The Evolving Landscape of Financial Crime
The banking sector has long been a prime target for financial criminals, with money laundering operations becoming increasingly sophisticated in the digital age. Anti-Money Laundering (AML) compliance has traditionally relied on rule-based systems and manual reviews, creating significant operational burdens for financial institutions worldwide. According to the United Nations Office on Drugs and Crime, an estimated 2-5% of global GDP, or $800 billion to $2 trillion, is laundered annually β a staggering figure that demonstrates the scale of the challenge. The emergence of artificial intelligence technologies represents a paradigm shift in how banks can detect, prevent, and report suspicious financial activities, transforming AML from a cost center into a strategic advantage. As financial institutions navigate complex regulatory requirements and evolving criminal tactics, AI-powered AML solutions are becoming essential components of modern banking infrastructure, similar to how AI voice assistants have revolutionized customer service operations.
Understanding AI’s Role in AML Compliance
Artificial intelligence for AML encompasses a suite of advanced technologies β including machine learning, natural language processing, and deep learning β designed to identify patterns, anomalies, and connections in financial data that would be impossible for human analysts to detect manually. Unlike traditional rule-based systems which operate on predefined thresholds, AI-powered AML solutions can adapt to emerging threats, learn from historical data, and continuously improve their detection capabilities. These systems excel at processing vast quantities of structured and unstructured data, analyzing transaction patterns across multiple dimensions, and generating risk assessments with significantly reduced false positives. Financial institutions implementing AI for AML compliance benefit from enhanced detection accuracy, operational efficiency, and regulatory alignment, creating a more robust defense against increasingly sophisticated money laundering schemes. The technology parallels advances seen in conversational AI systems that can understand context and nuance in communication.
Machine Learning Models for Transaction Monitoring
The cornerstone of AI-powered AML systems is advanced transaction monitoring powered by sophisticated machine learning models. These algorithms analyze millions of transactions in real-time, establishing behavioral baselines for individual customers, account types, and business relationships. By utilizing supervised learning approaches, systems can be trained on known instances of money laundering, while unsupervised learning models excel at identifying unusual patterns without prior examples. The most effective systems employ ensemble approaches, combining multiple algorithms to maximize detection capabilities across different types of financial crime. For example, a major European bank implemented machine learning models that reduced false positives by 60% while increasing true positive detection by 50%, demonstrating the transformative potential of these technologies. This kind of pattern recognition resembles how AI call assistants can detect customer intent from conversation patterns.
Natural Language Processing for KYC Enhancement
Know Your Customer (KYC) processes form the foundation of effective AML programs, and Natural Language Processing (NLP) is revolutionizing how banks collect, verify, and analyze customer information. Advanced NLP algorithms can extract relevant data from identification documents, compare information across multiple sources, and verify consistency across customer interactions. These systems can analyze news articles, social media, and other public sources to develop comprehensive risk profiles, flag potential political exposure, and identify hidden connections between entities. Sentiment analysis capabilities within NLP can even detect suspicious communications or negative news that might indicate increased risk. Leading financial institutions have deployed NLP-enhanced KYC systems that reduce onboarding times by up to 70% while significantly improving risk detection accuracy. The technology shares similarities with AI voice conversation systems that can understand and process natural language.
Network Analysis for Detecting Complex Relationships
Money laundering operations often involve complex networks of transactions, shell companies, and relationships designed to obscure the origin of illicit funds. AI-powered network analysis tools excel at mapping these connections, visualizing transaction flows, and identifying relationship patterns that indicate potential criminal activity. Graph-based analytical models can uncover hidden links between seemingly unrelated accounts, highlight unusual transaction paths, and identify central nodes within suspicious networks. These systems can also incorporate external data sources to enrich their analytical capabilities, creating comprehensive views of customer relationships across multiple institutions. A consortium of global banks implementing network analysis technology recently uncovered a major cross-border laundering operation that had eluded traditional detection methods for years, demonstrating the power of this approach. This networked intelligence mirrors how AI calling agents can connect different pieces of information during a conversation.
