Understanding the Foundations of AI Healthcare Integration
The implementation of AI in healthcare represents one of the most significant technological advancements in medical history. Unlike sporadic tech upgrades of the past, artificial intelligence has fundamentally altered how healthcare providers diagnose conditions, treat patients, and manage medical data. This integration isn’t merely about replacing human judgment; rather, it’s about augmenting clinical decision-making with computational power that can process vast datasets at unprecedented speeds. Healthcare institutions worldwide have begun embedding AI tools into their daily operations, creating systems that can detect patterns invisible to the human eye. For instance, the Mayo Clinic’s partnership with Google Cloud has established a framework for using AI to improve diagnostic accuracy by analyzing millions of patient records while maintaining stringent privacy protocols. These foundations mirror similar developments in other service sectors, where conversational AI platforms like those used in medical offices have demonstrated remarkable efficiency improvements.
AI-Powered Diagnostic Revolution: From Images to Insights
The diagnostic realm has witnessed perhaps the most immediate impact of healthcare AI deployment. Advanced image recognition algorithms can now scrutinize radiological scans with precision that rivals—and sometimes exceeds—that of experienced radiologists. These systems excel particularly with chest X-rays, mammograms, and brain MRIs, identifying subtle anomalies that might escape human attention. For example, Google Health’s AI system has demonstrated 99% accuracy in detecting metastatic breast cancer from lymph node tissue samples—performance that meets or exceeds pathologists’ capabilities. Similarly, Stanford University researchers have developed algorithms that can identify skin cancers with dermatologist-level accuracy using only smartphone photos. This diagnostic revolution extends beyond medical imaging into laboratory medicine, where AI systems like those from Twilio’s AI assistants can process and interpret blood test results with remarkable speed and consistency, allowing for earlier disease detection and intervention.
Patient Engagement Through Conversational AI Interfaces
The integration of AI-driven communication tools has dramatically transformed patient engagement across the healthcare continuum. Virtual nursing assistants and AI chatbots now serve as front-line responders for routine inquiries, symptom assessments, and medication reminders. These systems operate around the clock, providing timely information and reducing the burden on clinical staff. Notable implementations include Providence St. Joseph Health’s AI assistant "Grace," which guides patients through appropriate care channels based on symptom descriptions. Similarly, Intermountain Healthcare has deployed a virtual assistant that helps patients navigate insurance questions and appointment scheduling. The technology behind these tools bears striking similarities to AI voice agents used in commercial customer service, but with specialized medical knowledge bases and clinical protocols embedded in their architecture. By handling routine interactions, these systems free up healthcare professionals to focus on complex cases requiring human expertise and empathy.
Operational Excellence Through Intelligent Workflow Management
Healthcare administration has embraced AI to tackle inefficiencies in scheduling, billing, and resource allocation. Machine learning algorithms now predict patient flow patterns, allowing hospitals to optimize staffing levels and reduce wait times. At Johns Hopkins Hospital, an AI system called Capacity Command Center analyzes 500+ variables in real-time to coordinate patient transfers, predict bed availability, and manage operating room schedules. This application has reduced emergency department boarding by 20% and transfer delays from other hospitals by 70%. Similarly, Cleveland Clinic employs predictive analytics to forecast patient no-shows, enabling proactive intervention and rescheduling. These administrative applications mirror capabilities found in AI call center solutions but tailored specifically for healthcare’s unique operational challenges. The result is smoother patient journeys, reduced administrative overhead, and more efficient use of limited healthcare resources.
Precision Medicine: AI-Tailored Treatment Paths
The promise of personalized medicine has found a powerful ally in artificial intelligence. By analyzing genetic information, biomarkers, lifestyle factors, and treatment outcomes across vast patient populations, AI systems can help identify which interventions will most likely benefit specific individuals. At Memorial Sloan Kettering Cancer Center, the Watson for Oncology platform examines patient data against clinical expertise and medical literature to recommend tailored cancer treatments. Similarly, Massachusetts General Hospital utilizes machine learning to predict antibiotic resistance patterns, enabling physicians to select optimal antimicrobial therapy for each patient. These applications represent a fundamental shift from traditional "one-size-fits-all" treatment approaches toward precision medicine that considers individual variability. The implementation of such systems requires sophisticated data integration capabilities similar to those used in AI appointment scheduling but applied to complex medical decision-making.
