The Promise Beyond the Hype
Healthcare systems worldwide face unprecedented pressures – from aging populations to resource constraints and staffing shortages. Artificial intelligence appears as a potential remedy, promising to revolutionize everything from diagnosis to administrative tasks. Despite the buzz, implementing AI in healthcare settings presents unique challenges that extend far beyond technical considerations. Organizations attempting to integrate these systems face regulatory hurdles, data privacy concerns, and significant cultural resistance. The transformation isn’t simply about deploying technology; it requires reimagining healthcare delivery while maintaining patient trust. As healthcare providers consider solutions like conversational AI for medical offices, understanding these implementation barriers becomes crucial for successful deployment and meaningful clinical impact.
Regulatory Labyrinths and Approval Processes
One of the most daunting challenges in healthcare AI implementation is navigating complex regulatory frameworks designed for traditional medical devices and therapies. AI systems, particularly those using machine learning with continuously evolving algorithms, pose unique regulatory questions that established frameworks struggle to address. In the United States, the FDA has introduced the Digital Health Software Precertification Program to evaluate AI/ML-based medical devices, but many gray areas remain. According to research from the Brookings Institution, regulatory bodies worldwide are struggling to develop approaches that balance innovation with patient safety. Healthcare organizations must dedicate substantial resources to regulatory compliance and approval processes, often slowing implementation timelines and increasing costs. This regulatory complexity becomes particularly challenging when attempting to integrate AI call assistants into healthcare communication channels.
Data Privacy and Security Concerns
Healthcare data represents some of the most sensitive personal information, making privacy and security paramount concerns in AI implementation. Medical organizations must ensure their AI systems comply with regulations like HIPAA in the US, GDPR in Europe, and various local privacy laws. The implementation challenge intensifies when AI systems require large datasets for training and continuous improvement, creating potential vulnerabilities for data breaches. A study published in the Journal of the American Medical Association found that even de-identified health data can sometimes be re-identified using sophisticated algorithms. These privacy concerns often create resistance from patients and healthcare staff alike, necessitating robust security protocols and transparency about data usage. Healthcare organizations exploring AI phone service solutions must carefully evaluate how patient information is processed, stored, and protected throughout the AI communication workflow.
Integration with Legacy Systems
Most healthcare institutions operate with complex IT ecosystems built over decades, including electronic health records (EHRs), billing systems, and specialized clinical applications. Integrating AI solutions with these legacy systems presents significant technical hurdles. Interoperability problems arise from proprietary data formats, outdated interfaces, and limited API capabilities. According to a survey by the Healthcare Information and Management Systems Society (HIMSS), interoperability remains one of the top challenges facing healthcare technology implementation. Organizations must often invest in custom integration solutions or middleware to connect AI systems with existing infrastructure. These integration challenges frequently extend implementation timelines and increase costs, sometimes necessitating complete system overhauls. Solutions like AI voice conversation technologies must navigate these integration complexities to deliver seamless experiences for both providers and patients.
Clinical Workflow Disruption
Healthcare delivery relies on finely-tuned workflows developed over years of practice. Introducing AI systems frequently disrupts these established processes, creating resistance and implementation challenges. Clinicians may view AI tools as adding complexity rather than simplifying their work, especially during the transition period. A study in the Journal of Medical Internet Research found that poorly integrated AI tools can increase clinician workload rather than reducing it. Successful implementation requires thorough workflow analysis and redesign, with AI systems adapted to clinician needs rather than forcing users to adapt to technology. Organizations must involve frontline healthcare workers in design and implementation decisions, providing adequate training and support through the transition. Tools like AI voice agents can support clinical workflows, but only when carefully integrated into existing processes with appropriate stakeholder involvement.
The Trust Deficit in Healthcare AI
Healthcare operates on a foundation of trust between providers and patients. AI systems face a significant trust deficit from both clinicians and patients, creating implementation barriers. Providers may question AI recommendations that contradict their clinical judgment or experience, while patients may feel uncomfortable with AI involvement in their care. This trust issue becomes particularly acute when AI systems lack explainability – the ability to justify their recommendations in understandable terms. According to research published in Nature, healthcare professionals are more likely to reject AI recommendations when the system cannot explain its reasoning. Building trust requires transparent development processes, clear explanation of AI limitations, and evidence of improved outcomes through rigorous clinical validation. Healthcare organizations implementing AI call center solutions must address these trust concerns through careful design and communication strategies.
