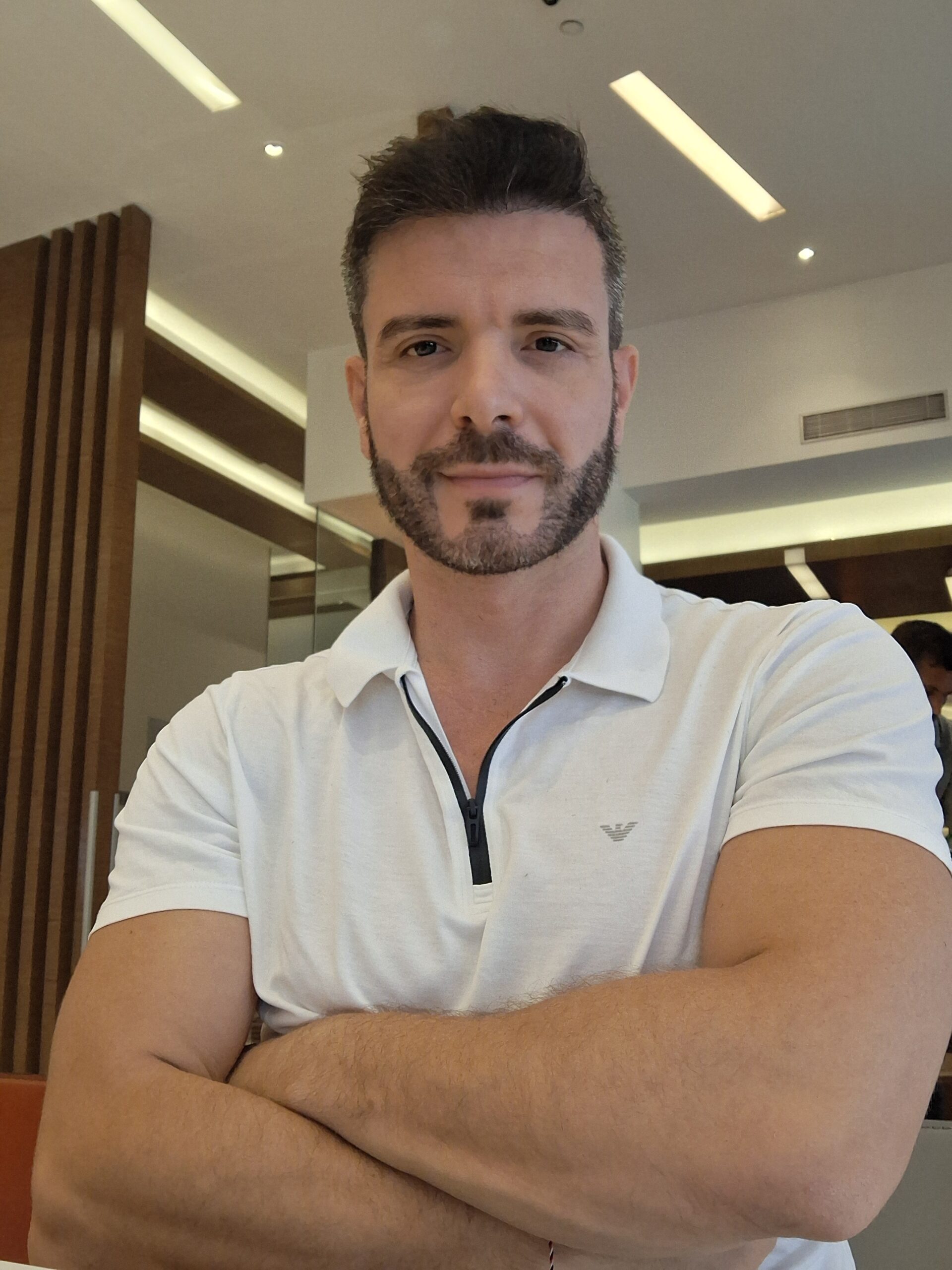
Helping businesses grow faster with AI. 🚀 At Callin.io, we make it easy for companies close more deals, engage customers more effectively, and scale their growth with smart AI voice assistants. Ready to transform your business with AI? 📅 Let’s talk!
Vincenzo Piccolo
Chief Executive Officer and Co Founder