Predictive Risk Scoring and Customer Segmentation
AI systems are transforming how banks assess customer risk by enabling dynamic, multidimensional risk scoring that adapts to changing behaviors and market conditions. Machine learning algorithms can analyze hundreds of variables simultaneously to create nuanced risk profiles that go far beyond traditional segmentation approaches. These systems incorporate transactional history, behavioral patterns, relationship networks, and external data points to generate holistic risk assessments. The most sophisticated models employ ensemble learning techniques that combine multiple algorithms to maximize predictive accuracy across different risk dimensions. Financial institutions implementing these technologies report up to 200% improvements in risk prediction accuracy compared to traditional methods, with corresponding reductions in compliance costs and regulatory exposure. This predictive capability shares characteristics with how AI appointment schedulers can anticipate customer needs.
Reducing False Positives Through Behavioral Analytics
One of the most significant challenges in traditional AML systems is the high rate of false positives that consume valuable investigator resources and create alert fatigue. AI-powered behavioral analytics address this issue by establishing comprehensive customer behavioral profiles that account for legitimate variations in transaction patterns. These systems can distinguish between truly suspicious activities and unusual but legitimate transactions by considering contextual factors such as seasonal business fluctuations, life events, and industry-specific transaction patterns. By applying deep learning techniques to historical transaction data, the systems continuously refine their understanding of normal behavior, resulting in more precise anomaly detection. Several tier-one banks have reported 40-60% reductions in false positive alerts after implementing AI-enhanced behavioral analytics, allowing their investigators to focus on genuine risks. This intelligent filtering is similar to how AI voice agents can distinguish between different customer intents.
Automating Suspicious Activity Reports (SARs) Generation
The preparation and filing of Suspicious Activity Reports (SARs) represents a significant operational burden for financial institutions, with each report requiring substantial investigator time. AI technologies are streamlining this process through automated documentation, evidence collection, and narrative generation. Natural language generation systems can transform investigation findings into coherent, regulator-ready narratives that meet filing requirements while maintaining consistency across reports. These systems can automatically incorporate relevant transaction data, relationship information, and historical context, ensuring comprehensive reports that satisfy regulatory expectations. Advanced solutions can even suggest appropriate regulatory classifications based on case characteristics. Banks implementing automated SAR generation have reported 30-50% reductions in preparation time while improving report quality and consistency, freeing investigators to focus on complex analysis rather than documentation. This automation parallels how AI sales representatives can generate detailed conversation summaries.
Real-time Monitoring and Instant Decision Making
Traditional AML systems often operate with significant time lags, analyzing transactions in batches that may delay the detection of suspicious activities. Modern AI-powered solutions enable real-time monitoring capabilities that can identify and flag potential issues as they occur, allowing for immediate intervention when necessary. These systems can evaluate transactions against current risk models at the moment they are initiated, pausing or flagging those that exhibit high-risk characteristics. By incorporating streaming analytics technologies, banks can process millions of transactions simultaneously with minimal latency, ensuring comprehensive coverage without impacting customer experience. Financial institutions implementing real-time monitoring have detected sophisticated fraud attempts that would have evaded traditional batch processing systems, preventing significant financial losses and regulatory issues. This real-time processing capability is similar to how AI phone agents can provide instant responses to customer queries.
Regulatory Technology (RegTech) Integration
The regulatory landscape for AML compliance is constantly evolving, with financial institutions required to adapt to new requirements across multiple jurisdictions. AI-powered RegTech solutions are helping banks manage this complexity through automated regulatory tracking, impact assessment, and implementation planning. These systems can analyze regulatory publications, identify relevant changes, and determine specific impacts on existing processes and systems. Advanced solutions can even suggest policy adjustments and control enhancements to address new requirements, ensuring continuous compliance in a changing environment. By integrating regulatory intelligence directly into operational systems, banks can implement a dynamic compliance approach that evolves alongside regulatory expectations. Financial institutions leveraging RegTech have reported significant reductions in compliance gaps and regulatory findings, demonstrating the value of these technologies. This adaptive regulatory awareness resembles how AI calling bots can follow specific compliance scripts.
Continuous Model Monitoring and Improvement
The effectiveness of AI-powered AML systems depends on their ability to maintain accuracy in the face of evolving criminal tactics and changing financial behaviors. Leading financial institutions implement continuous model monitoring frameworks that evaluate model performance against established metrics, identify potential drift or degradation, and trigger retraining when necessary. These systems incorporate feedback loops that capture investigator decisions and outcomes, using this information to improve future detection capabilities. Advanced approaches employ champion-challenger methodologies where new model versions are tested alongside existing ones to validate improvements before full deployment. By establishing governance frameworks for model risk management, banks can ensure their AI systems remain effective, explainable, and compliant with regulatory expectations. This approach to continuous improvement shares characteristics with how call center voice AI systems learn from each interaction.
