Understanding the SaaS BI Landscape
Business intelligence has undergone a remarkable transformation with the rise of SaaS (Software-as-a-Service) solutions that deliver powerful data analysis capabilities without the traditional infrastructure burdens. These cloud-based platforms have democratized access to advanced analytics, making sophisticated data insights available to organizations of all sizes.
Today’s SaaS BI tools combine user-friendly interfaces with enterprise-grade capabilities, enabling businesses to extract meaningful patterns from their data without requiring specialized technical expertise. The market has matured significantly in recent years, with vendors offering increasingly sophisticated features like natural language querying, automated insights, and embedded analytics.
For companies seeking to become more data-driven, SaaS BI solutions offer a compelling combination of accessibility, scalability, and cost-effectiveness. Unlike traditional on-premises systems that require substantial upfront investment, cloud-based BI tools operate on a subscription model that aligns costs with actual usage and value delivered. This shift mirrors broader trends in AI for sales and other business functions, where technology is becoming more accessible and adaptable.
Key Benefits of SaaS Business Intelligence
The migration to cloud-based business intelligence delivers numerous advantages that explain its growing popularity. First and foremost is accessibility – authorized users can access insights from anywhere with an internet connection, supporting distributed teams and remote work environments. This accessibility extends to mobile devices, enabling decision-makers to stay connected to critical business metrics even while on the move.
Cost considerations also strongly favor SaaS models. Traditional BI implementations often require significant capital expenditure on hardware, software licenses, and specialized IT staff. In contrast, SaaS solutions convert these into predictable operational expenses, with subscription pricing that scales according to your needs. Many providers offer tiered pricing structures that accommodate everything from small businesses to large enterprises.
Implementation speed represents another crucial advantage. While conventional BI projects might take months or even years to fully deploy, cloud-based alternatives can be operational in days or weeks. This rapid time-to-value allows businesses to start benefiting from data insights almost immediately, without lengthy development cycles. Companies exploring how to use AI for sales will find similar benefits in terms of quick implementation and ROI.
Essential Features of Modern BI Platforms
Today’s leading SaaS business intelligence solutions share several fundamental capabilities that define the category. Data connectivity stands at the foundation, with platforms offering pre-built integrations to common business systems like CRM, ERP, marketing automation tools, and databases. The best solutions support both cloud and on-premises data sources, creating a unified analytics environment regardless of where information resides.
Visual analytics capabilities transform raw data into comprehensible insights through interactive dashboards, charts, and graphs. Modern tools emphasize intuitive drag-and-drop interfaces that enable non-technical users to explore data relationships without writing code. These visualization features often incorporate best practices in data presentation automatically, ensuring that insights are communicated effectively.
Self-service capabilities have become increasingly important, allowing business users to create their own reports and analyses without constant IT involvement. This democratization of data access promotes a more pervasive analytics culture throughout organizations. Many platforms now incorporate conversational AI elements that let users query data using natural language rather than technical syntax, further lowering barriers to insight.
Top SaaS BI Tools for 2024
The market for business intelligence software has grown increasingly competitive, with several standout platforms dominating the conversation. Tableau (now part of Salesforce) continues to set standards for visual analytics with its intuitive interface and powerful data exploration capabilities. The platform excels at transforming complex datasets into compelling visualizations that reveal patterns and anomalies at a glance.
Microsoft Power BI leverages its integration with the broader Microsoft ecosystem to deliver seamless connectivity with Excel, Azure, and other Microsoft products. Its combination of accessibility and enterprise-grade features has made it particularly popular in organizations already committed to Microsoft technologies. Power BI’s rapid release cycle ensures continuous improvement with new features arriving monthly.
Looker (acquired by Google Cloud) distinguishes itself with a unique approach centered on its proprietary LookML modeling language. This creates a semantic layer that ensures consistent definitions and metrics across the organization. For companies already using AI for call centers, Looker’s ability to integrate call data with other business metrics can provide valuable context for customer interactions.
