The Evolution of Call Center Automation
Call center operations have undergone a remarkable transformation in recent years, primarily driven by robotic automation technologies that are reshaping how businesses handle customer interactions. This evolution represents a significant shift from traditional manual call handling to sophisticated automated systems capable of managing complex customer service scenarios. The journey from basic interactive voice response (IVR) systems to today’s AI-powered virtual agents illustrates how far the industry has come. According to a recent study by McKinsey, organizations implementing call center robotic automation have seen operational costs decrease by 15-25% while simultaneously improving customer satisfaction scores. This technological progression aligns perfectly with modern businesses seeking efficiency without sacrificing service quality, similar to the transformative impact of conversational AI in medical offices that has revolutionized patient communication systems.
Understanding Robotic Process Automation (RPA) in Call Centers
Robotic Process Automation, commonly abbreviated as RPA, forms the backbone of modern call center automation strategies. At its core, RPA involves deploying software robots or "bots" that can mimic human actions to execute rule-based tasks with precision and consistency. In call centers, these digital workers handle repetitive processes like data entry, customer information retrieval, and post-call documentation, allowing human agents to focus on complex problem-solving and emotional engagement. The technology utilizes screen scraping, workflow automation, and application programming interfaces (APIs) to interact with existing systems without requiring extensive IT infrastructure changes. For instance, when a customer calls with a routine billing inquiry, RPA bots can instantly access account information across multiple databases, dramatically reducing the handling time from minutes to seconds. Organizations planning to implement these solutions might benefit from exploring AI call center implementation guides to better understand the technical requirements and integration processes necessary for successful deployment.
AI-Powered Voice Assistants: The New Call Center Agents
The integration of advanced artificial intelligence with voice technology has given rise to sophisticated AI voice agents that are increasingly handling front-line customer interactions. These virtual assistants leverage natural language processing (NLP) and machine learning algorithms to understand customer intent, respond appropriately, and even detect emotional cues in voice patterns. Unlike their scripted predecessors, modern AI voice assistants can maintain contextual awareness throughout conversations, making interactions feel remarkably human-like. Companies like American Express have implemented AI voice systems that can authenticate customers, process payments, and resolve disputes without human intervention. The technology has matured to the point where AI phone services can now handle complex scenarios that previously required human judgment, such as negotiating payment plans or providing personalized product recommendations based on customer history and preferences. The continuous learning capability of these systems means they improve with each interaction, gradually expanding their knowledge base and conversational abilities.
Conversational AI and Its Impact on Customer Experience
Conversational AI represents the cutting edge of call center automation, enabling natural, fluid dialogues between customers and automated systems. This technology combines various AI components—speech recognition, natural language understanding, dialogue management, and response generation—to create seamless conversations that can address customer needs effectively. The impact on customer experience has been profound, with Twilio’s conversational AI and similar platforms enabling interactions that feel less robotic and more empathetic. For example, Delta Airlines implemented a conversational AI system that can handle over 40,000 customer conversations daily across multiple channels, resolving issues like flight changes and baggage claims with minimal friction. Research from Gartner indicates that businesses utilizing conversational AI in their call centers have seen customer satisfaction scores increase by up to 25%, primarily because customers appreciate the reduced wait times and 24/7 availability. Additionally, these systems excel at maintaining consistent service quality across all interactions, eliminating the variability often experienced with human agents working different shifts or experiencing different stress levels.
The Economics of Call Center Automation
The financial implications of implementing robotic automation in call centers extend far beyond the obvious labor cost reductions. A comprehensive cost-benefit analysis reveals multiple economic advantages that make the initial investment in automation technology increasingly justifiable for businesses of all sizes. The average call center experiences a 40-60% reduction in average handling time for routine inquiries after implementing robotic automation, while error rates in transaction processing typically decrease by 35-65%. Companies like T-Mobile have reported annual savings exceeding $5 million after deploying AI call assistants across their customer service operations. Beyond direct cost savings, there’s compelling evidence of revenue enhancement through improved upselling capabilities—automated systems consistently apply best-practice sales techniques and never forget to offer relevant add-ons or upgrades. Financial services giant JP Morgan Chase implemented call center automation that not only reduced operational costs by $11 billion but also decreased contract processing times from 16 days to just one day, significantly accelerating revenue realization. For smaller businesses considering similar implementations, exploring affordable SIP carriers can provide cost-effective telecommunication infrastructure to support automation initiatives.
