Understanding the AI Implementation Landscape
Artificial intelligence has moved from being a theoretical concept to an essential business tool across industries. Implementing AI in your organization isn’t just about staying current—it’s about gaining tangible advantages in efficiency, customer service, and decision-making. Before diving into the technical aspects of AI adoption, businesses need to develop a clear understanding of what AI implementation actually entails. This means recognizing that AI isn’t a one-size-fits-all solution, but rather a collection of technologies and methodologies that can be tailored to specific business challenges. According to research by McKinsey, companies that strategically implement AI report up to 40% higher productivity in the functions where it’s deployed. The goal isn’t to implement AI for its own sake, but to address specific business needs—whether that’s automating repetitive tasks, enhancing customer interactions, or uncovering insights from data that would otherwise remain hidden. For businesses exploring conversational AI for their office environments, the benefits can be particularly transformative in streamlining communications and improving customer satisfaction.
Assessing Your Business Readiness for AI
Before investing in AI technologies, it’s crucial to evaluate your organization’s readiness. This assessment involves examining your current technological infrastructure, data quality and accessibility, staff capabilities, and organizational culture. Companies frequently underestimate the importance of having clean, structured data—the lifeblood of effective AI systems. Without high-quality data, even the most sophisticated AI tools will deliver subpar results. Beyond technical considerations, your team’s openness to technological change plays a decisive role in successful implementation. Research from Harvard Business Review suggests that organizations with a strong change management approach are 6 times more likely to meet their AI implementation objectives. This preparation phase should include documenting your existing processes in detail, identifying potential AI application areas, and calculating the anticipated return on investment. For companies looking to incorporate AI phone services, this assessment phase is essential to ensure that the infrastructure can support the advanced communication tools that will be introduced.
Identifying High-Value AI Use Cases
Successful AI implementation begins with selecting the right use cases—those that address significant business challenges while offering measurable returns. Instead of attempting to revolutionize your entire organization at once, focus on specific, high-impact areas where AI can deliver clear value. Customer service often presents excellent opportunities, with AI call assistants reducing response times and improving satisfaction rates. Sales departments benefit from predictive analytics that prioritize leads likely to convert. Operations teams can leverage process automation to reduce costs and errors. When identifying potential use cases, prioritize them based on business impact, implementation complexity, and available resources. Document the current metrics of processes you’re targeting for improvement, so you can later measure AI’s actual impact. For example, many businesses are finding significant value in AI appointment scheduling systems that reduce no-shows while freeing staff from manual booking tasks.
Developing a Strategic AI Roadmap
Creating a comprehensive AI implementation roadmap provides direction and ensures alignment with broader business objectives. This strategic plan should outline short-term quick wins alongside longer-term transformational projects. Start with a 12-24 month horizon, detailing specific initiatives, required resources, key milestones, and success metrics. The roadmap should explicitly connect AI initiatives to overarching business goals, whether that’s increased revenue, improved customer satisfaction, or operational efficiency. Include provisions for regularly reviewing and adjusting the roadmap as implementation progresses and new opportunities emerge. The AI field evolves rapidly, so building flexibility into your strategy is essential. Companies implementing AI voice conversations have found that a phased approach—starting with simple customer inquiries before expanding to more complex interactions—yields the best results. This methodical progression allows for adjustment and learning at each stage, minimizing disruption while maximizing adoption.
Building the Right Team Structure
The human element is often the determining factor in AI implementation success. Creating the right team structure involves a mix of technical expertise and business knowledge. While data scientists and machine learning engineers provide the technical foundation, business analysts who understand operational challenges are equally important. Consider whether to build in-house capabilities, partner with external vendors, or adopt a hybrid approach. The right choice depends on your implementation timeline, budget constraints, and the strategic importance of AI to your business. For ongoing success, establish clear governance structures defining roles, responsibilities, and decision-making processes for AI initiatives. Organizations that create dedicated AI centers of excellence often achieve faster implementation and better results than those attempting more fragmented approaches. When implementing specialized solutions like white label AI receptionists, having team members who understand both customer service workflows and AI capabilities becomes particularly valuable.