Other notable platforms include Domo, with its emphasis on business-user accessibility and mobile capabilities; Sisense, known for handling large, complex data sets; and Qlik Sense, with its associative engine that preserves relationships between all data points. Each offers distinctive approaches to the core challenges of business intelligence.
Specialized BI Solutions for Different Industries
While general-purpose business intelligence platforms offer broad applicability, industry-specific solutions address the unique data challenges of particular sectors. Healthcare analytics platforms like Tableau Healthcare or Qlik Healthcare focus on patient outcomes, operational efficiency, and regulatory compliance, incorporating specialized visualizations for clinical metrics and integration with electronic health records systems.
Retail intelligence solutions prioritize inventory management, customer behavior analysis, and sales performance tracking. They often feature built-in metrics specific to retail operations and capabilities for analyzing omnichannel customer journeys. Companies in this space might benefit from combining these tools with AI voice assistants for FAQ handling to enhance customer service.
Financial services BI platforms emphasize risk analysis, portfolio management, and regulatory reporting. These systems typically incorporate advanced statistical capabilities and time-series analysis tools essential for financial modeling. Similarly, manufacturing-focused solutions center on production efficiency, quality control, and supply chain optimization, often with real-time monitoring capabilities for factory operations.
The advantage of these specialized tools lies in their pre-built functionality addressing industry-specific workflows and KPIs, potentially reducing implementation time and increasing relevance of insights. However, they may sacrifice some flexibility compared to general-purpose platforms.
Data Preparation and Integration Capabilities
Effective business intelligence depends heavily on properly prepared data, making preparation and integration capabilities critical considerations when evaluating SaaS BI tools. Data cleaning and transformation features help address quality issues that could otherwise compromise analysis, with capabilities ranging from simple operations like removing duplicates to complex transformations using expressions and functions.
ETL (Extract, Transform, Load) processes determine how efficiently data moves from source systems into the BI environment. Leading platforms offer both scheduled and real-time data synchronization options to balance performance with data freshness requirements. More advanced solutions provide custom SIP trunking style flexibility in how data connections are established and maintained.
The most comprehensive BI tools incorporate data governance capabilities that ensure information is used consistently and appropriately throughout the organization. This includes features for data lineage tracking, metadata management, and access controls based on roles and responsibilities. Such governance becomes increasingly important as analytics usage expands beyond specialized teams to broader business audiences.
For organizations managing diverse data sources, look for platforms offering pre-built connectors to common business systems alongside options for creating custom integrations when needed. The ability to blend data from multiple sources without extensive coding is particularly valuable for creating comprehensive business views.
Advanced Analytics and AI Capabilities
Modern SaaS BI platforms increasingly incorporate sophisticated analytics and artificial intelligence features that extend their capabilities beyond traditional reporting. Predictive analytics functionality enables forecasting future trends based on historical patterns, with algorithms ranging from simple linear regressions to complex machine learning models that can identify subtle relationships in the data.
Natural language processing (NLP) powers two transformative capabilities: the ability for users to query data conversationally (asking questions like "What were our top-selling products last quarter?") and automated narrative generation that explains insights in plain language rather than requiring interpretation of visualizations. These features significantly lower barriers to analytics adoption across organizations.
Anomaly detection algorithms automatically identify unusual patterns that might indicate problems or opportunities, directing attention to areas requiring investigation. For example, a sudden change in customer behavior or an unexpected shift in operational metrics would trigger alerts. This capability becomes particularly valuable as data volumes grow beyond what human analysts can manually review.
The integration of these AI capabilities with traditional BI creates systems that not only organize and visualize data but actively help interpret it, similar to how AI phone agents transform customer interactions. When evaluating platforms, consider not just current AI features but the vendor’s roadmap and commitment to ongoing AI innovation.