Integration Challenges and Solutions
Implementing robotic automation in established call centers often presents significant integration challenges that require careful navigation. Legacy systems with outdated architectures frequently resist seamless connection with modern automation platforms, creating potential disruption to existing operations. Organizations commonly encounter data silos, where critical customer information remains trapped in disparate systems, limiting the effectiveness of automated processes. Successful implementations typically employ middleware solutions that act as translational layers between legacy infrastructure and new automation tools. Companies like Vodafone addressed their integration challenges by implementing a phased approach—starting with simple, low-risk processes before advancing to more complex customer interactions. This gradual strategy allowed for continuous learning and adjustment while maintaining service continuity. Another effective approach involves creating a unified customer data platform that consolidates information from various sources before implementing automation, as exemplified by Bank of America’s successful call center transformation. For businesses seeking streamlined integration, white label AI call center solutions offer pre-configured systems that can significantly reduce implementation complexity and accelerate deployment timelines from months to weeks.
Workforce Transformation in Automated Call Centers
The introduction of robotic automation fundamentally transforms the workforce dynamics within call centers, necessitating strategic human resource management to navigate this transition successfully. Rather than wholesale replacement of human agents, forward-thinking organizations are embracing a collaborative human-AI approach that redefines job roles and creates new career paths. Agents previously handling routine queries are being upskilled to become "automation supervisors" who monitor, train, and improve automated systems. Companies like Humana have implemented comprehensive reskilling programs focused on developing employees’ analytical abilities, emotional intelligence, and complex problem-solving skills—attributes that complement rather than compete with automation capabilities. This workforce transformation has led to the emergence of specialized roles such as conversation designers who craft optimal dialogue flows, automation trainers who refine AI models, and escalation specialists who handle complex cases beyond AI capabilities. According to research from Deloitte, call centers implementing automation while investing in workforce development report 32% higher employee satisfaction and 28% lower turnover rates compared to those focusing solely on technology deployment. Organizations contemplating similar transformations might benefit from exploring how AI voice assistants handle FAQs to better understand which tasks can be automated and which require human intervention.
Data Security and Compliance in Automated Call Centers
Security and regulatory compliance remain paramount concerns as call centers increasingly adopt robotic automation technologies that process sensitive customer information. Automated systems must adhere to a complex web of regulations including GDPR, HIPAA, PCI DSS, and industry-specific mandates that govern data handling practices. The automation itself introduces unique security considerations—robotic processes typically require comprehensive system access across multiple platforms, creating potential vulnerability points if not properly secured. Leading organizations address these challenges through rigorous access control protocols, end-to-end encryption for data in transit and at rest, and regular security audits of automated workflows. Companies like Cigna have implemented sophisticated audit trail capabilities that record every action taken by automated systems, ensuring complete accountability and facilitating compliance verification. Additionally, implementing "zero-knowledge" protocols where automation tools process data without storing it has emerged as a best practice for maintaining customer privacy. Organizations in highly regulated industries are increasingly adopting AI call center solutions with built-in compliance features such as automatic call redaction for sensitive information and real-time compliance monitoring that flags potential violations before they occur.
Measuring Success: KPIs for Automated Call Centers
Establishing appropriate key performance indicators (KPIs) is essential for evaluating the effectiveness of robotic automation implementations in call center environments. The metrics for success extend beyond traditional call center measurements to encompass automation-specific indicators that provide a comprehensive view of performance improvements. First-contact resolution rate has emerged as a critical KPI, with automated systems typically improving this metric by 15-30% through consistent application of best practices and immediate access to complete customer information. Containment rate—the percentage of customer inquiries fully resolved by automated systems without human intervention—provides direct insight into automation effectiveness, with industry leaders achieving rates above 75% for routine inquiries. Beyond operational metrics, customer-centered KPIs such as Customer Effort Score (CES) and Net Promoter Score (NPS) reveal the actual impact on customer experience, with Verizon reporting a 22-point NPS improvement after implementing AI phone agents. Financial metrics including cost-per-contact, automation ROI, and revenue generation per automated interaction provide the business justification for continued investment. Progressive organizations are also tracking automation learning curve metrics that measure how quickly the automated systems improve over time through machine learning and continuous improvement cycles.