Ensuring Data Quality and Accessibility
AI systems are only as good as the data they’re trained on. Before implementation, conduct a comprehensive data audit to assess quality, completeness, and relevance. Establish data cleansing procedures to address issues like duplicate records, inconsistent formats, or missing information. Creating a unified data architecture that connects previously siloed information allows AI systems to generate more valuable insights. When implementing conversational AI systems, for example, access to customer interaction history significantly improves the quality of automated responses. Beyond quality, address data governance concerns including privacy regulations, security requirements, and ethical considerations. The European Union’s GDPR and California’s CCPA have raised the stakes for organizations handling personal data, making compliance a prerequisite for AI implementation. Developing clear data policies and procedures provides the foundation for trustworthy AI systems that deliver value without creating new risks.
Choosing the Right AI Technologies
The AI technology landscape is vast and constantly evolving, making technology selection a critical decision point. Different business challenges require different AI approaches—from machine learning for pattern recognition to natural language processing for text analysis or computer vision for image interpretation. When evaluating potential solutions, consider factors including performance capabilities, scalability requirements, integration complexity with existing systems, and total cost of ownership. Although proprietary solutions often offer simplified implementation, open-source alternatives can provide greater customization flexibility and cost advantages. For specialized applications like AI cold calling, evaluate whether the solution can handle the nuances of natural conversation and integrate with your existing customer relationship management systems. Technology selection should align with both immediate use cases and longer-term AI ambitions, creating a foundation that can expand as your organization’s AI maturity grows.
Planning for Integration with Existing Systems
Successful AI implementation rarely happens in isolation—it requires thoughtful integration with existing business systems and processes. Before implementation begins, map the required data flows between AI solutions and current systems like CRM platforms, ERP systems, or customer service tools. Assess whether current APIs are sufficient or if custom integration development will be needed. The integration plan should include provisions for both technical compatibility and process alignment, ensuring that AI outputs flow seamlessly into existing workflows. When implementing technologies like AI voice agents, integration with telephony systems and customer databases becomes particularly critical to delivering a coherent customer experience. Establish testing protocols to validate integrations before full deployment, and create contingency plans for addressing integration challenges. Companies that overlook integration complexity often face implementation delays and reduced adoption, regardless of the underlying AI technology’s quality.
Creating a Budget and Resource Allocation Plan
Realistic budgeting for AI implementation requires understanding both direct costs (software licensing, hardware infrastructure, external services) and indirect expenses (staff training, process changes, opportunity costs during transition). Development costs vary widely based on complexity—from relatively modest investments for implementing pre-built solutions to substantial commitments for custom AI development. Beyond the initial implementation, account for ongoing expenses including infrastructure maintenance, model retraining, and continuous improvement initiatives. When calculating return on investment, consider both hard benefits (reduced operational costs, increased revenue) and soft advantages (improved customer satisfaction, faster decision making). Organizations implementing call center voice AI often discover that while initial setup costs are significant, the long-term savings in staffing and training expenses create compelling ROI. Create a phased budget that aligns with your implementation roadmap, allowing for adjustment as early projects demonstrate actual costs and benefits.
Developing a Change Management Strategy
Even the most technically sound AI implementation can fail without effective change management. Resistance to AI often stems from misconceptions about job displacement or skepticism about system capabilities. Addressing these concerns requires transparent communication about how AI will augment rather than replace human workers. Create a comprehensive change management plan including awareness building, training programs, and ongoing support mechanisms. Include both technical training on new systems and conceptual education about how AI functions and its limitations. Early involvement of end users in the implementation process increases acceptance and provides valuable feedback for refinement. For implementations like AI call center solutions, allowing agents to experience the technology’s benefits firsthand can transform skeptics into advocates. The most successful organizations designate AI champions within departments who can provide peer-to-peer support and gather feedback during the transition period.
Implementing Pilot Projects
Starting with focused pilot projects allows organizations to demonstrate value quickly while containing risks. Select pilot projects that balance meaningful business impact with reasonable implementation complexity. Define clear success criteria for each pilot, including specific metrics and evaluation timeframes. When implementing Twilio AI assistants or similar technologies, companies often begin with internal use cases before expanding to customer-facing applications. This approach limits potential brand damage while providing valuable learning opportunities. Structure pilots to generate insights about both technical performance and organizational readiness. Document challenges encountered and solutions developed during pilot implementation to inform wider rollouts. The most valuable pilots create templates and best practices that can be applied to subsequent implementations, accelerating the organization’s overall AI adoption journey. Following successful pilots, conduct thorough reviews to capture learnings before scaling—even seemingly small insights can prevent major issues during broader deployment.