Security and Compliance Considerations
Data security remains a paramount concern for organizations implementing cloud-based business intelligence solutions. Robust encryption protocols should protect data both in transit and at rest, preventing unauthorized access even if underlying systems are compromised. Leading SaaS BI providers employ industry-standard encryption methods like TLS/SSL for transmission and AES-256 for stored data.
Access control mechanisms determine who can view and modify different types of information within the system. Look for platforms offering role-based permissions that can align with your organizational structure and data governance policies. The most sophisticated solutions provide row-level security that filters data dynamically based on user attributes, ensuring individuals see only information appropriate to their role.
Compliance certifications provide external validation of security practices. Depending on your industry, certifications like SOC 2, ISO 27001, HIPAA compliance (for healthcare data), or GDPR readiness (for European data subjects) may be essential requirements. Vendors should transparently share their certification status and provide documentation of their compliance programs.
Regular security audits and penetration testing help identify potential vulnerabilities before they can be exploited. Ask prospective vendors about their testing regimens and how quickly they address discovered issues. For organizations handling particularly sensitive information, virtual call security may require additional considerations beyond standard BI platform protections.
Scalability and Performance Optimization
As organizations grow and analytics usage expands, the ability of BI platforms to scale efficiently becomes increasingly important. User scalability refers to how well the system handles increasing numbers of simultaneous users without degradation in performance. Leading SaaS providers employ multi-tenant architectures with dynamic resource allocation to maintain responsiveness even during peak usage periods.
Data volume scalability addresses the platform’s ability to process growing data sets while maintaining acceptable query performance. Cloud-based solutions typically offer advantages here through distributed processing capabilities and elastic computing resources that can expand as needed. Some platforms employ in-memory processing or columnar storage techniques to further enhance performance with large datasets.
Performance optimization features help administrators fine-tune operations for specific workloads. These might include query caching that stores frequently accessed results, aggregation tables that pre-summarize data for common views, and intelligent query routing that directs requests to appropriate resources based on complexity. The best systems make these optimizations largely transparent to end users while still allowing technical staff to make adjustments when necessary.
For organizations anticipating substantial growth, evaluation should include not just current performance but how well the platform accommodates increasing demands without requiring fundamental architectural changes or migrations. This forward-looking assessment can prevent painful transitions later as usage expands.
Mobile and Embedded BI Capabilities
Access to business intelligence on mobile devices has evolved from a luxury to a necessity as decision-makers increasingly need insights while away from their desks. Native mobile applications provide optimized experiences for smartphones and tablets, with touch-friendly interfaces and layouts adapted for smaller screens. The most effective mobile BI solutions maintain consistent security controls across devices while leveraging mobile-specific capabilities like geolocation.
Responsive design approaches ensure that dashboards and reports automatically adjust to different screen sizes without requiring separate development for each device type. This adaptability becomes increasingly important in environments where users access analytics from multiple device types throughout their workday. Leading platforms design visualizations that remain effective even on smaller displays, avoiding information overload.
Embedded analytics functionality allows BI components to be integrated directly into other business applications, bringing insights directly into operational workflows. This capability creates contextual intelligence that helps users make informed decisions without switching between multiple systems. For example, embedding relevant metrics within a CRM system provides sales representatives with performance data alongside customer information, similar to how AI call center solutions bring intelligence directly into customer interactions.
The most advanced platforms support offline capabilities that allow continued access to critical insights even during temporary connectivity disruptions, with automatic synchronization once a connection is restored. This feature is particularly valuable for field personnel operating in areas with unreliable network coverage.
Cost Structures and ROI Considerations
Understanding the true cost of SaaS business intelligence involves examining several different expense categories. Subscription pricing models typically align costs with usage through tiered structures based on factors like number of users, data volume, processing requirements, or specific feature sets. While this approach provides flexibility, it requires careful forecasting to prevent unexpected cost increases as usage expands.
Implementation costs extend beyond software licensing to include expenses for data integration, custom development, training, and change management. Though generally lower than traditional on-premises deployments, these costs still represent a significant investment and should be fully accounted for when calculating total cost of ownership.