Data Quality and Integration Challenges
The effectiveness of AI for AML depends fundamentally on data quality, accessibility, and integration β areas that present significant challenges for many financial institutions. Banks often operate with fragmented data architectures, legacy systems, and inconsistent data standards that complicate the implementation of advanced analytics. Successful AI deployments require comprehensive data strategies that address cleansing, standardization, and integration across multiple sources. Leading institutions have established dedicated data governance frameworks that ensure consistent quality standards, appropriate access controls, and clear ownership responsibilities. Cloud-based data lakes and API-driven architectures have emerged as effective solutions for creating the unified data foundation necessary for advanced AML analytics. Financial institutions that prioritize data quality report significantly higher success rates in their AI implementations, demonstrating the critical importance of this foundational element. These data integration challenges mirror those faced when implementing AI call centers.
Explainability and Transparency Requirements
As financial institutions adopt increasingly sophisticated AI models for AML, they must navigate growing regulatory expectations for model explainability and decision transparency. Regulators worldwide are emphasizing the importance of understand able algorithms that can be validated, explained to non-technical stakeholders, and defended during examinations. Leading banks are addressing these requirements through techniques like SHAP (SHapley Additive exPlanations), LIME (Local Interpretable Model-agnostic Explanations), and attention mechanisms that highlight the specific factors contributing to risk determinations. These explainability approaches allow compliance teams to understand why particular transactions or customers are flagged as suspicious, building confidence in the system’s decisions. Financial institutions that prioritize explainability report more successful regulatory examinations and greater internal adoption of AI technologies. This need for transparency parallels requirements for AI voice assistants for FAQ handling to explain their reasoning.
Human-AI Collaboration Models
The most effective AML programs leverage the complementary strengths of human expertise and artificial intelligence through thoughtfully designed collaboration models. Rather than replacing investigators, AI systems augment their capabilities by handling routine screening, prioritizing alerts based on risk, and providing relevant context for decision-making. Advanced institutions are implementing "human-in-the-loop" frameworks where investigator feedback continuously improves the AI’s detection capabilities. Workflow designs that optimize the division of labor between human and machine components have proven particularly effective, with systems handling initial screening and humans focusing on complex judgment and decision-making. Financial institutions implementing these collaborative approaches report both efficiency gains and improved detection outcomes, demonstrating that the combination of human and artificial intelligence exceeds the capabilities of either component alone. This collaborative approach is similar to how AI phone consultants work alongside human staff.
Security and Privacy Considerations
The sensitive nature of financial data used in AML analytics necessitates robust security and privacy frameworks for AI implementations. Financial institutions must navigate complex requirements including data protection regulations, cross-border data transfer limitations, and customer consent considerations. Leading organizations address these challenges through comprehensive governance frameworks that include privacy-by-design principles, anonymization techniques, and granular access controls. Advanced approaches incorporate differential privacy methods that allow analytics while mathematically guaranteeing individual privacy, and federated learning techniques that enable model training across institutions without sharing raw data. Financial institutions with mature security and privacy frameworks report greater regulatory confidence and customer trust in their AI initiatives, creating competitive advantages in addition to risk reduction. These security considerations parallel those addressed in white label AI receptionist implementations.
Cost-Benefit Analysis of AI for AML
Financial institutions considering AI investments for AML must conduct thorough cost-benefit analyses that account for both quantitative and qualitative factors. The direct costs include technology infrastructure, software licensing, implementation services, ongoing maintenance, and staff training. Benefits typically include reduced false positives, improved detection rates, investigator efficiency gains, and regulatory penalty avoidance. Leading institutions also consider less tangible benefits such as improved risk management capability, enhanced customer experience through reduced friction, and competitive differentiation. When properly implemented, AI for AML delivers compelling returns on investment β with several major banks reporting 200-300% ROI within three years of implementation. However, these returns depend on appropriate scoping, realistic implementation timeframes, and effective change management practices. This financial analysis is similar to evaluations for implementing AI sales call systems.
