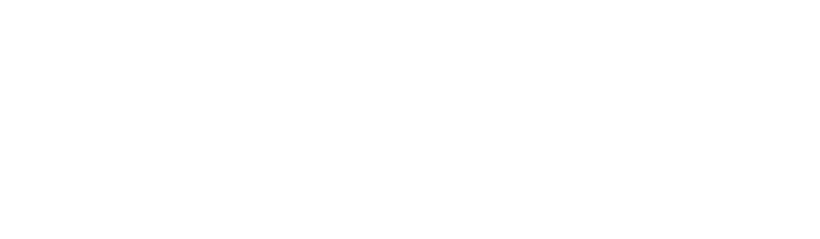
Instant, ephemeral environments & intelligent testing for streamlined software development
Curated list of the best AI Apps and software for any task.
Instant, ephemeral environments & intelligent testing for streamlined software development
Generate endless laughs with your own AI jokester buddy
Custom pickup lines to make memorable first impressions on dating apps
Level up your content game with AI superpowers
Chat with your favorite fictional characters brought to life through conversational AI.
Accurately convert speech into text with our advanced AI transcription technology
Revolutionize content creation with Beebzi.AI's advanced AI technology.
Innovative AI art generator offering unlimited creative possibilities
Revolutionize search and recommendations with Trieve's robust AI infrastructure API.
Unwear.ai: AI-Powered Fashion Image Generation Made Simple
Tavrn: AI-Powered Medical Chronologies for Attorneys
Revolutionize how you interact with AI - monitor, debug, and enhance language models seamlessly.
Callin AI is GDPR compliant. Data security and customer privacy are at the forefront of our business commitments.