Understanding Chatbot Fundamentals
When diving into the world of automated conversational solutions, businesses typically face a critical fork in the road: should they implement rule-based chatbots or invest in AI-powered chatbot systems? This decision isn’t merely technical—it directly impacts customer experience, operational efficiency, and business outcomes. Rule-based chatbots function through predefined pathways and scripted responses, making them straightforward but limited in handling unexpected queries. In contrast, AI chatbots leverage natural language processing (NLP) and machine learning to understand context, learn from interactions, and provide more dynamic responses. According to a Stanford University study on conversational AI, organizations that have implemented advanced conversational solutions report up to 30% reduction in customer service costs while simultaneously improving satisfaction rates. Understanding these fundamental differences forms the essential first step in determining which solution aligns with your specific business requirements and customer interaction goals.
The Mechanics of Rule-Based Chatbots
Rule-based chatbots operate on a simple yet effective principle: if the user says X, the bot responds with Y. These systems rely on decision trees and predefined patterns, essentially following a flowchart of conversation possibilities crafted by developers. The structured nature of rule-based chatbots makes them particularly effective for handling routine inquiries with predictable paths, such as providing business hours, processing simple orders, or guiding users through standardized procedures. Their operation involves keyword recognition and exact pattern matching rather than true language understanding. While this makes them incredibly reliable for specific, anticipated queries, they stumble when confronted with questions phrased in unexpected ways or queries outside their programmed knowledge base. As highlighted in Callin.io’s guide on conversational AI, rule-based systems excel in controlled environments where conversation paths can be accurately anticipated and mapped out in advance.
How AI Chatbots Transform Conversations
AI chatbots represent a quantum leap in conversational technology, employing sophisticated algorithms and natural language understanding to interpret user intent rather than merely matching keywords. These systems utilize machine learning models trained on vast datasets of human conversations, enabling them to recognize context, remember previous exchanges, and even detect sentiment. Unlike their rule-based counterparts, AI chatbots can handle complex, multi-turn conversations and adapt their responses based on nuanced understanding of user queries. They’re constantly learning from interactions, becoming progressively more adept at addressing diverse inquiries and understanding various phrasings of the same question. The AI voice assistant technologies developed for modern business applications demonstrate this evolution, offering increasingly natural-sounding interactions that can understand colloquialisms, idioms, and even detect emotional undertones in customer communications—capabilities far beyond the reach of traditional rule-based systems.
Cost and Implementation Considerations
The financial aspect of choosing between rule-based and AI chatbots demands careful consideration. Rule-based systems typically require lower initial investment, with straightforward development costs and minimal ongoing maintenance for stable use cases. They’re essentially "set and forget" solutions until your business requirements change. AI chatbots, while offering superior capabilities, generally demand greater upfront investment in technology, data preparation, and integration. They also require ongoing training and optimization to maintain performance. However, as detailed in Callin.io’s guide to starting an AI calling agency, the return on investment calculation must consider the total cost of ownership alongside potential benefits. While Rule-based systems may seem economically attractive initially, their limited scalability often leads to higher long-term costs if business needs expand or customer expectations evolve, potentially necessitating complete redesigns rather than incremental improvements.
Response Accuracy and Handling Exceptions
The accuracy with which a chatbot responds to user inquiries significantly impacts customer satisfaction and operational efficiency. Rule-based chatbots offer high precision for queries they’re specifically programmed to handle, virtually eliminating errors for anticipated questions. However, they typically respond with generic fallback messages ("I don’t understand") when confronting unanticipated inquiries, creating potential customer frustration. AI chatbots, leveraging sophisticated conversational AI frameworks, demonstrate remarkable versatility in handling exceptions and unexpected queries. Their ability to extract meaning from imperfect inputs allows them to provide relevant responses even when questions are phrased unusually or contain typos. Advanced AI systems can also acknowledge their limitations transparently, offering to escalate to human agents when necessary. According to research published by MIT Technology Review, AI-powered systems can reduce customer frustration by up to 40% compared to rule-based systems when handling edge cases and unexpected queries.
Scalability and Language Support
As businesses expand globally, the scalability of conversational solutions becomes increasingly crucial. Rule-based chatbots face significant scalability challenges, particularly regarding language support and conversation complexity. Adding new languages to rule-based systems typically requires building separate decision trees for each language, essentially creating multiple independent bots. Similarly, expanding the scope of conversations demands manual creation of new rules and pathways. In contrast, AI chatbots demonstrate remarkable scalability advantages, as explored in Callin.io’s analysis of AI for call centers. Modern AI systems can leverage transfer learning techniques to expand language capabilities more efficiently, often requiring only a fraction of the training data needed for the primary language. Furthermore, AI systems can scale conversation complexity organically through additional training rather than complete redesigns, allowing them to grow alongside business needs without proportional increases in development resources.
