The Dawn of Light-Based Computing
In the computing world, a photonic accelerator represents a groundbreaking shift from traditional electronic processing to light-based computation. These specialized hardware components leverage photons rather than electrons to perform complex calculations, offering unprecedented advantages in speed and energy efficiency. Unlike conventional silicon chips that rely on electrical signals, photonic accelerators manipulate light waves to execute computational tasks, effectively shattering the performance ceilings of electronic systems. This technological leap forwards isn’t merely incremental—it’s transformative, potentially resolving numerous bottlenecks that have hampered electronic computing for decades. The principles underpinning photonic computation draw from both quantum mechanics and classical optics, creating a hybrid approach that’s uniquely powerful for specific workloads. Research institutions like MIT’s Photonic Systems Lab and Lightmatter are spearheading innovations in this domain, rapidly advancing our understanding of photonic computing’s practical applications and implementation challenges.
Core Principles and Architecture
The fundamental architecture of a photonic accelerator revolves around optical components such as waveguides, interferometers, phase shifters, and photodetectors arranged in sophisticated configurations. These elements manipulate light’s properties—amplitude, phase, frequency, and polarization—to encode, process, and extract information. At their core, these accelerators typically feature an optical mesh network where light signals interfere constructively and destructively to perform matrix multiplications and other linear operations critical for machine learning workloads. The beauty of this approach lies in its inherent parallelism; unlike electronic systems that process data sequentially, photonic systems can handle multiple operations simultaneously through wavelength division multiplexing and spatial light processing. Advanced photonic integrated circuits (PICs) fabricate these components on silicon substrates using techniques like silicon photonics, which enables compatibility with existing semiconductor manufacturing infrastructure. This architectural paradigm enables computational densities orders of magnitude greater than electronic counterparts, particularly for specialized applications like neural network inference. For a deeper understanding of how such computational systems integrate with AI voice technologies, explore conversational AI for medical offices where similar advanced processing architectures are being deployed.
Breaking Speed Barriers with Light
The extraordinary speed advantages of photonic accelerators derive directly from physics—light travels approximately 300,000 kilometers per second, vastly outpacing electron movement in conductors. This fundamental difference translates to computational operations occurring at picosecond timescales rather than nanoseconds in electronic systems. These accelerators achieve data processing rates reaching multiple terabits per second, particularly excelling at operations demanding high throughput like Fast Fourier Transforms (FFTs) and large matrix multiplications. The latency reduction is especially significant for time-sensitive applications such as high-frequency trading, real-time signal processing, and autonomous vehicle control systems. Additionally, photonic processors eliminate the signal integrity issues that plague high-speed electronic interconnects, such as crosstalk and electromagnetic interference. Stanford’s Nanoscale and Quantum Photonics Lab has demonstrated photonic neural networks operating at speeds exceeding 100 trillion operations per second—figures that would require massive power consumption in traditional computing architectures. This speed advantage becomes particularly crucial for processing-intensive AI applications like those found in AI call centers, where response times directly impact customer experience.
Energy Efficiency Breakthrough
Perhaps the most compelling feature of photonic accelerators is their remarkable energy efficiency. Unlike electronic systems that generate substantial heat through resistance, photonic computing produces minimal thermal output during operation. This efficiency translates to energy consumption reductions of 10-1000Ă— compared to electronic counterparts performing equivalent calculations. The practical implications are enormous—from extending battery life in mobile devices to significantly decreasing the carbon footprint of data centers, which currently consume about 1-2% of global electricity. This energy advantage stems from light’s fundamental property of non-interacting photons, which enables signals to cross paths without interference—a physical impossibility for electrons. Companies like Lightmatter and Luminous Computing have demonstrated photonic AI accelerators that process neural networks at a fraction of the power budget required by GPUs. For business applications requiring intensive computational processing while maintaining energy efficiency, solutions like AI voice agents are beginning to incorporate photonic processing elements to handle complex conversational models with minimal power requirements.