Data Security and Ethical Considerations in Healthcare AI
The implementation of artificial intelligence in healthcare brings unprecedented data security and ethical challenges. Medical information is among the most sensitive personal data, requiring robust protection while simultaneously needing to be accessible for AI training and operation. Healthcare organizations must navigate stringent regulations like HIPAA in the US and GDPR in Europe while deploying AI solutions. Massachusetts General Hospital and MIT researchers have pioneered federated learning approaches that allow AI models to learn from distributed datasets without centralizing sensitive information. Beyond technical safeguards, ethical questions abound regarding algorithmic bias, transparency, and accountability. The American Medical Association has established guiding principles for healthcare AI that emphasize the need for clinician oversight and clear disclosure when AI influences medical decisions. These considerations parallel concerns in other sectors using AI voice conversation technologies but with higher stakes given healthcare’s direct impact on human wellbeing.
Remote Patient Monitoring Enhanced by AI Analytics
The fusion of wearable technology with AI analytics has revolutionized remote patient monitoring, particularly for chronic conditions. Smart devices continuously collect vital signs, activity levels, and other health metrics, while AI algorithms analyze this data stream to detect concerning patterns or deterioration before patients themselves notice symptoms. Providers like Kaiser Permanente have implemented remote monitoring programs for congestive heart failure patients that use AI to identify subtle changes in weight, blood pressure, and other indicators that might signal impending exacerbation. When warning signs appear, the system can alert care teams or even patients directly. Similarly, Ochsner Health System’s "Digital Medicine" program for hypertension management combines connected blood pressure cuffs with AI analysis to adjust medication regimens without requiring office visits. These applications demonstrate how AI extends clinical oversight beyond facility walls, similar to how AI calling solutions have expanded business reach, but applied specifically to continuous health monitoring.
Surgical Innovation Through AI Assistance
The operating room has become another frontier for AI implementation in healthcare. Computer vision systems now assist surgeons by analyzing real-time video feeds from laparoscopic cameras, highlighting anatomical structures and potential hazards. At Johns Hopkins, researchers have developed an AI system that helps surgeons identify critical structures during robot-assisted procedures, reducing the risk of accidental damage. Meanwhile, companies like Activ Surgical have created platforms that enhance visualization during surgery by overlaying blood flow information invisible to the naked eye. Beyond direct surgical assistance, AI systems analyze historical surgical data to identify best practices and predict complications. For instance, researchers at Massachusetts General Hospital have developed algorithms that can predict the need for ICU care following surgery based on intraoperative vital signs. These innovations parallel developments in AI-assisted customer service, but applied to the high-stakes environment of surgical intervention.
Pharmaceutical Research Acceleration Via AI Modeling
Drug discovery and development have traditionally required decades of work and billions in investment. Artificial intelligence has begun dramatically compressing these timelines by predicting molecular behavior, identifying promising compounds, and optimizing clinical trial design. Companies like Insilico Medicine have used generative adversarial networks to design novel molecules targeting specific disease mechanisms; their AI system recently identified a previously unknown compound for idiopathic pulmonary fibrosis that progressed from conception to preclinical candidate in just 18 months instead of the usual 3-5 years. Similarly, BenevolentAI’s platform has identified existing drugs that could be repurposed for new conditions, including baricitinib for COVID-19 treatment. AI also enhances clinical trial processes by identifying suitable participants, predicting drop-out risks, and detecting safety signals earlier. These capabilities mirror the data-processing advantages seen in AI call assistant technologies but applied specifically to accelerate the pharmaceutical research pipeline.
Integration Challenges: Systems, Staff, and Stakeholder Adoption
Despite its promise, the implementation of AI in healthcare faces substantial integration hurdles. Legacy electronic health record systems often operate in isolated silos, making data aggregation for AI training difficult. Technical challenges include standardizing data formats across diverse sources and ensuring interoperability between AI tools and existing clinical systems. Human factors present equally significant barriers. Clinician skepticism, workflow disruption concerns, and varying levels of digital literacy can hamper adoption. Organizations like Cleveland Clinic have addressed these challenges through comprehensive change management approaches that include clinician champions, phased implementation, and robust training programs. Patient acceptance also varies widely depending on demographic factors and the specific AI application. A survey by Accenture found that while 75% of patients were comfortable with AI analyzing their medical data, only 28% wanted AI directly involved in treatment decisions. These integration challenges parallel those faced when implementing AI voice assistants for FAQ handling but with added complexity due to healthcare’s regulatory environment and clinical implications.