The Expertise Gap in Healthcare Technology
Successfully implementing AI in healthcare requires specialized expertise that bridges clinical knowledge and technical capabilities. Many healthcare organizations face a significant talent gap in these roles. Data scientists who understand healthcare contexts and clinicians who comprehend AI capabilities are rare combinations. According to a report by Deloitte, 63% of healthcare organizations cite hiring qualified AI talent as a major implementation challenge. This expertise deficit extends beyond initial implementation to ongoing maintenance and optimization, which requires continuous technical support. Healthcare institutions must invest in developing internal capabilities through training programs, strategic hiring, and partnerships with technology providers. Organizations exploring solutions like AI appointments schedulers need staff who understand both scheduling workflows and AI configuration requirements.
Data Quality and Standardization Issues
AI systems are only as good as the data they’re trained on, and healthcare data frequently suffers from quality and standardization problems. Electronic health records contain inconsistent formatting, missing values, and variations in terminology that complicate AI training. Data bias represents a particularly insidious challenge, as historical healthcare data often reflects existing disparities in care delivery across demographic groups. According to research published in the New England Journal of Medicine, these biases can perpetuate and even amplify healthcare inequities. Healthcare organizations must invest in data cleaning, standardization, and bias mitigation strategies before implementing AI systems. Continuous data quality monitoring becomes an ongoing implementation requirement, adding to the resource demands of AI projects. Solutions like conversational AI face additional challenges in understanding the nuances of medical terminology and patient communication patterns.
Cost and Resource Allocation Challenges
Implementing AI in healthcare demands significant financial investments beyond the initial technology purchase. Organizations face costs for infrastructure upgrades, integration services, staff training, and ongoing system maintenance. The total cost of ownership frequently surprises healthcare executives, with McKinsey research suggesting that implementation costs can be 2-3 times higher than the original technology investment. Healthcare institutions must carefully evaluate the business case for AI implementation, identifying clear ROI mechanisms that may include improved efficiency, reduced errors, better patient outcomes, or enhanced revenue capture. Smaller healthcare organizations with limited budgets face particular challenges, often needing to prioritize AI implementations with the most immediate financial returns. Technologies like AI appointment setters can provide tangible ROI through improved scheduling efficiency and reduced administrative overhead.
Ethical Considerations in Medical AI
Ethical questions permeate every aspect of healthcare AI implementation. Medical decisions have profound human consequences, raising concerns about algorithm transparency, accountability for AI-assisted decisions, and potential exacerbation of existing healthcare disparities. Who bears responsibility when an AI system contributes to a medical error? How do organizations ensure equitable AI benefits across diverse patient populations? According to the World Health Organization’s guidance on ethics in AI for health, organizations must address these ethical dimensions throughout the AI lifecycle. Implementation challenges include establishing ethical review processes, developing appropriate governance structures, and creating mechanisms for ongoing ethical oversight. Healthcare institutions must engage diverse stakeholders, including patients and ethicists, in implementation planning. Tools such as AI phone consultants require ethical frameworks governing how patient information is handled and what recommendations these systems can provide.
Change Management and Organizational Culture
Technological implementation always occurs within organizational cultures that can either facilitate or obstruct change. Healthcare institutions often maintain deeply entrenched routines and professional hierarchies that resist technological disruption. According to research in the Harvard Business Review, cultural resistance represents one of the primary reasons healthcare technology implementations fail. Successful AI adoption requires comprehensive change management strategies that address stakeholder concerns, provide adequate training, and create incentives for new behavior adoption. Organizations must identify and empower change champions who help colleagues navigate the transition. Clear communication about why the AI implementation matters and how it benefits providers and patients becomes essential. Solutions like call center voice AI require careful change management as they transform traditional human-centered roles.