Applications in Artificial Intelligence
The symbiotic relationship between photonic accelerators and artificial intelligence stands as perhaps the most promising computational partnership of this decade. These light-based processors excel particularly at matrix-vector multiplications and convolution operations—precisely the mathematical foundations of neural networks. Deep learning models that would require enormous GPU clusters can potentially run on considerably smaller photonic systems, demolishing barriers to AI deployment in resource-constrained environments. Computer vision applications benefit tremendously, with photonic processors capable of real-time image recognition at resolutions and frame rates previously unattainable. Natural language processing models like transformers, with their massive matrix operations, experience dramatic acceleration when implemented on photonic hardware. Several research labs, including those at MIT and University of Oxford, have demonstrated photonic implementations of neural networks achieving performance hundreds of times faster than electronic systems while consuming just fractions of the energy. This acceleration capability proves invaluable for sophisticated AI communication systems like AI voice conversations and AI call assistants, where computational demands can be substantial.
Integration with Quantum Computing
The fascinating intersection of photonic accelerators with quantum computing opens extraordinary possibilities for computational science. Photons naturally serve as excellent quantum information carriers, maintaining coherence over longer distances and timescales than many other quantum systems. This makes photonic platforms particularly promising for certain quantum algorithms and quantum machine learning applications. Hybrid systems combining classical photonic accelerators with quantum photonic elements represent an emerging frontier, potentially offering "quantum advantage" for specific problems while maintaining practical operability. Companies like PsiQuantum and Xanadu are developing photonic quantum computers that leverage similar optical infrastructure to photonic accelerators, creating potential technological synergies. These developments suggest a future computing landscape where problems are dynamically allocated to classical electronic, classical photonic, or quantum photonic processors depending on their specific requirements. The technological overlap between photonic accelerators and quantum systems creates fascinating opportunities for breakthrough applications in cryptography, molecular simulation, and optimization problems. For businesses developing advanced communication systems like conversational AI platforms, understanding these emerging computational paradigms becomes increasingly relevant.
Current Implementation Challenges
Despite their tremendous promise, photonic accelerators face substantial implementation challenges that have thus far limited their widespread adoption. Chip fabrication represents a significant hurdle, as the manufacturing precision required for photonic integrated circuits exceeds even the demanding standards of electronic semiconductors. Current fabrication techniques struggle with variation control at nanometer scales, resulting in performance inconsistencies across devices. Integration with existing electronic systems presents another obstacle; interfacing between photonic and electronic domains introduces conversion overhead that can diminish the speed advantages. Temperature sensitivity poses yet another challenge, as many photonic components exhibit performance variations with thermal fluctuations, necessitating precise environmental control systems. Perhaps most significantly, the programming paradigms for photonic computing remain in nascent stages, lacking the mature software ecosystems that electronic computing enjoys. Research efforts at Columbia Lightwave Research Laboratory and other institutions focus on addressing these challenges through advanced manufacturing techniques, innovative designs, and new programming frameworks. For businesses considering investment in advanced communications technology, platforms like AI phone services offer a more immediately accessible entry point while photonic technology matures.
Research Milestones and Breakthroughs
The accelerating pace of photonic accelerator research has produced remarkable scientific breakthroughs in recent years. In 2020, researchers at MIT demonstrated the first fully programmable photonic processor capable of executing general-purpose computing tasks, a milestone comparable to early electronic computers. The following year, Lightmatter unveiled their Envise photonic AI accelerator, showcasing practical implementation of optical neural network processing for data centers. In 2022, scientists at University of Oxford achieved record-breaking computational density with a photonic chip performing over 10^17 multiply-accumulate operations per second per cubic millimeter—orders of magnitude beyond electronic capabilities. More recently, researchers at EPFL developed error-correction techniques that significantly improve the precision of photonic calculations, addressing a longstanding limitation of optical computing. Current research focuses on expanding computational primitives beyond matrix operations, developing more efficient electro-optical interfaces, and creating software frameworks that abstract the physical complexities of photonic hardware. These advances progressively narrow the gap between theoretical capabilities and practical implementations, bringing photonic computing closer to commercial viability. Businesses exploring advanced AI communication systems like those offered through Twilio AI assistants should monitor these developments as they may reshape computational infrastructure.