Economic Impact and Healthcare Cost Containment
The economic implications of healthcare AI extend throughout the system. Initial implementation requires significant investment in technology infrastructure, data preparation, and staff training. However, long-term benefits include substantial cost reductions through improved efficiency, reduced errors, and earlier interventions. Accenture estimates that key healthcare AI applications could save the US healthcare economy $150 billion annually by 2026. These savings derive from multiple sources: AI-driven triage systems redirect patients to appropriate care levels, predictive analytics prevent costly complications, and automation reduces administrative overhead. For instance, the University of Pittsburgh Medical Center deployed an AI system to identify patients at high risk for readmission, enabling targeted interventions that reduced readmission rates by 40% and saved millions in associated costs. Similarly, Humana’s AI-powered medication adherence program improved compliance rates while reducing emergency department visits and hospitalizations. These economic benefits mirror cost efficiencies seen with AI phone agents in commercial settings but potentially with even greater impact given healthcare’s significant economic footprint.
Global Health Equity and AI Implementation Access
The global distribution of healthcare AI technologies raises important questions about equitable access and potential exacerbation of existing healthcare disparities. While high-resource settings rapidly adopt sophisticated AI systems, many facilities in low and middle-income countries lack even basic digital infrastructure. Organizations like PATH have begun addressing this gap by developing AI tools specifically designed for resource-constrained environments, such as smartphone-based diagnostic apps that don’t require constant internet connectivity. The World Health Organization has established working groups to develop frameworks for equitable AI deployment in healthcare globally. Promising models include public-private partnerships like the one between Novartis Foundation and Ghana Health Service, which implemented an AI-powered screening system for hypertension in rural communities. These initiatives demonstrate how thoughtfully implemented AI can actually reduce healthcare disparities rather than widen them. The challenges mirror those seen in scaling AI calling services to diverse markets but with even greater implications for human health and wellbeing across different socioeconomic contexts.
Predictive Analytics for Population Health Management
The implementation of predictive analytics powered by AI has transformed population health management from reactive to proactive approaches. County health departments and integrated delivery networks now deploy machine learning models that analyze demographic data, social determinants, clinical records, and environmental factors to identify at-risk individuals and communities before crises emerge. Kaiser Permanente’s predictive model examines 200+ variables to identify members at highest risk for developing chronic conditions, enabling early intervention programs that have reduced hospital admissions by 30% in target populations. Similarly, Chicago’s Department of Public Health uses an AI system to predict which children are most likely to develop lead poisoning based on housing data, allowing preventive home inspections. These applications demonstrate AI’s ability to shift healthcare focus from treatment to prevention. The underlying technology shares similarities with predictive systems used in AI sales representatives but applied specifically to population health patterns and public health interventions.
Natural Language Processing for Clinical Documentation
The burden of clinical documentation has long contributed to healthcare provider burnout. Natural language processing (NLP) applications now offer relief by automatically generating clinical notes from doctor-patient conversations. Systems like Nuance’s Dragon Ambient eXperience (DAX) use sophisticated AI to listen to clinical encounters, distinguish relevant medical information, and create structured documentation that integrates with electronic health records. Early adopters like Novant Health report that physicians using these tools save 2+ hours daily on documentation while producing more comprehensive and accurate notes. Similarly, Beth Israel Deaconess Medical Center employs NLP to analyze unstructured clinical notes for important information that might otherwise be missed during handoffs. These applications free clinicians to focus on patient care rather than administrative tasks. The underlying technology shares capabilities with conversational AI systems used in business settings but adapted specifically for medical terminology and documentation requirements.
Telemedicine Enhancement Through AI Capabilities
The explosive growth of telemedicine during the COVID-19 pandemic created new opportunities for AI implementation in virtual care delivery. AI-powered symptom checkers now serve as first-line triage tools for telehealth platforms, directing patients to appropriate care levels based on reported symptoms. Companies like Babylon Health have developed diagnostic algorithms that compare patient-reported symptoms against millions of data points to suggest possible conditions and next steps. During virtual visits, AI tools can analyze speech patterns, facial expressions, and other subtle cues to provide additional diagnostic information to clinicians. For instance, Winterlight Labs has developed algorithms that detect cognitive impairment by analyzing speech patterns during virtual cognitive assessments. After encounters, AI summarization tools extract key points from telemedicine visits to ensure important details aren’t missed and follow-up actions are taken. These enhancements make virtual care more effective while preserving the convenience and access benefits of telemedicine. The technology parallels developments in virtual call systems but specifically optimized for clinical interactions and medical decision support.