Clinical Validation and Evidence Generation
Healthcare’s evidence-based culture demands rigorous validation of new technologies before widespread adoption. AI systems face particularly stringent requirements to demonstrate clinical value and safety. The validation process for healthcare AI differs substantially from other industries, requiring clinical trials, peer-reviewed research, and real-world evidence generation. According to guidance from the FDA, developers must establish not only that their AI performs as intended but also that it provides meaningful clinical benefits. This validation process creates significant implementation delays as organizations must gather evidence specific to their patient populations and use cases. Healthcare institutions frequently struggle with designing appropriate validation studies that balance scientific rigor with practical considerations. Technologies like AI voice assistants require validation across different healthcare scenarios and patient demographics.
Implementation Scalability Challenges
Many healthcare AI implementations begin with promising pilots but fail during wider deployment. Scaling AI solutions across large healthcare systems introduces complexity not present in controlled pilot environments. According to research by MIT Sloan Management Review, fewer than 10% of companies successfully scale their AI pilots. Healthcare organizations face particular challenges with maintaining consistent performance across different facilities, patient populations, and clinical scenarios. Implementation strategies must address variation in local workflows, staff capabilities, and infrastructure. Organizations need robust governance frameworks that standardize certain aspects while allowing appropriate local customization. Creating scalable training programs and support systems becomes essential for organization-wide adoption. Technologies like Twilio AI phone calls require scaling strategies that consider varying call volumes and scenarios across different departments.
Real-Time Decision Support Challenges
Many healthcare AI applications aim to provide real-time decision support during patient care, introducing unique implementation challenges. Clinical decisions often occur under time pressure, requiring AI systems to deliver insights within tight timeframes. According to research in JAMA Network Open, the timing of AI recommendations significantly impacts their adoption by clinicians. Systems must balance comprehensive analysis with practical speed requirements. Implementation challenges include ensuring adequate technical infrastructure for real-time processing, developing appropriate alert mechanisms that don’t contribute to "alert fatigue," and creating fallback procedures when systems are unavailable. Healthcare organizations must carefully consider workflow integration points that maximize utility without disrupting critical clinical processes. Solutions like AI phone agents must operate within acceptable response timeframes while maintaining conversation quality.
Managing Patient and Public Perceptions
Public perception significantly influences healthcare AI implementation success. Patients may express concerns about reduced human interaction, privacy risks, or algorithmic decision-making in their care. Media coverage of healthcare AI often amplifies both potential benefits and risks, shaping public expectations. According to research in the Journal of Medical Internet Research, patient acceptance of healthcare AI varies substantially based on application type and how the technology is framed. Implementation strategies must include thoughtful communication plans that address patient concerns while realistically setting expectations. Organizations should consider involving patient representatives in implementation planning and creating clear explanations of how AI tools are used in care delivery. Transparent policies regarding data usage and human oversight help build public trust. Technologies like AI voice agents for FAQ handling must be presented to patients in ways that emphasize convenience while acknowledging human backup availability.
Balancing Automation and Human Judgment
Finding the right balance between AI automation and human clinical judgment represents a core implementation challenge. Healthcare requires nuanced decision-making that considers factors difficult to capture in algorithms, including psychosocial elements and patient preferences. According to research in the BMJ, the most effective healthcare AI implementations augment rather than replace human capabilities. Organizations must carefully delineate appropriate boundaries for automated decision-making versus human oversight. Implementation strategies should establish clear protocols for when AI recommendations can be automatically implemented versus requiring human review. These boundaries may shift over time as systems demonstrate reliability and clinicians build trust. Healthcare institutions must avoid both over-reliance on imperfect algorithms and underutilization of valuable AI insights. Solutions like AI call centers require thoughtful design of escalation pathways to human agents.
Legal Liability and Responsibility Frameworks
Healthcare AI implementations introduce complex liability questions that existing legal frameworks struggle to address. Who bears responsibility when AI contributes to adverse outcomes – the technology developer, the healthcare institution, or the clinician who used the system? According to analysis in the Journal of Law and the Biosciences, liability uncertainty creates significant implementation barriers as organizations attempt to mitigate unknown risks. Implementation strategies must include developing clear policies regarding AI system use, documentation requirements, and appropriate oversight. Organizations need updated informed consent processes that disclose AI involvement in patient care when appropriate. Risk management departments require new expertise to evaluate AI-specific liability concerns. Technology contracts must clearly delineate vendor versus institutional responsibilities. Solutions like AI sales representatives in healthcare contexts require careful consideration of what recommendations and information these systems can provide.