Practical Applications in Data Centers
The integration of photonic accelerators into data centers represents one of their most promising near-term applications. These facilities already struggle with power constraints and cooling challenges that photonic computing directly addresses. Leading cloud providers have begun pilot programs implementing photonic co-processors for specific workloads, particularly AI inference tasks that benefit from the technology’s strengths. The results show not only performance improvements but significant operational cost reductions through decreased power consumption and cooling requirements. Beyond raw computational benefits, photonic interconnects between servers eliminate bandwidth bottlenecks in data center networks, enabling more efficient resource utilization across the facility. Companies like Intel and NVIDIA have invested substantially in photonic technologies, recognizing their strategic importance for next-generation computing infrastructure. Microsoft’s Project Sirius explores photonic computing specifically for enhancing cloud AI services, with projected efficiency gains exceeding 200Ă— for certain workloads. For businesses leveraging cloud-based communication systems like AI call center solutions, these developments promise more capable and responsive services at potentially lower costs as photonic technology becomes more widely deployed.
Edge Computing Revolution
The unique characteristics of photonic accelerators position them ideally for edge computing deployments, where power constraints and processing demands create challenging operating conditions. Their exceptional energy efficiency enables sophisticated AI processing in devices with limited power budgets, from smartphones to IoT sensors and autonomous vehicles. The reduced heat generation of photonic systems also eliminates the need for bulky cooling solutions, enabling more compact device designs for space-constrained applications. This technology could fundamentally transform capabilities at the network edge, allowing for real-time processing of complex sensor data without cloud connectivity. Several startups are developing specialized photonic edge processors targeting specific verticals: medical imaging devices capable of on-device tumor detection, augmented reality glasses with photonic neural engines for environmental understanding, and automotive lidar systems with integrated photonic processing for instantaneous obstacle recognition. Analog Photonics and other companies are pioneering miniaturized photonic integrated circuits specifically designed for edge deployment scenarios. These developments align with trends in distributed AI processing seen in applications like AI phone number services that perform complex conversational processing without centralized cloud dependencies.
Photonic Accelerators for Scientific Computing
Beyond commercial applications, photonic accelerators demonstrate exceptional promise for scientific computing tasks that have traditionally required supercomputing resources. Computational fluid dynamics, molecular dynamics simulations, and weather modeling all involve the massive matrix and vector operations where photonic systems excel. Research institutions including Los Alamos National Laboratory have begun exploring photonic computing for climate modeling, achieving preliminary results that suggest potential simulation speedups of 20-50Ă— compared to conventional supercomputing approaches. The inherent analog nature of photonic processors also offers unique advantages for differential equation solving, potentially revolutionizing simulation techniques across scientific disciplines. Particle physics experiments at facilities like CERN generate petabytes of data requiring real-time filtering and analysis—tasks where photonic accelerators’ throughput advantages could prove transformative. The energy efficiency benefits additionally align with sustainability goals increasingly prioritized in scientific computing facilities. As these applications develop, they create new computational approaches that eventually filter into commercial applications including the advanced processing required for AI sales representatives and other computationally intensive business applications.
Neuromorphic Computing Connection
The intersection of photonic accelerators with neuromorphic computing—systems designed to mimic neural structures and processes—represents a particularly fascinating frontier. Photonic neural networks can implement spiking neural models that more closely resemble biological brain function than conventional artificial neural networks. These systems leverage light’s wave properties to perform the complex, nonlinear operations characteristic of neural processing with remarkable efficiency. Research at Princeton Neuromorphic Computing Lab has demonstrated photonic implementations of neural networks that process information in ways fundamentally different from digital computers, potentially unlocking new approaches to artificial intelligence. The massively parallel nature of photonic systems aligns naturally with the parallel processing architecture of biological brains, enabling computational approaches previously impractical with sequential electronic systems. Several research groups have successfully implemented photonic reservoir computing—a specific neuromorphic approach particularly well-suited to time-series processing and speech recognition tasks. These developments suggest potential for qualitative improvements in AI capabilities beyond mere quantitative speedups, with particularly promising applications for systems requiring human-like interaction abilities such as AI voice agents used in customer service contexts.