Mental Health Interventions Powered by AI
Mental healthcare has embraced artificial intelligence to extend limited provider resources and offer innovative treatment approaches. Conversational agents specifically designed for psychological support now provide cognitive behavioral therapy techniques to patients with mild to moderate depression and anxiety. Woebot, developed by clinical psychologists at Stanford, delivers evidence-based interventions through natural language conversations, with studies showing significant symptom reduction among users. For providers, AI tools analyze speech, text, and behavioral patterns to identify patients at risk for self-harm or suicide, enabling timely intervention. The Department of Veterans Affairs employs an AI system called REACH VET that identifies veterans at elevated suicide risk based on electronic health record data, facilitating proactive outreach. Even traditional therapy benefits from AI augmentation; systems like Ellie (developed by USC’s Institute for Creative Technologies) can analyze subtle facial expressions and vocal tones during therapy sessions to help clinicians identify emotional states patients may not verbalize. These applications demonstrate AI’s unique capacity to address the growing global mental health crisis. The underlying technology shares characteristics with AI bots used in customer service but specifically designed with psychological expertise and clinical safeguards.
Radiology Workflow Transformation Through AI Analysis
Radiology departments have experienced remarkable workflow transformation through AI implementation. Intelligent worklist prioritization systems now analyze incoming imaging studies to flag critical findings like intracranial hemorrhages or pulmonary embolisms, ensuring urgent cases receive immediate attention. Mount Sinai Health System has implemented an AI platform that reprioritizes radiological exams based on detected abnormalities, reducing critical finding turnaround times by 96 minutes on average. Beyond triage, AI tools now serve as radiologists’ "second readers," highlighting suspicious areas for closer examination. The University of Chicago Medical Center uses AI-assisted chest X-ray analysis that increased detection rates of clinically significant findings by 22% compared to conventional review. These tools don’t replace radiologists but rather augment their capabilities, allowing them to work more efficiently and focus on complex interpretations. The technology shares computational vision capabilities with AI voice agents but applied specifically to medical image analysis and radiological workflow optimization.
Emergency Medicine and Critical Care AI Applications
Emergency departments and intensive care units represent particularly challenging healthcare environments where rapid decision-making can dramatically affect outcomes. AI implementation in these settings focuses on real-time monitoring and predictive analytics. Advanced monitoring systems continuously analyze multiple vital sign parameters to detect subtle deterioration patterns before traditional alarms would trigger. The University of Pennsylvania Health System deployed an AI-based early warning system that reduced cardiac arrests outside the ICU by 30% by identifying at-risk patients hours before clinical decompensation. In emergency departments, predictive models help manage patient flow by forecasting visit volumes and acuity levels 24-48 hours in advance, enabling proactive staffing adjustments. Banner Health’s ED AI system predicts hourly patient arrivals with 90% accuracy, significantly reducing wait times during peak periods. For critical care decisions, AI systems integrate laboratory values, vital signs, medication data, and clinical notes to provide decision support for complex cases. These applications demonstrate how AI can enhance human capabilities in high-stress, time-sensitive clinical environments. The underlying technology shares similarities with call center voice AI systems but specifically engineered for the life-and-death decisions of emergency and critical care.
Healthcare Education and Training Augmented by AI
Medical education has been transformed by AI-powered simulation and personalized learning tools. Virtual patients powered by natural language processing now allow medical students to practice diagnostic reasoning and communication skills in safe, standardized environments. NYU Grossman School of Medicine has implemented AI-driven virtual patient encounters that adapt to student responses, creating realistic clinical scenarios without risking actual patient wellbeing. For practicing clinicians, AI-based systems analyze performance during simulated procedures to provide objective feedback on technique and decision-making. Advanced haptic simulators combined with machine learning at Stanford’s Medical Simulation Center offer surgeons personalized recommendations for improving specific surgical movements based on comparison with expert technique databases. Beyond individual training, AI tools now help healthcare organizations identify knowledge gaps across staff and deliver targeted continuing education. These applications demonstrate how AI implementation enhances clinical education through personalization, objective assessment, and expanded practice opportunities. The technology shares capabilities with AI phone consultants but specifically designed for medical education and clinical skill development.