Maintaining Business Continuity During Implementation
Healthcare operations cannot pause during technology implementation, creating significant continuity challenges. Patient care must continue uninterrupted while new AI systems are deployed, tested, and refined. According to research by Gartner, maintaining operational stability while implementing new technologies ranks among the top concerns for healthcare executives. Implementation strategies must include robust contingency planning, phased rollouts that limit disruption, and adequate system redundancy. Organizations need parallel workflows that allow fallback to pre-AI processes when necessary. Temporary staffing increases may be required during transition periods to maintain service levels. Implementation timelines must account for healthcare’s seasonal variations, avoiding critical periods like flu season for major changes. Technologies like virtual secretary solutions require careful implementation planning to maintain administrative continuity.
Continuous Learning and System Evolution
Unlike traditional healthcare technologies that remain static after implementation, AI systems require continuous learning and adaptation. Machine learning models can degrade over time as clinical practices evolve, patient populations shift, or documentation patterns change. According to research in Nature Medicine, maintaining AI system performance requires ongoing monitoring and periodic retraining. Healthcare organizations must develop capabilities to evaluate AI performance post-implementation, identifying performance drift and triggering appropriate interventions. Implementation planning should include dedicated resources for this continuous monitoring and improvement cycle. Governance structures need clear processes for approving algorithm updates and validating continued safety and effectiveness. Healthcare institutions often underestimate these post-implementation resource requirements, leading to performance degradation over time. Technologies like conversational AI for medical offices require monitoring for conversation quality and continuous improvement of response capabilities.
Measuring Success and Quantifying Benefits
Healthcare AI implementations frequently struggle with defining and measuring success metrics that capture true value. Traditional return-on-investment calculations may not adequately reflect qualitative improvements in care quality, patient experience, or provider satisfaction. According to research by the Healthcare Information and Management Systems Society (HIMSS), organizations often lack appropriate frameworks to evaluate AI implementations. Implementation strategies should establish clear baseline measurements and relevant success metrics before deployment begins. Organizations need to develop capabilities for both quantitative and qualitative benefit assessment, potentially including surveys, observational studies, and operational metrics. These evaluation frameworks must account for initial performance dips during transition periods before measuring long-term benefits. Healthcare institutions should consider broader impact measures beyond financial returns, including staff retention, patient satisfaction, and quality indicators. Solutions like AI phone numbers require appropriate metrics for call handling efficiency, resolution rates, and caller satisfaction.
Transforming Healthcare with AI: The Path Forward
While implementing AI in healthcare presents numerous challenges, the potential benefits for patients, providers, and health systems justify the effort required to overcome these barriers. Success requires collaborative approaches that bring together clinical expertise, technical capabilities, ethical considerations, and patient perspectives. Future healthcare AI implementations will benefit from emerging best practices, growing regulatory clarity, and improved technical standards. Organizations should approach implementation as a transformational journey rather than a simple technology deployment, recognizing that the greatest value comes from reimagining care processes rather than simply automating existing workflows. As healthcare faces growing demands with constrained resources, thoughtful AI implementation offers a pathway to more sustainable, equitable, and effective care delivery. The challenges are significant, but so is the potential to transform healthcare through carefully implemented artificial intelligence solutions.
Advancing Your Healthcare AI Journey with Callin.io
As you navigate the complex landscape of healthcare AI implementation, having the right technology partners becomes essential for success. If you’re looking to enhance patient communication while reducing administrative burden, Callin.io offers specialized solutions designed specifically for healthcare environments. Our AI phone agents can handle appointment scheduling, answer common patient questions, and provide information about services—all while maintaining HIPAA compliance and seamless integration with your existing systems.
The free account on Callin.io provides an intuitive interface to configure your healthcare-specific AI agent, with test calls included and access to the task dashboard for monitoring interactions. For healthcare organizations seeking advanced capabilities like Google Calendar integration and CRM connectivity, subscription plans start at just $30 USD monthly. Take the next step in your healthcare AI journey by exploring Callin.io today and discover how our solutions can address the unique communication challenges in healthcare environments.