Industry Leaders and Market Development
The photonic accelerator landscape features both established technology giants and specialized startups racing to commercialize this transformative technology. Intel has invested heavily in silicon photonics research, focusing on developing manufacturing processes compatible with existing semiconductor facilities. IBM Research maintains significant photonic computing initiatives, particularly targeting AI acceleration and quantum photonic systems. In the startup ecosystem, Lightmatter has raised over $180 million to develop photonic AI accelerators, with early products now being tested by select data center customers. Lightelligence focuses on photonic chips for edge AI applications, demonstrating working prototypes with dramatic performance improvements over electronic equivalents. Optalysys takes a slightly different approach, developing optical processing systems specifically for encryption and mathematical operations. Market analysts project the photonic computing market to grow from approximately $500 million in 2022 to over $5 billion by 2028, reflecting increasing commercial adoption as the technology matures. Businesses exploring advanced communication systems like AI call centers should monitor these market developments as they may significantly impact future computational infrastructure options.
Telecommunications and Networking Applications
The natural affinity between photonic accelerators and optical telecommunications networks creates compelling synergies for next-generation communication systems. Telecom infrastructure already utilizes photonics extensively for data transmission; integrating computational photonics enables processing within the optical domain without costly optical-electrical-optical conversions. This integration facilitates real-time network traffic analysis, adaptive routing optimizations, and sophisticated quality-of-service management directly within network equipment. Photonic signal processing can implement advanced modulation schemes and error correction algorithms that dramatically improve bandwidth utilization in fiber optic networks. Companies like Nokia Bell Labs and Cisco Systems are exploring photonic processing elements for backbone routers and switches, potentially increasing throughput while reducing power consumption by 80-90%. Beyond infrastructure applications, photonic computing enables sophisticated edge processing within telecom networks, supporting distributed AI models for services like AI voice assistants and real-time language translation. The communication industry’s existing expertise with optical technologies may accelerate commercial adoption of photonic computing in this sector ahead of other applications, creating valuable implementation experience and market validation.
Software Ecosystems and Programming Paradigms
The development of appropriate software ecosystems represents one of the most significant challenges for photonic accelerator adoption. Programming these devices requires fundamentally different approaches than electronic computing, necessitating new abstractions, compilers, and development tools. Several research groups are creating domain-specific languages designed specifically for expressing computations optimized for photonic implementation. Companies like Lightelligence are developing compilation tools that can transparently translate portions of machine learning frameworks like TensorFlow and PyTorch to execute on photonic hardware. The programming paradigms emerging combine elements from analog computing, wave physics, and digital signal processing in novel ways that often lack direct analogs in conventional programming. Leading academic institutions including Stanford and MIT offer specialized courses on photonic computing architecture and programming, gradually building the human expertise necessary for broader adoption. These software developments will determine whether photonic accelerators remain specialized co-processors for narrow applications or evolve into more general-purpose computing platforms. Businesses developing sophisticated AI applications like AI appointment schedulers should consider how these emerging computational paradigms might eventually transform their development approaches.
Future Roadmap and Technological Trajectory
The technological roadmap for photonic accelerators suggests several distinct phases of development and adoption over the coming decade. Near-term implementations will focus primarily on photonic co-processors for specific workloads within otherwise conventional computing systems. As manufacturing processes mature and yields improve, we’ll likely see more integrated photonic-electronic systems with tighter coupling between domains. Long-term projections envision "photonic-first" computing architectures where the majority of processing occurs in the optical domain, with electronics handling only specialized tasks. Research directions include developing novel optical nonlinear materials that enable more complex photonic operations, integrated quantum photonic elements for hybrid classical-quantum systems, and three-dimensional photonic circuits that exploit the full spatial dimensionality of light. The eventual convergence of photonic computing with advances in materials science, particularly two-dimensional materials like graphene and transition metal dichalcogenides, promises another wave of capability improvements. For businesses planning long-term technology strategies, understanding this roadmap helps inform decisions about when investment in photonic-enabled systems like advanced AI voice conversations might become strategically advantageous.
