Understanding the AI Prospecting Landscape
AI has revolutionized how sales teams identify and engage potential customers. However, many organizations rush into implementing AI for sales prospecting without adequate planning or understanding.
The promise of automated lead generation and qualification has created tremendous excitement, but this enthusiasm often leads to costly implementation errors. According to recent studies, over 60% of companies using AI for prospecting report significant challenges during their initial deployment.
These difficulties stem largely from misaligned expectations and poor planning strategies. Rather than viewing AI as a complete replacement for human sales professionals, successful organizations position it as a powerful enhancement tool.
Many sales leaders fail to recognize that AI tools for sales prospecting require thoughtful configuration to match specific business needs. When properly deployed, AI can analyze vast amounts of data to identify patterns that human prospectors might miss, creating opportunities that would otherwise remain hidden.
Relying Too Heavily on Automation
One of the most damaging mistakes sales teams make is over-automating their prospecting process. While artificial intelligence in sales offers tremendous efficiency benefits, treating AI as a complete replacement for human judgment leads to disastrous results.
Sales prospecting fundamentally involves building relationships, and customers can easily detect when they’re interacting with fully automated systems. According to a HubSpot study, 77% of buyers report negative impressions of businesses that rely exclusively on automated outreach.
The key is finding the right balance between automation and personalization. Modern prospecting requires a hybrid approach where AI handles data analysis and initial outreach scheduling, while sales professionals add the crucial human element that builds trust.
Many organizations implement AI for sales without clear boundaries on which tasks should remain human-centered. Effective teams use AI to enhance human capabilities rather than replace them, creating synergies that neither could achieve independently.
Neglecting Data Quality and Integration
AI-powered prospecting tools are only as effective as the data they process. Despite this reality, many organizations implement sophisticated AI bots for sales without addressing fundamental data quality issues in their CRM systems.
Poor data quality manifests in multiple ways: duplicate records, outdated contact information, missing fields, and inconsistent formatting. When AI systems analyze compromised datasets, they inevitably produce flawed recommendations and inaccurate prospect profiles.
Research from MIT Technology Review suggests data quality issues waste up to 50% of AI implementation efforts. Before deploying prospecting AI, companies should invest in comprehensive data cleaning initiatives and establish ongoing data governance procedures.
Beyond quality concerns, many organizations struggle with integration challenges. Effective AI prospecting requires seamless connections between CRM platforms, communication tools, and analytics systems. Without proper integration, sales teams face fractured workflows and incomplete prospect views that significantly reduce AI effectiveness.
Failing to Customize AI for Your Specific Market
Generic AI prospecting approaches rarely deliver optimal results. Many organizations make the critical mistake of implementing AI phone agents without tailoring them to their specific industry, market segment, or buyer personas.
Different industries exhibit unique buying signals and decision-making processes. Financial services buyers, for example, demonstrate significantly different engagement patterns compared to manufacturing or healthcare prospects. AI systems must be calibrated to recognize these industry-specific indicators.
Similarly, market segments within industries show distinct characteristics. Enterprise buyers typically involve multiple stakeholders and longer sales cycles, whereas SMB prospects often have more straightforward buying processes but heightened price sensitivity.
The most effective AI prospecting implementations incorporate detailed buyer persona data, including behavioral patterns, engagement preferences, and common objections. This customization enables AI voice assistants to identify high-probability prospects more accurately and engage them more effectively. Organizations like Hyperbound.ai have demonstrated that market-specific AI customization can increase qualification accuracy by over 40%.
Ignoring Ethical Considerations and Compliance
In the rush to implement AI prospecting, many organizations overlook critical ethical considerations and compliance requirements. This oversight can result in damaged brand reputation, regulatory penalties, and eroded customer trust.
Privacy regulations like GDPR in Europe and CCPA in California impose strict requirements on data collection and usage. AI prospecting must comply with these frameworks by ensuring proper consent management and data handling procedures. According to recent findings from Salesforce, 84% of customers say that the experience a company provides is as important as its products or services.
Beyond regulatory compliance, ethical AI implementation includes considerations like algorithmic bias. Unchecked AI prospecting systems may inadvertently discriminate against certain demographic groups or reinforce existing market inequalities.
Transparency represents another crucial ethical dimension. Prospects deserve clarity about when they’re interacting with AI systems versus human representatives. Many organizations implementing conversational AI fail to properly disclose automated interactions, creating feelings of deception among potential customers when they discover the truth.
Underestimating the Learning Curve
Many sales leaders assume that implementing AI for call centers and prospecting will deliver immediate results. This unrealistic expectation leads to premature disappointment and abandonment of potentially valuable AI investments.