Self-Service Portals and Automation Synergy
The strategic integration of self-service portals with call center automation creates a powerful synergy that maximizes operational efficiency while enhancing customer satisfaction. Modern self-service platforms serve as the first line of engagement, handling straightforward transactions and information requests through intuitive interfaces, while robotic automation manages the exceptions and more complex scenarios that emerge from these interactions. Companies like American Airlines have implemented sophisticated handoff protocols that seamlessly transfer customers from web or mobile self-service to automated voice systems when needed, carrying context and customer information across channels to eliminate repetitive information gathering. Data analysis reveals that effective self-service integration typically reduces call volume by 25-40%, allowing automation resources to focus on higher-value interactions. The technological connection between these systems often employs webhooks, APIs, and unified customer identity management to create a coherent experience regardless of entry point. Organizations looking to implement similar multi-channel approaches might benefit from Twilio AI calling solutions to connect their existing web infrastructure with automated voice capabilities. Additionally, leading implementations leverage customer behavior analytics across self-service and automated voice channels to continuously optimize both systems based on actual usage patterns.
The Role of Machine Learning in Continuous Improvement
Machine learning capabilities form the cornerstone of truly effective call center automation systems, enabling continuous improvement through ongoing analysis of interaction data. Unlike static rule-based systems, ML-powered automation continuously refines its performance based on millions of customer interactions, identifying patterns and optimization opportunities that would remain invisible to human analysts. For instance, TD Bank’s implementation of machine learning in their automated customer service system analyzes conversation flows to identify common points of customer confusion, automatically adjusting dialogue patterns to improve clarity without requiring manual reprogramming. Sentiment analysis algorithms examine vocal tones and word choices to detect customer frustration early in conversations, triggering appropriate response modifications or proactive escalation to human agents. Companies like Capital One employ reinforcement learning techniques where their automated systems receive continuous feedback on successful and unsuccessful interactions, gradually optimizing conversation strategies to maximize positive outcomes. This perpetual improvement capability represents a significant advantage over traditional automation approaches, with ML-powered systems typically demonstrating a 5-8% performance improvement quarterly compared to the 1-2% improvement seen in conventional systems. Organizations seeking similar capabilities might explore AI voice conversation technologies that incorporate advanced learning algorithms to power their customer service automation.
Omnichannel Integration with Robotic Automation
The integration of robotic automation across multiple communication channels creates a unified customer experience that significantly enhances service quality and operational efficiency. Modern call center automation extends beyond voice interactions to encompass chat, email, social media, and messaging platforms through a coordinated omnichannel strategy. Companies like Zappos have implemented centralized automation engines that maintain consistent customer knowledge and conversation context regardless of which channel a customer uses to make contact. This omnichannel approach typically reduces resolution times by 35-50% by eliminating the need for customers to repeat information when switching between channels. Sophisticated implementations employ unified customer profiles that aggregate interaction data across all touchpoints, allowing automated systems to provide personalized service based on complete relationship history rather than isolated interactions. The technology underpinning these integrations often involves specialized middleware that standardizes data formats across disparate systems while maintaining the unique characteristics of each channel. For businesses seeking to implement omnichannel capabilities, platforms like Twilio AI Assistants provide ready-made solutions that can dramatically reduce integration complexity while enabling consistent automation across multiple communication channels.