Scaling Successful AI Implementations
After validating AI approaches through pilots, developing a structured scaling plan becomes essential. This expansion strategy should address technical scaling requirements (increased data volumes, additional computing resources, enhanced security measures) alongside organizational scaling needs (expanded training programs, adapted governance structures, revised processes). When scaling technologies like AI appointment setters, gradually increasing the volume and complexity of scheduled appointments helps maintain quality while expanding capacity. Create repeatable implementation playbooks documenting best practices, common challenges, and tested solutions from earlier deployments. These resources significantly accelerate subsequent implementations while maintaining consistency. Establish clear criteria for evaluating which additional business areas would benefit from similar AI applications. The most successful scaling approaches maintain balance between standardization (for efficiency) and customization (for effectiveness), adapting core solutions to the specific needs of different business units or customer segments.
Ensuring Ethical AI Implementation
Ethical considerations should be integrated throughout the AI implementation process, not treated as an afterthought. Develop clear ethical guidelines for AI use addressing issues like transparency, accountability, fairness, and privacy protection. When implementing conversational AI for phone calls, for example, organizations must decide how and when to disclose that customers are interacting with an AI system rather than a human agent. Implement processes to audit AI systems for biases that might disadvantage particular customer groups or lead to unfair outcomes. These reviews should occur during initial development and continue through regular auditing cycles. Establish oversight mechanisms involving diverse perspectives to evaluate complex ethical questions around AI deployment. Organizations that proactively address ethical considerations build customer trust while reducing regulatory and reputational risks. Creating channels for stakeholder feedback about ethical concerns provides early warning about potential issues and demonstrates organizational commitment to responsible AI use.
Measuring AI Implementation Success
Effective measurement frameworks evaluate both technical performance and business impact of AI implementations. Technical metrics might include accuracy rates, processing speeds, or system reliability statistics. Business metrics should tie directly to the objectives established during planning—whether that’s cost reduction, revenue growth, or customer satisfaction improvements. When measuring the impact of AI sales calls implementations, for instance, track not just call completion metrics but also conversion rates, average deal sizes, and customer sentiment scores. Establish measurement processes that capture both quantitative data and qualitative feedback from users and customers. Regular review cycles (monthly for operational metrics, quarterly for strategic outcomes) maintain implementation momentum while enabling course correction. The most sophisticated measurement approaches track how AI systems evolve over time—measuring not just point-in-time performance but learning and improvement rates. This monitoring becomes particularly important as AI systems encounter new situations or as underlying business conditions change.
Developing AI Governance Structures
As AI implementations expand across an organization, formal governance structures become increasingly important. Effective AI governance defines decision rights, accountability frameworks, and management processes for AI systems throughout their lifecycle. This governance should address questions like who approves new AI use cases, how systems are monitored for performance drift, and what review processes exist for algorithm updates. When implementing white label AI solutions across multiple business units, clear governance prevents inconsistent customer experiences while enabling appropriate customization. Create documentation standards for AI models that capture development methodologies, training data characteristics, known limitations, and testing results. These records support both internal management and potential regulatory compliance requirements. Establish clear incident response protocols for addressing AI system failures or unintended consequences. Organizations with mature AI governance find that these structures accelerate rather than hinder implementation by creating trusted frameworks within which innovation can safely occur.
Addressing AI Security Considerations
AI implementations introduce unique security considerations that extend beyond traditional IT security frameworks. Potential vulnerabilities include adversarial attacks (deliberately manipulated inputs designed to fool AI systems), data poisoning during training, and model theft. When implementing systems like AI phone numbers that handle sensitive customer information, robust security becomes particularly critical to maintaining trust. Develop comprehensive security protocols addressing the entire AI lifecycle—from data collection and model training through deployment and ongoing operations. Implement technical safeguards including access controls, encryption, and input validation while also establishing procedural controls like regular security reviews and clear responsibility assignments. Create monitoring systems to detect unusual patterns that might indicate security breaches or manipulation attempts. The most effective AI security approaches balance protection with usability, recognizing that excessive security measures can undermine system adoption and value delivery.