Even the most advanced AI prospecting systems require significant training periods to reach optimal performance. Machine learning algorithms need sufficient data exposure to recognize meaningful patterns and refine their recommendations. During this initial period, prospecting results may actually decline as the system learns.
Organizations must also account for the human learning curve. Sales professionals need time to understand how to effectively collaborate with AI tools, interpret their recommendations, and integrate them into existing workflows. Research from McKinsey indicates that comprehensive training programs can reduce AI implementation timelines by up to 40%.
The most successful AI prospecting implementations incorporate phased rollout strategies with clearly defined performance benchmarks. This approach allows for controlled learning and refinement before scaling across the entire sales organization.
Prioritizing Quantity Over Quality in Prospecting
AI tools can dramatically increase the volume of prospects identified and contacted, but this capability often leads organizations astray. Many sales teams make the mistake of prioritizing quantity metrics over quality engagement when implementing AI for sales prospecting.
This volume-centric approach produces predictable outcomes: decreased response rates, damaged brand reputation, and diminished conversion percentages. Modern buyers are increasingly sensitive to mass-produced outreach efforts, regardless of how they’re delivered.
Effective AI prospecting focuses on identifying fewer, higher-probability prospects and engaging them with relevant, personalized communication. According to Gartner, sales teams that prioritize prospect quality over quantity achieve 50% higher conversion rates and 35% larger average deal sizes.
The best implementations use AI to analyze prospect data more deeply rather than simply processing more prospects. This approach identifies genuine buying signals and engagement opportunities that create meaningful sales conversations rather than unwelcome interruptions.
Neglecting Cross-Functional Collaboration
AI prospecting implementations frequently fail because they operate in departmental isolation. Sales teams often deploy these tools without adequate input from marketing, customer service, IT, and compliance stakeholders.
This siloed approach creates numerous challenges. Marketing teams possess valuable insights about messaging effectiveness and buyer journey mapping that should inform AI prospecting strategies. Without this input, sales AI may deliver inconsistent brand messaging or target prospects at inappropriate journey stages.
Similarly, customer service teams understand common pain points and satisfaction drivers that can enhance prospect qualification models. IT departments provide crucial infrastructure expertise to ensure seamless integration between AI phone calls systems and existing technology stacks.
Research from Deloitte indicates that cross-functional AI implementations are 2.5 times more likely to achieve ROI targets compared to departmentally isolated projects. The most successful organizations establish formal collaboration frameworks that include:
- Joint planning sessions before implementation
- Shared performance metrics across departments
- Regular cross-functional review meetings
- Integrated feedback mechanisms
Misaligning AI Capabilities with Sales Process Stages
Different stages of the sales process benefit from different AI capabilities, but many organizations apply the same AI approach across their entire pipeline. This one-size-fits-all strategy significantly reduces effectiveness.
Early-stage prospecting requires AI systems that excel at pattern recognition across large datasets to identify potential matches. These systems should focus on analyzing firmographic data, digital behavior signals, and market triggers that indicate potential interest.
Middle-stage engagement benefits from AI that can personalize communication based on prospect characteristics and engagement history. Tools like AI cold calls can be effective here when properly implemented with sufficient personalization.
Later-stage qualification requires more sophisticated AI capabilities that can evaluate complex buying signals and predict conversion probability. These systems often incorporate natural language processing to analyze prospect communication patterns and sentiment indicators.
Organizations that strategically align AI capabilities with specific sales process stages report 65% higher ROI from their technology investments, according to Forrester Research. This alignment requires careful mapping of the sales process and thoughtful tool selection rather than implementing a single AI solution across all activities.
Overlooking Human-AI Collaboration Training
Even the best AI prospecting technology fails when sales teams lack proper training on how to effectively collaborate with these systems. Many organizations invest heavily in AI implementation but neglect the crucial human side of the equation.
Sales professionals require specialized training to understand how AI generates recommendations, what data informs these suggestions, and when human judgment should override algorithmic guidance. Without this understanding, teams either blindly follow AI recommendations or ignore them entirely.
Effective human-AI collaboration in prospecting requires new skill development. Sales professionals need to learn how to:
- Interpret AI-generated prospect insights
- Recognize patterns across recommendation sets
- Provide productive feedback to improve system performance
- Determine when to diverge from AI suggestions
Research from Harvard Business Review indicates that organizations investing equally in AI technology and human training achieve 3x better results than those focusing primarily on technology implementation. Companies like Sales-mind.ai have demonstrated successful approaches to this training challenge by developing specialized coaching programs for AI-enhanced prospecting teams.
Setting Unrealistic Performance Expectations
Many organizations implement AI prospecting with dramatically unrealistic performance expectations. Sales leaders often anticipate immediate productivity increases of 50% or more, leading to inevitable disappointment when actual results fall short.