Personalization Capabilities
The ability to deliver personalized customer experiences has become a competitive differentiator in today’s business landscape. Rule-based chatbots offer limited personalization options, typically restricted to incorporating basic customer information like names or account details into otherwise standardized responses. They generally treat each conversation as an isolated event, unable to adapt their approach based on customer history or preferences. AI chatbots, however, excel at creating tailored experiences by analyzing conversation history, purchase patterns, and even browsing behavior to customize interactions. As demonstrated in Callin.io’s exploration of AI sales applications, advanced AI systems can adjust their communication style to match customer preferences, recommend products based on past behavior, and even modulate their tone based on detected customer sentiment. This customer-specific adaptation creates more engaging, relevant interactions that significantly enhance conversion rates and customer satisfaction levels.
Integration Capabilities with Business Systems
The effectiveness of a chatbot solution often depends on its ability to connect with existing business systems. Rule-based chatbots typically offer straightforward integration with simple systems through basic APIs, allowing them to retrieve information from databases or pass collected data to CRM systems. However, they generally require predefined integration points and struggle with dynamic, complex system interactions. AI chatbots provide significantly more robust integration possibilities, as explored in Callin.io’s guide to AI phone services. These advanced systems can interface with multiple business platforms simultaneously—from inventory management to scheduling systems to payment processors—creating cohesive customer experiences across touchpoints. Additionally, AI solutions can often perform complex operations like analyzing data across systems, identifying patterns, and making recommendations based on comprehensive business intelligence, rather than merely passing information between isolated platforms.
Development and Maintenance Requirements
The ongoing resource commitment required for chatbot solutions varies significantly between rule-based and AI approaches. Rule-based chatbots typically demand extensive upfront planning to map all possible conversation scenarios, create response scripts, and design decision trees. While initial development might be straightforward, maintenance becomes increasingly complex as the system grows, with each new feature potentially requiring adjustments throughout the conversation flow to maintain coherence. AI chatbots generally involve different resource allocation, with significant initial investment in data preparation, model training, and testing. However, as highlighted in Callin.io’s guide to prompt engineering for AI callers, their maintenance often becomes more efficient over time. Rather than requiring rewrites of conversation logic, improvements typically come through additional training data and refinement of underlying models, allowing the system to evolve organically without complete overhauls.
Customer Experience and Satisfaction Impacts
The ultimate measure of any conversational solution lies in its impact on customer experience. Rule-based chatbots offer consistent, predictable interactions that perform reliably within their designed parameters. Their scripted nature ensures quality control for covered topics, providing uniformly accurate information for standard questions. However, their rigidity and limited conversational abilities can create frustrating experiences when customer inquiries deviate from anticipated patterns. AI chatbots, conversely, provide more natural, flexible interactions that better accommodate the unpredictable nature of human communication. According to case studies featured on Callin.io’s customer service solutions page, businesses implementing advanced AI conversational systems report not only higher resolution rates but also improved customer sentiment scores. The ability of AI systems to handle diverse phrasings, remember context across conversation turns, and provide nuanced responses creates interactions that feel more human and less mechanical, significantly enhancing overall customer experience.
Industry-Specific Considerations
Different sectors face unique challenges that influence the choice between chatbot technologies. In the healthcare industry, rule-based chatbots offer the predictability and compliance certainty essential for handling sensitive medical information, but they struggle with the complexity of symptom description and triage. Callin.io’s analysis of conversational AI for medical offices demonstrates how AI solutions can provide the nuanced understanding needed for initial patient screening while maintaining necessary compliance guardrails. In financial services, rule-based systems excel at handling standardized transactions but falter when addressing complex financial planning queries that require contextual understanding. E-commerce businesses often find that rule-based chatbots efficiently handle order status inquiries but need AI capabilities to provide personalized product recommendations. Each industry must weigh regulatory requirements, conversation complexity, and customer expectations when selecting the appropriate chatbot technology for their specific operational context.