Providing Ongoing Training and Support
Successful AI implementation requires continuous investment in human capabilities alongside technological systems. Develop tiered training programs addressing different roles—from general awareness for all employees to specialized technical training for those directly managing AI systems. When implementing AI voice assistants, for example, customer service managers need training on supervision and exception handling, while technical staff require deeper knowledge of model maintenance and tuning. Create easily accessible resources including knowledge bases, troubleshooting guides, and best practice repositories to support ongoing implementation. Internal communities of practice where practitioners share experiences accelerate learning across the organization. Schedule regular refresher training as systems evolve and capabilities expand. The most forward-thinking organizations incorporate AI literacy into broader professional development programs, recognizing that general AI fluency has become a core business competency. This ongoing capability development ensures that technological implementations deliver sustained value rather than deteriorating over time.
Managing AI Vendor Relationships
For many organizations, partnerships with external vendors play crucial roles in AI implementation. Develop structured processes for evaluating potential partners based on technical capabilities, domain expertise, implementation support, and long-term viability. When selecting partners for specialized implementations like AI bots for businesses, examine both technical performance and ease of integration with existing systems. Create clear contractual agreements addressing questions like data ownership, model training responsibilities, performance guarantees, and exit provisions. Establish governance mechanisms for managing vendor relationships, including regular review cycles and escalation procedures for addressing issues. The most productive vendor relationships balance formal management structures with collaborative working relationships where knowledge transfers in both directions. For organizations pursuing white label AI solutions, careful vendor selection becomes particularly important as the partner’s capabilities directly impact brand reputation through customer-facing interactions.
Planning for Continuous Improvement
AI implementation is never truly complete—it requires ongoing refinement to maintain performance and incorporate new capabilities. Establish systematic processes for collecting performance data, user feedback, and enhancement suggestions. Regular review cycles should evaluate both immediate optimization opportunities and longer-term strategic enhancements. When operating AI call centers, for instance, continuous improvement might involve both refining conversation flows based on customer feedback and incorporating entirely new capabilities as technology evolves. Create clear paths for testing and deploying system updates that balance innovation with stability. The most effective continuous improvement approaches combine quantitative performance metrics with qualitative insights from users and customers. Organizations that excel at continuous improvement typically dedicate specific resources to this function rather than treating it as a side responsibility. This dedicated focus ensures that AI systems continue delivering increasing value rather than becoming technological dead ends after initial implementation.
Anticipating Future AI Developments
While addressing current implementation needs, forward-thinking organizations simultaneously prepare for emerging AI capabilities. Stay informed about technological developments through industry publications, academic research, and vendor relationships. When possible, design implementations with flexible architectures that can incorporate new capabilities without complete rebuilds. For companies using SIP trunking providers alongside AI voice systems, ensuring compatibility with evolving communication standards prevents future integration challenges. Allocate resources for limited experimentation with emerging technologies to build organizational familiarity before formal implementation projects begin. The most strategic organizations create regular technology review cycles to evaluate how emerging capabilities might address business challenges or create new opportunities. While avoiding distraction by every new development, maintain sufficient awareness to identify truly transformative technologies early in their development cycle. This balanced approach prevents both premature adoption of unproven technologies and delayed implementation of valuable new capabilities.
Transforming Your Business with AI: Next Steps
Implementing AI successfully requires disciplined execution across multiple dimensions—from technology selection and data preparation to organizational change management and continuous improvement. The journey typically progresses through distinct phases: initial exploration, focused pilots, systematic scaling, and eventually, transformation of core business operations. For businesses ready to explore practical applications, specialized solutions like AI sales representatives offer immediate value without requiring extensive technical expertise. Remember that successful implementation balances technological capabilities with human factors—the most advanced AI delivers limited value if employees don’t understand its purpose or customers don’t trust its outputs. By following the structured approaches outlined here, organizations can navigate the complexities of AI implementation while maximizing returns on their investment. The most successful implementations start with clear business objectives rather than technology fascination, maintain that focus throughout implementation, and continuously measure results against initial goals.
Elevate Your Business with Intelligent Communication Solutions
If you’re looking to transform your business communications with powerful AI technology, explore what Callin.io has to offer. This platform enables you to implement AI-powered phone agents that independently handle incoming and outgoing calls. With Callin.io’s innovative AI voice agent, you can automate appointment scheduling, answer common customer questions, and even close sales through natural, human-like conversations.
Callin.io’s free account provides an intuitive interface for setting up your AI agent, with included test calls and access to a comprehensive task dashboard for monitoring interactions. For businesses requiring advanced capabilities like Google Calendar integration and built-in CRM functionality, subscription plans start at just 30USD monthly. Discover how Callin.io can revolutionize your customer communications while reducing operational costs.