Realistic AI prospecting implementations typically follow a predictable performance curve. Initial results often show modest improvements or even temporary declines during the learning period. As systems accumulate data and sales teams develop collaborative skills, performance gradually improves, eventually reaching significant gains.
According to research from Bain & Company, most successful AI sales implementations achieve 15-30% productivity improvements after full implementation—substantial gains, but far below the transformative outcomes many leaders initially expect.
Organizations should establish realistic performance benchmarks based on industry-specific case studies rather than generalized AI marketing claims. These benchmarks should include both short-term transition metrics and long-term success indicators to maintain momentum through implementation challenges.
Ignoring Customer Experience Impact
In the pursuit of internal efficiency gains, many organizations neglect to consider how AI prospecting affects the customer experience. This oversight can severely damage brand perception and relationship development.
Prospects increasingly recognize automated outreach, and their reactions depend largely on execution quality. Poorly implemented AI calling agents create frustrating experiences that permanently damage brand perception, while thoughtfully designed systems can provide value from the first interaction.
Several specific factors influence customer experience in AI prospecting:
- Disclosure transparency about AI usage
- Personalization depth beyond basic mail merges
- Contextual awareness of previous interactions
- Seamless transitions between AI and human touchpoints
- Consistent brand voice across all communications
Research from PwC indicates that 73% of consumers point to customer experience as a critical factor in purchasing decisions. Organizations must evaluate AI prospecting not only through internal efficiency metrics but also through the prospect’s experience lens.
Failing to Iterate and Improve AI Systems
Many organizations treat AI prospecting implementation as a one-time project rather than an ongoing evolution. Once deployed, these systems often receive minimal updates or optimization, severely limiting their long-term effectiveness.
AI prospecting requires continuous refinement based on performance data, market changes, and emerging capabilities. According to MIT Sloan Management Review, organizations with formalized AI improvement processes achieve 3x better results compared to those treating AI as static implementations.
Several specific areas require ongoing attention:
- Training data refreshes with current market patterns
- Algorithm tuning based on conversion outcomes
- Feature enhancements aligned with sales process changes
- Integration updates as tech stack evolves
- User interface refinements based on sales team feedback
Companies like Fireflies.ai have demonstrated the value of continuous improvement cycles through their regular system updates based on user feedback and performance metrics. This approach ensures AI prospecting systems deliver increasing value rather than degrading performance over time.
Overlooking Integration with Existing Workflows
AI prospecting tools must seamlessly integrate into existing sales workflows to deliver maximum value. Many implementations fail because they require sales professionals to adopt entirely new processes or constantly switch between disparate systems.
Effective integration focuses on embedding AI capabilities within familiar workflows rather than creating parallel processes. This approach minimizes adoption friction and accelerates value realization. According to Accenture, workflow-integrated AI implementations achieve adoption rates 2.5x higher than standalone deployments.
Several integration points prove particularly valuable:
- CRM embedding of AI-generated prospect insights
- Calendar integration for automated meeting scheduling
- Communication platform integration for seamless outreach
- Mobile accessibility for on-the-go sales professionals
- Notification systems for time-sensitive prospect opportunities
Organizations like Bland.ai have demonstrated successful integration approaches by designing their AI prospecting tools to complement existing sales processes rather than disrupt them. This philosophy recognizes that AI should enhance established workflows rather than requiring wholesale transformation.
Not Measuring the Right Performance Indicators
Many organizations evaluate AI prospecting using inappropriate metrics, leading to inaccurate performance assessments and misguided optimization efforts. Traditional volume-based measures like call quantity or email send rates poorly reflect AI prospecting effectiveness.
More meaningful metrics focus on progression efficiency and conversion quality. According to Boston Consulting Group, high-performing AI prospecting implementations track indicators that span the entire funnel rather than focusing exclusively on top-of-funnel activities.
Key metrics for AI prospecting effectiveness include:
- Conversion rate improvements across funnel stages
- Engagement quality indicators like response rates and meeting completions
- Time efficiency measures for prospect qualification
- Revenue influence compared to control groups
- Cost per qualified opportunity
Organizations should establish pre-implementation baselines for these metrics to enable accurate performance comparison. Additionally, AI prospecting assessment should include qualitative feedback from both sales professionals and prospects to capture dimensions beyond numeric indicators.
Enhance Your Prospecting Strategy with Callin.io
After exploring the pitfalls of AI implementation in sales prospecting, it’s clear that the right technology partner makes all the difference in achieving success. If you’re ready to elevate your prospecting efforts while avoiding these common mistakes, Callin.io offers the perfect solution.
Callin.io provides AI-powered phone agents that handle both inbound and outbound calls autonomously, delivering natural conversations that genuinely engage prospects. Unlike many AI systems that create frustrating customer experiences, Callin.io’s technology focuses on meaningful interactions that build relationships rather than just generating volume.