Future Directions: Quantum Computing and Healthcare AI Synergies
The future of healthcare AI implementation points toward integration with quantum computing, creating unprecedented computational capabilities for solving previously intractable medical problems. While current AI systems already process vast datasets, quantum computing promises exponential acceleration in complex biological modeling. Early research collaborations between quantum computing pioneers and pharmaceutical companies are exploring protein folding simulations that could revolutionize drug discovery. IBM and Cleveland Clinic have established a quantum computing center specifically focused on healthcare applications, including genomic analysis and drug interaction modeling. Quantum machine learning algorithms may eventually enable real-time analysis of complete human genomes during routine office visits, allowing truly personalized treatment recommendations. While full-scale quantum computing remains developmental, hybrid quantum-classical systems are already demonstrating advantages in specific healthcare AI applications like molecular dynamics simulations. These emerging synergies represent the leading edge of healthcare technology, promising capabilities far beyond today’s most advanced systems. This future direction shares the forward-looking innovation seen in creating custom LLMs but applied specifically to the intersection of quantum computing and healthcare’s most challenging computational problems.
Regulatory Frameworks and Governance for Healthcare AI
The rapid implementation of AI in healthcare has prompted regulatory bodies worldwide to develop new frameworks for evaluating safety, efficacy, and ethical deployment of these technologies. In the United States, the FDA has established a Digital Health Center of Excellence to streamline regulatory processes for AI-based medical devices while ensuring appropriate oversight. Their proposed regulatory framework includes monitoring throughout the product lifecycle to address the unique challenges posed by continuously learning algorithms. The European Medicines Agency has similarly created guidelines specifically for AI-based medical devices under their MDR/IVDR regulations. Healthcare organizations have responded by developing internal AI governance committees that establish policies for algorithm validation, monitoring for bias, and appropriate clinical integration. Mayo Clinic’s AI oversight structure includes multidisciplinary review of all algorithms before clinical deployment, with continuous performance monitoring against predefined metrics. These governance frameworks balance innovation with patient safety by ensuring appropriate validation while avoiding unnecessary barriers to beneficial technologies. The regulatory considerations parallel those faced when establishing AI calling businesses but with additional complexities due to direct patient impact and existing healthcare regulatory structures.
Transforming Healthcare Delivery with AI: Your Next Step
The implementation of AI in healthcare is fundamentally reconfiguring medical practice and patient care across every specialty and setting. From diagnostic accuracy and administrative efficiency to personalized treatment and expanded access, these technologies offer solutions to healthcare’s most pressing challenges. However, successful implementation requires thoughtful planning, robust governance, and ongoing evaluation. Healthcare organizations that approach AI adoption strategically—with clear problem identification, appropriate technology selection, and comprehensive change management—stand to gain significant advantages in both clinical outcomes and operational performance. As AI capabilities continue advancing, the gap between early adopters and laggards will likely widen, making thoughtful implementation increasingly important for healthcare providers of all sizes. If you’re looking to enhance your healthcare organization’s communication capabilities specifically, Callin.io offers AI-powered phone agents that can streamline appointment scheduling, answer common patient questions, and improve accessibility while maintaining the human touch that remains essential in healthcare.
Elevate Your Healthcare Communication with AI-Powered Solutions
If you’re ready to transform how your healthcare organization communicates with patients, Callin.io offers cutting-edge solutions designed specifically for medical environments. Our AI phone agents can seamlessly handle appointment scheduling, insurance verification, prescription refill requests, and routine inquiries—all while maintaining natural conversational flow that patients appreciate. This technology integrates smoothly with existing electronic health record systems and practice management software through secure APIs.
The account setup process is straightforward, with a free tier that allows you to test functionality with included trial calls before full deployment. Our healthcare-specific AI agents understand medical terminology and can be configured to follow your exact protocols for patient interaction. For practices seeking more advanced features like Google Calendar integration and CRM connectivity, premium plans start at just $30 USD monthly.
By implementing Callin.io’s AI communication tools, your clinical staff can focus their attention on complex care needs rather than routine administrative calls. Discover how our healthcare-optimized AI phone solutions can enhance patient experience while improving operational efficiency by visiting Callin.io today.