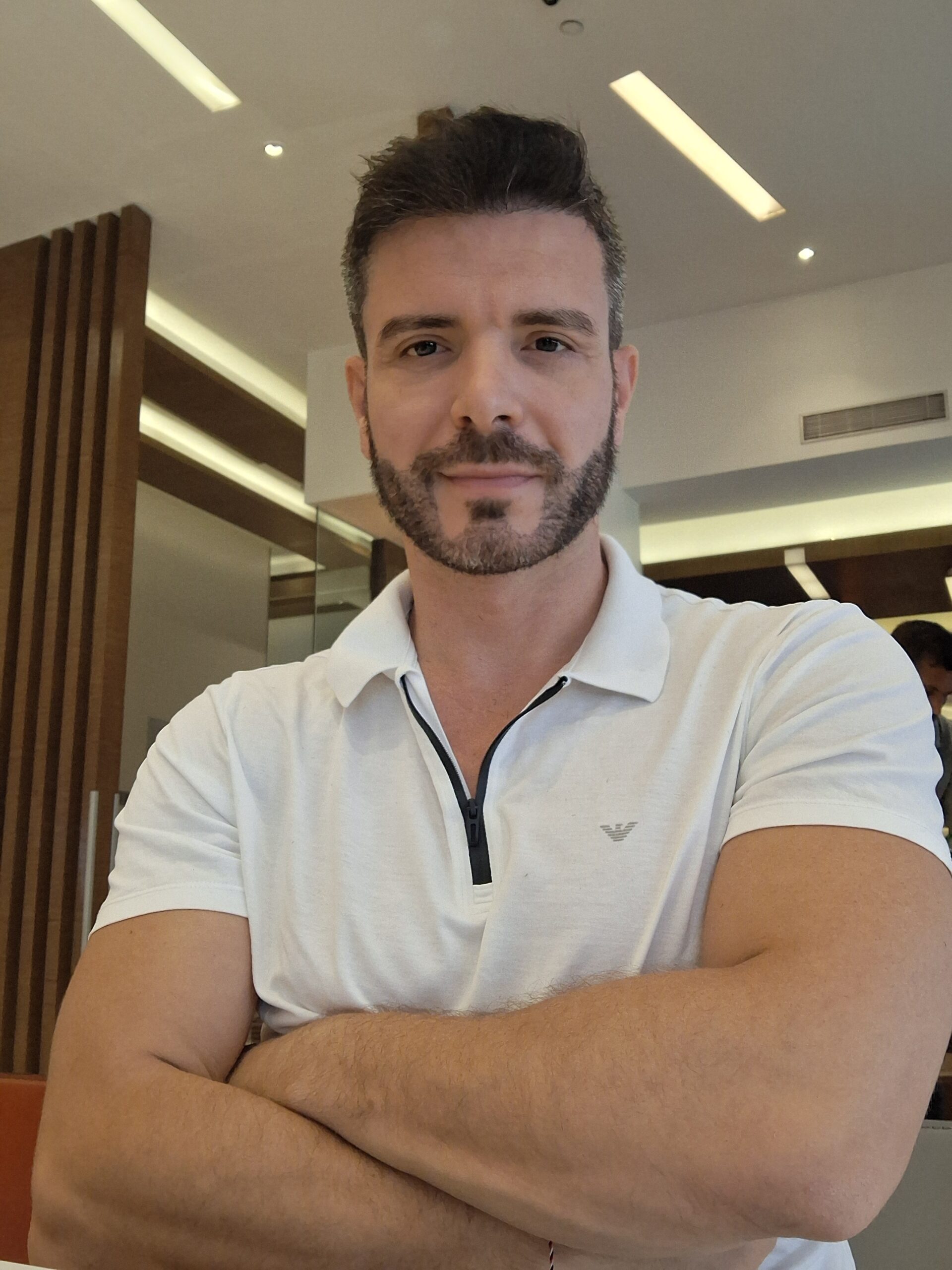
Helping businesses grow faster with AI. 🚀 At Callin.io, we make it easy for companies close more deals, engage customers more effectively, and scale their growth with smart AI voice assistants. Ready to transform your business with AI? 📅 Let’s talk!
Vincenzo Piccolo
Chief Executive Officer and Co Founder