Measuring return on investment should consider both tangible and intangible benefits. Direct financial impacts might include revenue growth through improved targeting, cost reductions from operational efficiencies, or reduced expenses from eliminating legacy systems. Harder-to-quantify benefits often include faster decision-making, improved collaboration, and greater organizational agility.
To maximize ROI, organizations should establish clear metrics for success before implementation and track these consistently over time. This disciplined approach helps justify continued investment and identifies areas where adjustments might increase value. For specialized uses like AI appointment booking, ROI frameworks should include metrics specific to those business processes.
User Adoption and Training Strategies
Even the most powerful business intelligence tools deliver value only when actively used throughout the organization. Effective onboarding processes create positive first experiences that encourage continued engagement. The best approaches combine standardized training with personalized guidance based on specific user roles and responsibilities within the analytics ecosystem.
Training resources should accommodate different learning styles and technical comfort levels. Interactive tutorials, video demonstrations, searchable documentation, and live support channels all play important roles in comprehensive training programs. Vendors offering robust learning paths with certification options often see higher adoption rates among their customers.
Champions and governance committees help institutionalize analytics usage by providing internal advocacy, establishing best practices, and creating accountability for data-driven decision making. These organizational structures bridge the gap between technical capabilities and business application, ensuring that analytics investments translate into actual behavior changes.
Measuring adoption through usage analytics provides visibility into which features and dashboards receive the most attention, which users are most engaged, and where additional training or refinement might be needed. This feedback loop allows continuous improvement of both the technical implementation and the surrounding processes, similar to how customer service analytics drives ongoing enhancement of support operations.
Integration with Other Business Systems
The value of business intelligence increases significantly when it connects seamlessly with other enterprise applications. CRM integration enables analysis of customer interactions, sales performance, and pipeline metrics alongside other business data. This consolidated view helps organizations understand the complete customer journey and its relationship to overall business outcomes.
ERP connections bring financial and operational data into the analytics environment, supporting comprehensive performance management and resource optimization. The ability to correlate financial results with operational metrics creates powerful insights into profitability drivers and potential efficiency improvements. Similarly, integration with marketing automation platforms enables analysis of campaign performance in the context of broader business impacts.
API availability determines how easily the BI platform can exchange data with both current and future business systems. Well-documented, standards-compliant APIs facilitate custom integrations when pre-built connectors aren’t available. The most flexible platforms offer both REST and GraphQL APIs, webhook support for event-driven interactions, and developer tools that simplify integration work.
For organizations with complex system landscapes, look for BI tools that support metadata-driven integration approaches. These create consistent definitions across different data sources, ensuring that metrics maintain their meaning regardless of which system originated the information. This semantic consistency becomes increasingly important as analytics usage expands throughout the organization.
Collaboration and Knowledge Sharing Features
Modern business intelligence platforms recognize that insights gain value when shared and discussed across teams. Collaborative annotation capabilities allow users to add comments, questions, and context directly to visualizations and reports. These annotations create documented conversations around data points, helping teams reach consensus on interpretations and next actions.
Sharing controls balance accessibility with security by allowing content creators to specify exactly who can view, edit, or redistribute different analytics assets. Granular permissions ensure sensitive information remains protected while still enabling broad distribution of appropriate insights. The most sophisticated platforms integrate with enterprise identity management systems to maintain consistent access controls.
Automated distribution mechanisms deliver relevant insights to stakeholders through scheduled emails, embedded content in business applications, or integration with collaboration platforms like Microsoft Teams or Slack. These proactive delivery approaches ensure critical information reaches decision-makers without requiring them to seek it out, similar to how AI voice receptionists proactively manage incoming communications.
Knowledge repositories capture organizational learning by preserving successful analyses, documentation of data sources, and best practices for different types of business questions. These centralized resources accelerate onboarding of new users and promote consistency in analytical approaches across the organization, creating institutional memory that persists despite personnel changes.