Measuring ROI and Performance Metrics
Establishing meaningful performance benchmarks is essential for evaluating chatbot effectiveness. For rule-based chatbots, key metrics typically focus on operational efficiency measures such as conversation completion rates, reduction in human agent workload, and accurate routing to appropriate information resources. Since these systems have clearly defined capabilities, measuring their success centers on how efficiently they handle their programmed scenarios. For AI chatbots, performance evaluation becomes more nuanced, encompassing not only efficiency metrics but also learning and adaptation indicators. As detailed in Callin.io’s guide to AI call centers, meaningful AI performance metrics include continuous improvement rates, context retention accuracy, sentiment analysis precision, and human-like conversation scores. Both approaches require careful consideration of customer satisfaction metrics like Net Promoter Score and post-interaction surveys to ensure technological performance translates into genuine business benefits and positive customer experiences.
Hybrid Approaches: Combining Rule-Based and AI Elements
Many forward-thinking businesses are discovering that the most effective conversational solutions combine elements from both rule-based and AI methodologies. These hybrid chatbot systems leverage the predictability and reliability of rule-based approaches for routine, structured interactions while employing AI capabilities for handling complex, nuanced conversations requiring deeper understanding. For instance, a customer service chatbot might use rules-based decision trees for collecting basic information and categorizing inquiries, then transition to AI-powered conversation when addressing specific product questions requiring contextual understanding. Callin.io’s implementation of AI call assistants demonstrates this hybrid approach, where structured conversation flows are enhanced with AI-powered comprehension for handling unexpected questions and delivering personalized responses. This combined methodology often provides the ideal balance of development efficiency, operational reliability, and conversation quality, mitigating the weaknesses of each approach while capitalizing on their respective strengths.
Future-Proofing Your Chatbot Strategy
As conversational AI technology rapidly evolves, businesses must consider the long-term viability of their chatbot investments. Rule-based systems offer stability and predictability but risk becoming outdated as customer expectations for natural conversation increase. Their rigid architecture typically requires complete rebuilds rather than incremental improvements when fundamental changes are needed. AI chatbots, while requiring ongoing attention to remain current, generally offer better future-proofing through their ability to evolve with additional training and model updates. As explored in Callin.io’s analysis of AI voice conversation technologies, organizations should consider not only current requirements but also anticipated future needs when selecting a chatbot approach. This forward-looking perspective might include evaluating platforms that support smooth transitions from rule-based to AI capabilities, ensuring integration with emerging channels, and considering how conversational data collected today might power improved AI performance tomorrow.
Real-World Success Stories and Case Studies
Examining successful implementations provides valuable insights into the practical applications of different chatbot approaches. In the retail sector, major brands like Sephora have demonstrated how rule-based chatbots can effectively streamline product discovery and purchasing processes through clearly defined interaction paths. Conversely, healthcare providers implementing AI chatbots for patient screening have reported significant improvements in appointment allocation efficiency and patient satisfaction. Callin.io’s collection of AI appointment scheduling case studies highlights how financial services firms have successfully deployed hybrid solutions—using rule-based frameworks for regulatory-sensitive transactions while leveraging AI capabilities for financial advisory services. These real-world examples consistently demonstrate that success depends not on choosing the theoretically superior technology but on selecting the approach that best aligns with specific business objectives, customer expectations, and operational constraints. Organizations achieving the greatest ROI typically match chatbot capabilities precisely to their unique use cases rather than pursuing technological sophistication for its own sake.
Implementation Timeline and Resource Planning
The journey from concept to fully operational chatbot varies significantly between rule-based and AI approaches. Rule-based implementations typically follow a linear development path with clearly defined stages: conversation mapping, script writing, decision tree creation, testing, and deployment. Organizations can often launch basic rule-based solutions in weeks or a few months, depending on complexity. AI chatbot development generally follows a more iterative process involving data collection, model training, testing, refinement, and continuous improvement. As detailed in Callin.io’s guide to creating an AI call center, successful AI implementations require not only technical resources but also cross-functional teams including subject matter experts who can provide domain knowledge for training. While rule-based projects often involve higher initial certainty around timelines and resource requirements, AI projects typically demand more flexible planning approaches that accommodate discovery and iterative improvement phases before reaching optimal performance.
Security and Compliance Considerations
As chatbots increasingly handle sensitive customer information, security and compliance requirements become critical evaluation factors. Rule-based chatbots offer predictable security profiles with clearly defined data handling pathways, making them easier to audit for compliance with regulations like GDPR, HIPAA, or financial services requirements. Their limited functionality can actually become an advantage in highly regulated industries, as they’re less likely to inadvertently process or store sensitive information in unanticipated ways. AI chatbots present more complex compliance challenges due to their learning capabilities and more sophisticated data processing. However, as explored in Callin.io’s white label AI receptionist solutions, advanced AI systems increasingly incorporate robust security features like end-to-end encryption, secure data handling protocols, and compliance-specific training guardrails. Organizations must carefully evaluate both approaches against their specific regulatory requirements, implementing appropriate safeguards such as data minimization, explicit consent mechanisms, and regular security audits regardless of the chosen technology.