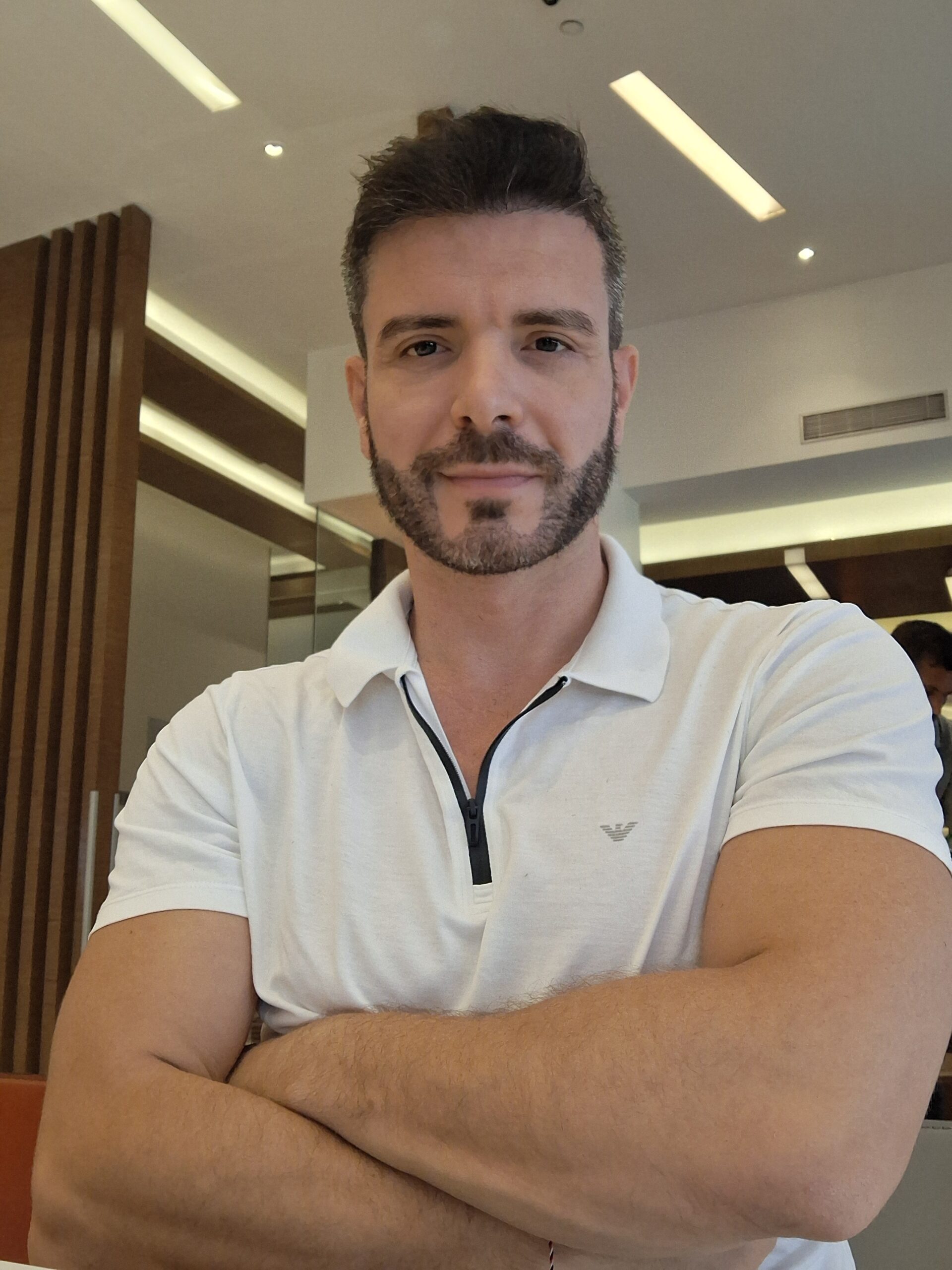
Helping businesses grow faster with AI. 🚀 At Callin.io, we make it easy for companies close more deals, engage customers more effectively, and scale their growth with smart AI voice assistants. Ready to transform your business with AI? 📅 Let’s talk!
Vincenzo Piccolo
Chief Executive Officer and Co Founder