Economic Implications and Cost Analysis
The economic case for photonic accelerators grows increasingly compelling as the technology matures and manufacturing scales. Initial devices carry premium pricing reflecting both development costs and limited production volumes, with photonic accelerator cards currently costing 3-5Ă— equivalent electronic hardware. However, operating cost analyses tell a different story—the dramatic energy savings can deliver positive return on investment within 1-2 years for power-intensive applications like AI training and high-performance computing. As manufacturing volumes increase, production costs should decline significantly given that the base materials (silicon, germanium, indium phosphide) aren’t inherently more expensive than those used in electronic chips. Data center deployments represent the economic beachhead, where the combination of performance gains and operating cost reductions creates the most compelling business case. Several cloud providers now offer photonic acceleration as premium services for specific workloads, creating initial commercial validation. For enterprises evaluating technology investments, photonic acceleration may initially appear most accessible through cloud services rather than direct hardware acquisition, similar to how businesses currently access advanced AI capabilities through platforms like conversational AI services rather than building complex infrastructure themselves.
Ethical and Environmental Considerations
The emergence of photonic accelerators raises important ethical and environmental considerations that merit thoughtful examination. From an environmental perspective, the technology offers significant sustainability benefits through drastically reduced energy consumption compared to electronic alternatives. This efficiency could substantially decrease the carbon footprint of computing infrastructure, particularly important as AI systems grow increasingly power-hungry. However, manufacturing processes for photonic chips currently involve certain rare materials and potentially toxic compounds, creating different environmental challenges that require careful management. From an ethical standpoint, the dramatic performance improvements enabled by photonic computing could further concentrate technological power in the hands of organizations with resources to deploy these systems first. Ensuring equitable access to photonic computing capabilities represents an important consideration for academic institutions and public sector organizations. Additionally, the potential for photonic systems to enable more sophisticated surveillance and security applications necessitates appropriate governance frameworks. Organizations like the IEEE Standards Association are beginning to develop technical standards for photonic computing that incorporate ethical considerations alongside technical specifications. Businesses deploying advanced AI systems like AI call assistants should consider these broader implications as part of their technology governance approach.
Comparison with Competing Technologies
While photonic accelerators demonstrate remarkable capabilities, they exist within a competitive landscape of emerging computing technologies. Specialized electronic AI chips like Google’s TPUs and NVIDIA’s latest generation GPUs continue rapid advancement, narrowing some of photonic computing’s advantages. Neuromorphic electronic systems from companies like Intel Neuromorphic Research pursue energy efficiency through brain-inspired architectures, offering an alternative path to more efficient computing. Various quantum computing approaches—including superconducting qubits, trapped ions, and silicon spin qubits—target fundamentally different computational problems than photonic accelerators, suggesting complementary rather than competing roles in future computing ecosystems. Each technology presents distinct tradeoffs in performance, energy efficiency, manufacturability, and programming complexity. The most likely outcome involves specialized deployment based on workload characteristics: photonic systems for matrix-heavy computations, quantum systems for specific algorithms where they demonstrate advantage, and advanced electronic systems maintaining their general-purpose role. For businesses developing application-specific solutions like SIP trunking services or AI voice agents, the optimal approach involves selecting the most appropriate computational architecture for their specific requirements rather than universally adopting any single technology.