Evaluating Vendor Support and Community Resources
The long-term success of a BI implementation depends significantly on the support ecosystem surrounding the technology. Technical support quality varies substantially between vendors, with differences in availability (hours of coverage, response time guarantees), access methods (phone, email, chat), and support staff expertise. Premium support tiers often provide dedicated account representatives who develop familiarity with your specific implementation.
Implementation services help organizations navigate the complex process of deploying new analytics capabilities. These services range from basic setup assistance to comprehensive consulting engagements covering data strategy, governance frameworks, and change management. Vendors with strong professional services teams can accelerate time-to-value, particularly for organizations with limited internal BI expertise.
User communities provide peer-to-peer knowledge exchange through forums, local user groups, and annual conferences. Active communities generate valuable resources like sample dashboards, custom visualizations, and integration examples that extend the platform’s core capabilities. The vibrancy of these communities often indicates the overall health of the product ecosystem.
Training programs should evolve beyond initial onboarding to support ongoing skill development as both the platform and user needs mature. Leading vendors offer structured learning paths with progressive certification levels that recognize developing expertise, similar to specialization programs for AI sales techniques or other technical domains.
Future Trends in SaaS Business Intelligence
The business intelligence landscape continues to evolve rapidly, with several emerging trends shaping future development. Augmented analytics represents perhaps the most transformative direction, with AI capabilities increasingly automating insight discovery and explanation. These systems proactively identify significant patterns, anomalies, and correlations, then present them in accessible formats with natural language explanations of their importance.
Decision intelligence frameworks extend traditional BI by incorporating prescriptive elements that recommend specific actions based on analytical findings. By connecting insights directly to operational decisions, these approaches close the loop between analysis and action, increasing the practical impact of analytics investments. This evolution mirrors developments in other AI domains like virtual secretaries that not only provide information but assist in acting upon it.
Low-code/no-code development capabilities are democratizing the creation of analytics applications beyond traditional developer roles. Drag-and-drop interfaces for creating both visualizations and underlying data models allow business analysts and other non-technical users to build sophisticated analytical tools tailored to specific needs without extensive programming knowledge.
Real-time analytics processing continues advancing to support increasingly time-sensitive decision contexts. The ability to analyze data streams as events occur enables immediate responses to changing conditions in areas like operations monitoring, security, and customer experience management. Organizations preparing for these trends should consider platforms with clear roadmaps addressing these emerging capabilities.
Maximizing Your BI Investment with Callin.io
Implementing effective business intelligence represents just one element of a comprehensive data strategy. To truly transform operations, organizations must connect insights to action across customer touchpoints, including telephone communications. This is where Callin.io offers remarkable synergy with your BI initiatives.
Callin.io’s AI-powered phone agents can operationalize the insights generated through your business intelligence platform by automatically adapting customer interactions based on data-driven understanding of preferences and behaviors. For instance, when your BI tools identify high-value customer segments or product affinities, Callin.io agents can immediately incorporate this knowledge into their conversations, creating a seamless connection between analysis and customer experience.
The platform’s ability to handle both inbound and outbound calls autonomously means your team can focus on acting upon the strategic insights revealed through business intelligence rather than managing routine communications. The natural-sounding AI interactions maintain quality customer experiences while dramatically increasing efficiency and consistency in telephone operations.
If you’re looking to implement a truly data-driven approach across all customer touchpoints, explore Callin.io today. With a free account option that includes test calls and a comprehensive dashboard for monitoring interactions, you can quickly evaluate how AI-powered communications complement your business intelligence strategy. For organizations ready to scale, subscription plans starting at $30 per month provide advanced features including CRM integration and Google Calendar synchronization, creating a unified system that turns business intelligence into tangible customer value.