Handling Exceptions and Escalations
A sophisticated exception management framework represents an essential component of successful call center automation implementations, addressing the inevitable situations where robotic processes encounter scenarios beyond their capabilities. Rather than viewing exceptions as failures, forward-thinking organizations treat them as valuable learning opportunities that strengthen the overall system. Industry leaders like USAA have developed nuanced escalation architectures that include multiple intervention levels—from automation self-correction to specialized human expert involvement—depending on the complexity of the exception encountered. Effective implementations typically feature warm transfer protocols that provide human agents with complete context when receiving escalations, eliminating customer frustration from having to repeat information. Analytics applied to escalation patterns reveal systematic improvement opportunities, with companies like Northwestern Mutual using this data to prioritize automation enhancements that address the most frequent or costly exceptions. The most advanced implementations utilize predictive analytics to anticipate potential exceptions before they occur, proactively routing complex cases to appropriate resources before customer frustration develops. Skills-based routing algorithms ensure that when human intervention becomes necessary, the customer reaches an agent specifically qualified to address their particular issue, significantly improving first-contact resolution rates for escalated cases. For organizations seeking to implement similar capabilities, exploring AI call center companies can provide insights into mature exception handling frameworks.
Emotional Intelligence in Automated Systems
The incorporation of emotional intelligence capabilities represents the frontier of call center automation development, enabling robotic systems to detect, interpret, and appropriately respond to customer emotions. Advanced implementations analyze paralinguistic features—tone, pitch, speaking rate, and micro-pauses—to gauge emotional states with surprising accuracy. Companies like Discover Financial have deployed emotion-aware systems that can detect rising frustration and adapt responses accordingly, either by offering additional assistance or preemptively transferring to human agents before negative experiences escalate. Beyond detection, cutting-edge systems employ sophisticated response models that adjust language patterns, pacing, and content based on emotional context—using more empathetic phrasing with distressed customers or more direct approaches with those exhibiting impatience. Research from MIT indicates that emotion-aware automated systems achieve 28% higher customer satisfaction scores compared to traditional automation that lacks this capability. Some implementations incorporate visual sentiment analysis for video interactions, detecting facial expressions that provide additional emotional context beyond voice cues alone. Organizations looking to enhance their customer service with emotion-aware capabilities might explore voice synthesis technologies that support the subtle vocal modulations necessary for conveying appropriate emotional tones in automated responses.
Personalization at Scale Through Automation
Robotic automation enables call centers to deliver personalized customer experiences at unprecedented scale by combining data analytics with adaptive interaction capabilities. Unlike traditional mass-service approaches, modern automation systems leverage comprehensive customer data—including past purchases, service history, communication preferences, and behavioral patterns—to tailor each interaction uniquely. Companies like Netflix have implemented systems that dynamically generate millions of distinct conversation paths based on individual customer profiles, making automated interactions feel remarkably personal despite their technological nature. The personalization extends beyond simple name recognition to include substantive content customization—proactively addressing typical concerns for the customer’s specific products, referencing relevant past interactions, and adjusting communication style to match personal preferences. Research from Accenture reveals that 91% of consumers are more likely to patronize businesses that provide personalized recommendations and offers, highlighting the business value of automation-enabled personalization. Leading implementations utilize machine learning to continuously refine personalization algorithms based on interaction outcomes, gradually improving relevance and effectiveness without requiring manual intervention. Organizations seeking similar personalization capabilities might explore AI receptionist solutions that can be trained with company-specific knowledge to deliver tailored experiences to each caller.
Case Study: Banking Sector Automation Success
The banking industry has emerged as a pioneer in call center robotic automation, with numerous institutions demonstrating exceptional results that provide valuable implementation insights. JPMorgan Chase’s implementation of conversational AI for mortgage servicing represents a particularly instructive case, handling over 350,000 customer inquiries monthly with a remarkable 92% accuracy rate. Their phased deployment strategy began with simple balance inquiries before progressively adding capabilities including payment processing, disputes handling, and eventually complex advisory services. The bank reported annual savings exceeding $15 million while simultaneously improving their Net Promoter Score by 18 points. Similarly, Santander Bank’s automation journey illustrates the workforce transformation aspect, with 78% of their call center employees transitioning to higher-value roles following automation implementation—contradicting fears of widespread job elimination. Their success hinged on comprehensive retraining programs that developed employees’ analytical and oversight capabilities. Both institutions emphasized the importance of continuous tuning based on real customer interactions, with JPMorgan dedicating a specialized team to analyze automation performance and implement bi-weekly enhancements based on interaction data. Organizations in various industries can apply these lessons by exploring similar technologies like AI appointment scheduling systems that incorporate the same conversational intelligence principles pioneered by these banking leaders.