The platform seamlessly integrates with your existing workflows, offering easy connections to popular CRM systems and calendar applications. This integration addresses one of the most significant challenges organizations face when implementing AI prospecting solutions.
You can start with a free account that includes test calls and access to the comprehensive task dashboard. For more advanced features, subscription plans begin at just $30 per month. Experience how thoughtful AI implementation can transform your prospecting without falling into common traps – visit Callin.io today.
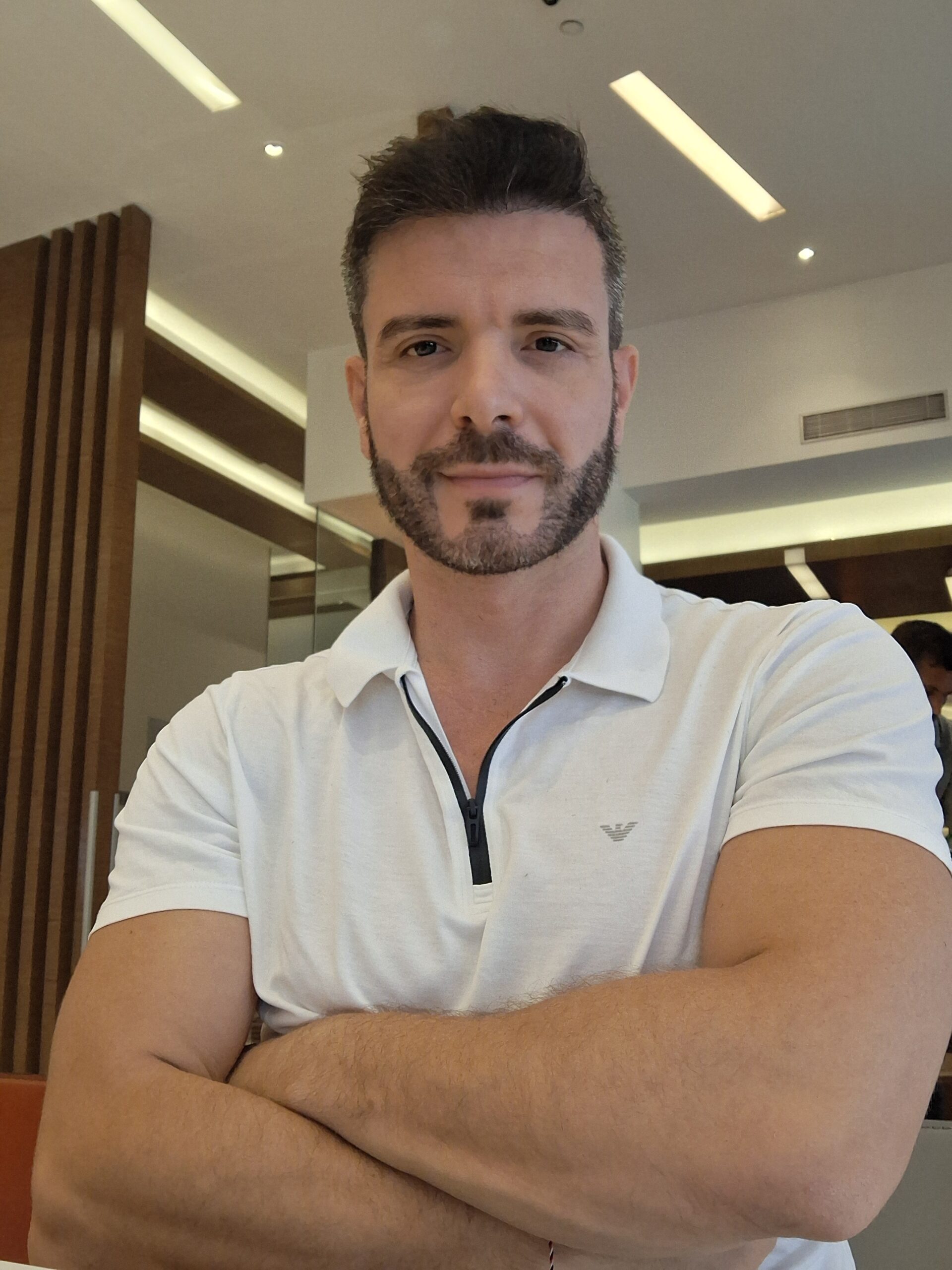
Helping businesses grow faster with AI. 🚀 At Callin.io, we make it easy for companies close more deals, engage customers more effectively, and scale their growth with smart AI voice assistants. Ready to transform your business with AI? 📅 Let’s talk!
Vincenzo Piccolo
Chief Executive Officer and Co Founder