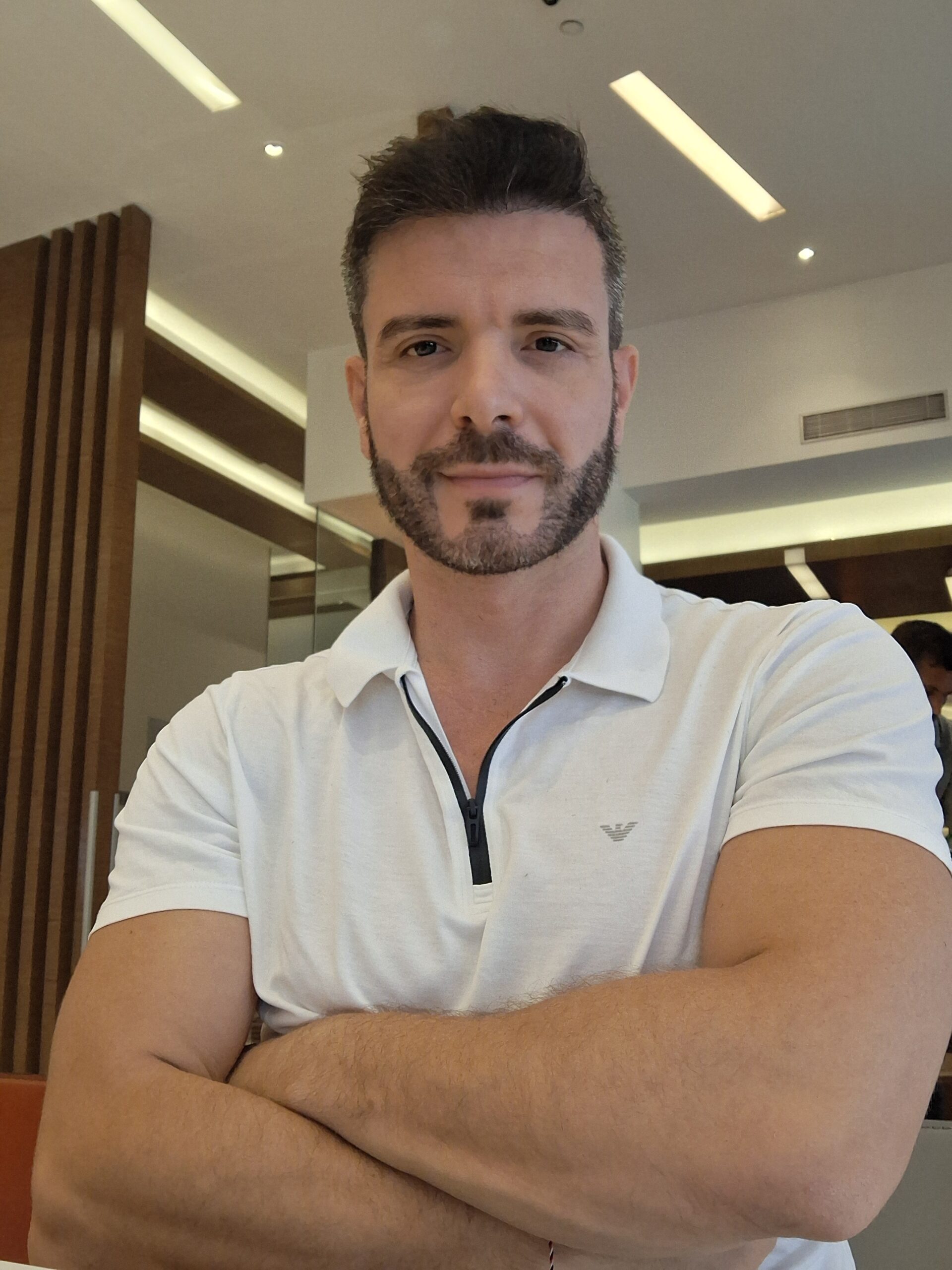
Helping businesses grow faster with AI. 🚀 At Callin.io, we make it easy for companies close more deals, engage customers more effectively, and scale their growth with smart AI voice assistants. Ready to transform your business with AI? 📅 Let’s talk!
Vincenzo Piccolo
Chief Executive Officer and Co Founder