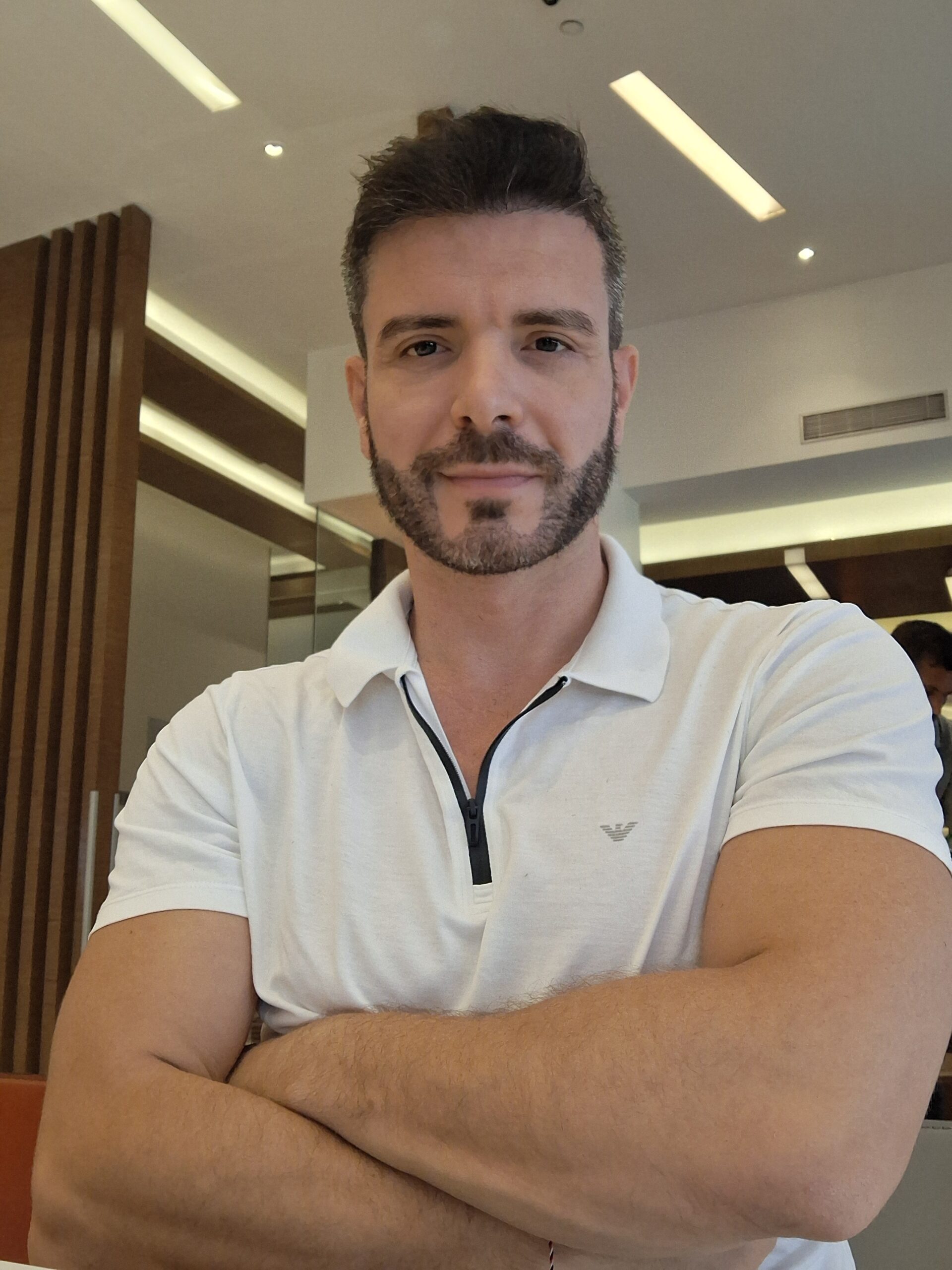
Helping businesses grow faster with AI. π At Callin.io, we make it easy for companies close more deals, engage customers more effectively, and scale their growth with smart AI voice assistants. Ready to transform your business with AI? π Β Letβs talk!
Vincenzo Piccolo
Chief Executive Officer and Co Founder