Human Oversight and Escalation Protocols
Even the most sophisticated chatbot systems benefit from thoughtful integration with human support processes. Rule-based chatbots typically incorporate straightforward escalation paths based on specific triggers like keyword detection or conversation dead-ends. Their predictable operation makes it relatively easy to identify situations requiring human intervention. However, their limited self-awareness means they might continue attempted resolutions even when failing to provide value. AI chatbots generally offer more nuanced escalation capabilities, including sentiment-based triggers that can detect customer frustration, complexity assessment algorithms that recognize when conversations exceed their capabilities, and intelligent routing to appropriate human specialists based on conversation context. As highlighted in Callin.io’s exploration of call center voice AI, the most effective implementations blend automated and human elements seamlessly, creating "human-in-the-loop" systems where AI handles routine matters while facilitating smooth transitions to human agents for complex or sensitive situations requiring judgment, empathy, or creative problem-solving.
Training Requirements for AI Chatbots
The performance of AI chatbots depends significantly on the quality and quantity of their training data. Effective AI chatbot implementation requires a comprehensive data strategy addressing both initial training and ongoing optimization. Organizations must typically assemble diverse conversational datasets representing the full spectrum of customer inquiries, including variations in phrasing, intent, and complexity. As explored in Callin.io’s guide to creating custom LLMs, successful training approaches often combine public conversation datasets with industry-specific examples and company-unique interactions. Beyond initial development, organizations must establish processes for continuous improvement through feedback loops, performance monitoring, and periodic retraining with new data. This ongoing commitment represents a significant difference from rule-based systems, which require explicit programming changes rather than learning from experience. However, the investment in proper AI training typically yields substantial returns through the system’s ability to handle increasingly diverse queries and adapt to changing customer behaviors without requiring complete redesigns.
Decision Framework for Choosing the Right Solution
Selecting between rule-based and AI chatbots requires a structured evaluation process aligned with business objectives. Organizations should begin by conducting a thorough needs assessment examining conversation complexity, query variability, personalization requirements, and integration needs. For businesses primarily handling standardized inquiries with predictable paths—such as appointment scheduling, basic order processing, or FAQ responses—rule-based chatbots often provide sufficient capability with lower implementation complexity. Conversely, organizations managing diverse, complex customer interactions requiring contextual understanding and personalization typically benefit from AI-powered solutions despite higher initial investment. Callin.io’s guide to AI phone agents recommends evaluating both current requirements and anticipated future needs, particularly considering how customer expectations around conversational experiences are rapidly evolving. Many organizations find that starting with a well-designed rule-based solution for core functions while implementing AI capabilities for complex scenarios offers an effective staged approach, allowing operational benefits while building expertise for more advanced implementations.
Embracing the Future of Conversational Technology
The evolution of conversational AI continues at a remarkable pace, transforming how businesses engage with customers across channels and touchpoints. Today’s decision between rule-based and AI chatbots represents just one step in an ongoing technological journey. Forward-thinking organizations are already exploring emerging capabilities like multimodal AI that combines textual, visual, and audio understanding; emotion recognition systems that adapt conversational tone to customer sentiment; and increasingly sophisticated personalization engines that tailor interactions based on comprehensive customer profiles. As these technologies mature, the distinction between rule-based and AI systems may eventually blur into a spectrum of conversational solutions optimized for specific business contexts. Whatever approach you select today, maintaining flexibility and adaptability in your conversational strategy will be essential for long-term success in an increasingly AI-driven business landscape.
Taking Your Customer Communications to the Next Level
Ready to transform how your business handles customer conversations? Whether you’re considering a rule-based system for specific structured interactions or exploring comprehensive AI solutions for more natural customer engagement, implementing the right conversational technology can dramatically improve operational efficiency and customer satisfaction. If you’re looking to implement state-of-the-art conversational solutions with minimal technical complexity, Callin.io offers an ideal platform for deploying AI-powered phone agents capable of handling both inbound and outbound customer communications autonomously. With Callin.io’s intelligent AI phone agents, you can automate appointment scheduling, answer common questions, and even close sales through natural-sounding conversations that deliver consistent results.