Implementation Strategies for Financial Institutions
Successful implementation of AI for AML requires thoughtful planning, appropriate scoping, and effective change management across people, processes, and technology. Rather than attempting comprehensive transformation, leading institutions follow **phased implementation
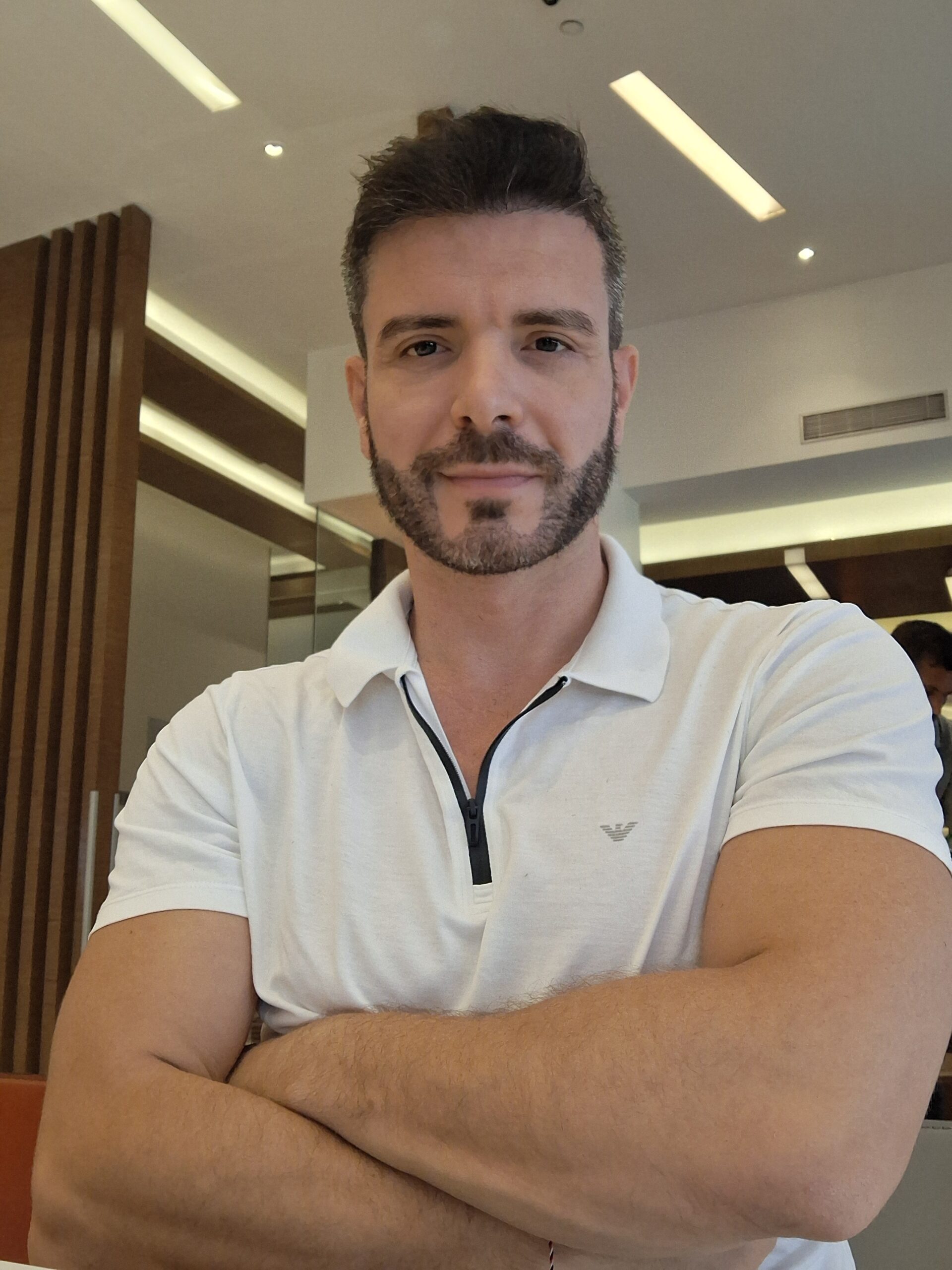
Helping businesses grow faster with AI. π At Callin.io, we make it easy for companies close more deals, engage customers more effectively, and scale their growth with smart AI voice assistants. Ready to transform your business with AI? π Β Letβs talk!
Vincenzo Piccolo
Chief Executive Officer and Co Founder