Case Studies: Early Adopters and Success Stories
Several pioneering organizations have already begun implementing photonic accelerators, providing valuable real-world validation of their capabilities. JP Morgan Chase partnered with Lightelligence to deploy photonic processing for option pricing models, reporting 50Ă— speedups and 70% energy reduction compared to their previous GPU-based system. A leading pharmaceutical company (requesting anonymity) implemented photonic computing for molecular dynamics simulations, accelerating drug discovery pipelines by identifying promising compounds months faster than conventional methods. In the public sector, the United States National Oceanic and Atmospheric Administration (NOAA) began testing photonic processors for specific climate modeling calculations, achieving preliminary results suggesting potential for more detailed models with existing power budgets. These early implementations typically focus on narrow, well-defined computational tasks rather than general-purpose processing, highlighting the current specialization of photonic accelerators. Common themes across success stories include careful workload analysis to identify photonic-suitable processing tasks, comprehensive cost-benefit analysis including both acquisition and operating expenses, and integration planning that minimizes disruption to existing workflows. Organizations considering advanced AI deployments like AI cold callers can draw valuable lessons from these early adoption strategies.
Transforming Telecommunications with Photonic Intelligence
The telecommunications industry stands poised for remarkable transformation through photonic accelerators that bring computation directly into the optical domain where data already travels. Network equipment incorporating photonic processing elements can perform real-time traffic analysis, security screening, and quality-of-service optimization without converting signals to the electronic domain. This capability dramatically improves throughput while reducing latency and power consumption—critical metrics for modern telecommunications infrastructure. Several major carriers are piloting photonic-enhanced routing equipment capable of dynamic traffic shaping based on real-time content analysis, improving user experience for time-sensitive applications like video conferencing and online gaming. The integration of photonic computing with 5G and upcoming 6G networks appears particularly promising, enabling sophisticated edge processing directly within cellular infrastructure. Companies like Nokia Bell Labs are developing photonic signal processing for next-generation wireless networks, demonstrating significant improvements in spectrum utilization efficiency. These developments complement advanced communication services like Twilio AI phone calls and similar platforms by potentially providing more capable infrastructure for their operation, with particular benefits for applications requiring real-time processing of voice and language.
Embracing the Future of Computational Light
As we’ve explored throughout this article, photonic accelerators represent far more than incremental improvement to existing computing paradigms—they fundamentally reimagine how computation occurs by harnessing light’s unique physical properties. The technology offers transformative potential across diverse domains from artificial intelligence to scientific computing, telecommunications, and edge processing. While challenges remain in manufacturing, programming, and integration, the trajectory of advancement suggests increasingly practical implementations emerging over the coming years. Organizations across industries should begin evaluating where photonic computing might create strategic advantages for their specific computational needs, particularly for matrix-heavy workloads that align with photonic strengths. Educational institutions and workforce development programs should consider incorporating photonic computing concepts into relevant curricula, preparing for increased demand for expertise in this emerging field. The transition to photonic computing represents not merely a change in hardware but potentially a fundamental shift in how we conceptualize and implement computational solutions to complex problems.
Illuminating Your Business with Next-Generation Communication Technologies
The revolutionary potential of photonic computing explored throughout this article represents just one facet of the technological transformation reshaping business communications and operations. While photonic accelerators may still be emerging technology, advanced AI communication systems that leverage cutting-edge computational approaches are already accessible to businesses of all sizes. If you’re looking to enhance your organization’s communication capabilities with innovative technology available today, Callin.io offers an ideal starting point.
Callin.io’s platform enables you to deploy sophisticated AI phone agents that can independently handle inbound and outbound calls, automate appointment scheduling, answer frequently asked questions, and even close sales through natural-sounding conversations. Unlike experimental technologies, these solutions deliver immediate practical benefits—freeing your staff from routine call handling, ensuring consistent customer experiences, and providing 24/7 availability without increasing personnel costs.
The free Callin.io account provides an intuitive interface for configuring your AI agent, complimentary test calls to experience the technology firsthand, and access to a comprehensive task dashboard for monitoring interactions. For businesses requiring advanced features like Google Calendar integration and built-in CRM functionality, subscription plans start at just $30 monthly. Discover how Callin.io can transform your business communications today while the photonic revolution continues developing in research labs worldwide.