Callin.io provides a user-friendly interface for configuring your AI agent, with free trial calls and comprehensive interaction monitoring through the task dashboard. For businesses requiring advanced capabilities like Google Calendar integration and built-in CRM functionality, subscription plans start at just $30 per month. Discover how Callin.io can help your business leverage the power of conversational AI by visiting Callin.io today.
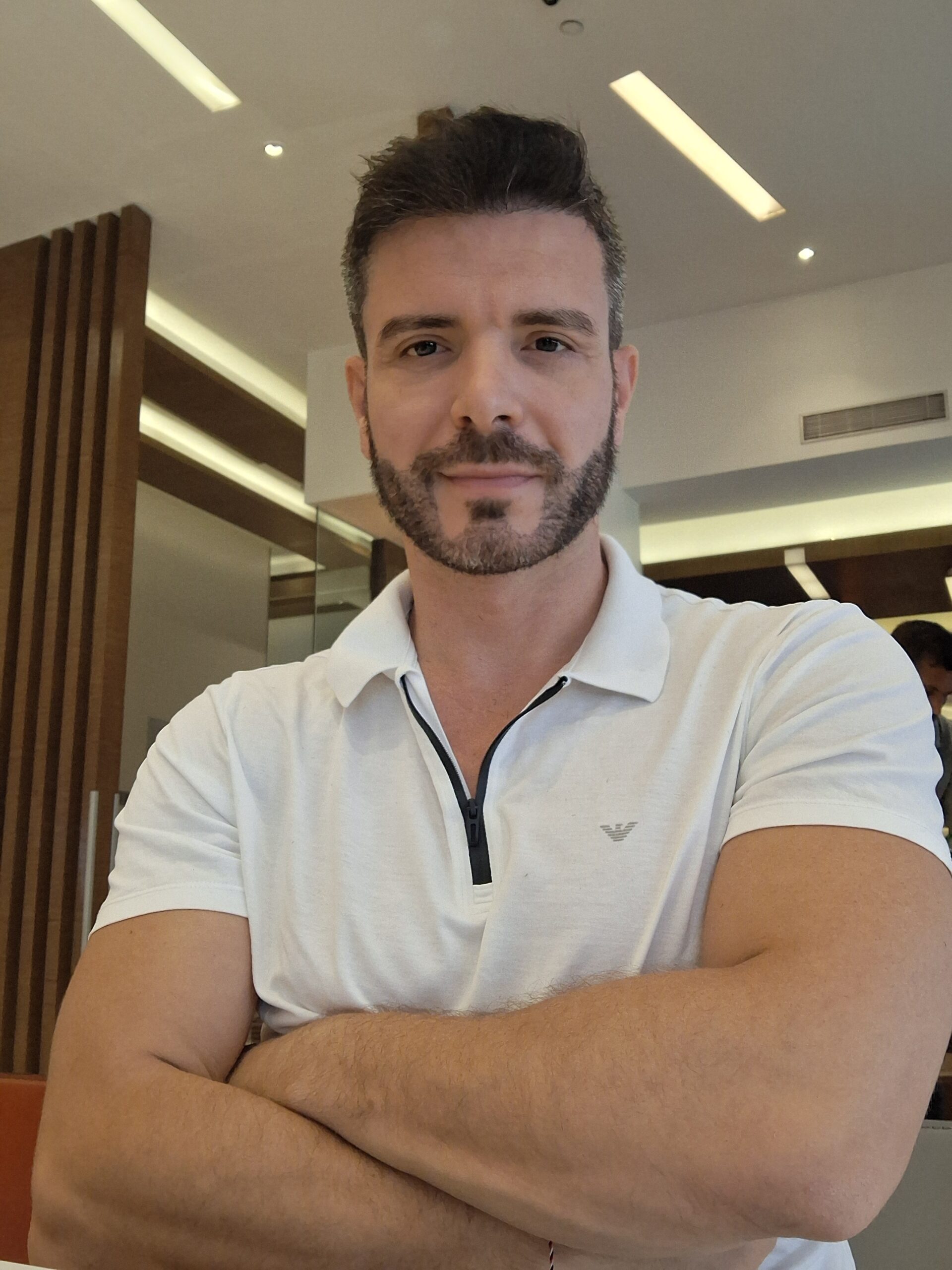
Helping businesses grow faster with AI. 🚀 At Callin.io, we make it easy for companies close more deals, engage customers more effectively, and scale their growth with smart AI voice assistants. Ready to transform your business with AI? 📅 Let’s talk!
Vincenzo Piccolo
Chief Executive Officer and Co Founder