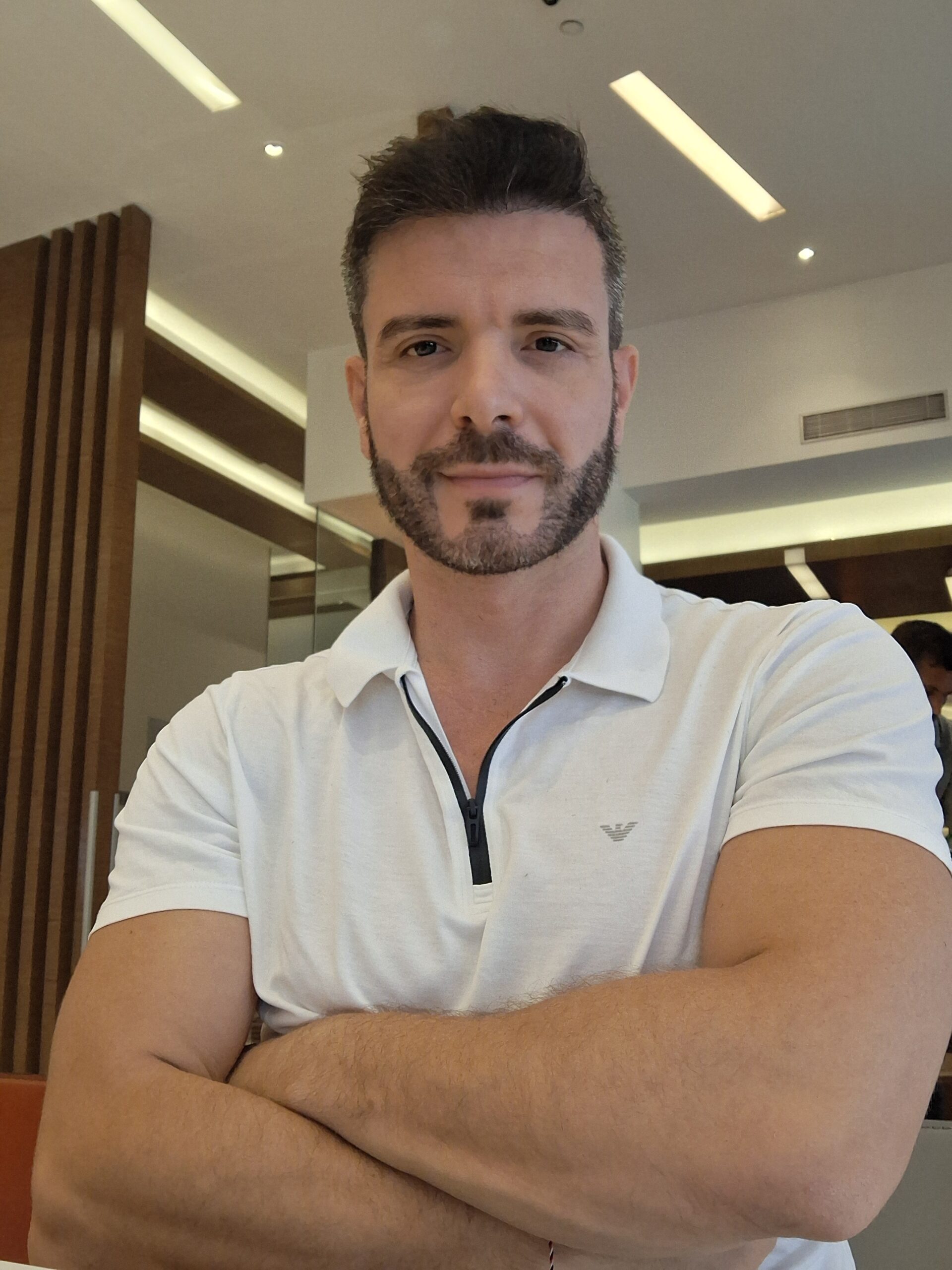
Helping businesses grow faster with AI. 🚀 At Callin.io, we make it easy for companies close more deals, engage customers more effectively, and scale their growth with smart AI voice assistants. Ready to transform your business with AI? 📅 Let’s talk!
Vincenzo Piccolo
Chief Executive Officer and Co Founder