Future Trends: Predictive Service and Proactive Automation
The future of call center automation extends beyond reactive customer service toward predictive models that address customer needs before they explicitly arise. Cutting-edge implementations are already deploying anticipatory systems that analyze patterns in customer behavior, product usage, and environmental factors to predict potential service requirements. For example, American Express has implemented systems that detect unusual spending patterns and proactively contact customers about potential fraud before customers notice suspicious transactions themselves. Telecommunications provider Vodafone utilizes network monitoring data to predict potential service disruptions and automatically notify affected customers with estimated resolution times before they experience problems. The most sophisticated implementations combine multiple data streams—including IoT device signals, weather data, and social media sentiment—to construct comprehensive predictive models that anticipate service needs with remarkable accuracy. Research from Salesforce indicates that 62% of customers now expect companies to anticipate their needs, highlighting the growing importance of these predictive capabilities. Beyond individual predictions, pattern recognition across large customer populations enables systemic service improvements that reduce future call volumes by addressing root causes. Organizations seeking to implement similar forward-looking approaches might explore AI calling technologies that enable proactive outreach based on predictive signals from their existing customer data.
Implementing Voice Biometrics for Enhanced Security
Voice biometric authentication represents a significant advancement in call center security, enabling automated systems to verify customer identities through unique vocal characteristics rather than easily forgotten passwords or vulnerable personal information. This technology analyzes over 100 distinct voice features—including harmonic patterns, speech cadence, and formant frequencies—to create a voiceprint as unique as a fingerprint but far less intrusive to verify. Financial institutions like HSBC have implemented voice biometrics that reduce authentication time from 45 seconds to under 10 seconds while simultaneously increasing security and reducing fraud by 50% in their automated call systems. Beyond the security benefits, customers report significantly higher satisfaction with biometric authentication compared to traditional knowledge-based methods, appreciating the elimination of frustrating security questions. Advanced implementations employ continuous authentication that verifies identity throughout the conversation rather than just at the beginning, providing persistent security without additional customer effort. Some systems incorporate anti-spoofing capabilities that can detect recorded voices or synthetic speech attempts, addressing sophisticated fraud vectors. For organizations considering voice biometric implementation, solutions that combine this technology with other AI calling capabilities can provide a comprehensive approach to both security and service efficiency in automated call handling.
ROI Analysis: Making the Business Case for Automation
Constructing a comprehensive return on investment analysis provides essential justification for call center automation initiatives by quantifying both direct financial benefits and indirect value creation. Detailed ROI calculations typically reveal payback periods between 9-14 months for comprehensive automation implementations, with ongoing annual returns exceeding 250% of initial investment for mature deployments. Direct cost savings emerge from multiple sources: labor optimization typically reduces staffing requirements by 25-40% for routine inquiries, while error reduction decreases costly remediation activities by 35-60%. Infrastructure savings accrue through reduced need for physical call center space, with companies like American Express reporting 30% reduction in real estate requirements following automation implementation. Revenue enhancement represents a frequently underestimated benefit, with sophisticated automation improving upsell conversion rates by 15-25% through consistent application of optimized sales techniques. Customer retention improvements directly impact lifetime value calculations, with research indicating that customers experiencing efficient automated service demonstrate 28% higher retention rates compared to those facing long wait times for human agents. Organizations contemplating automation investments might benefit from exploring AI sales solutions that combine service automation with revenue generation capabilities, potentially accelerating ROI realization through dual-purpose deployment.
Global Implementation Considerations and Cultural Adaptation
Successfully deploying call center automation across international markets requires thoughtful adaptation to linguistic, cultural, and regulatory variations that significantly impact customer experience. Global enterprises like Samsung have developed regionalized automation frameworks that maintain consistent core capabilities while adapting conversation styles, problem resolution approaches, and compliance mechanisms to local expectations. Linguistic considerations extend beyond simple translation to include dialect recognition, culturally appropriate phrasing, and market-specific terminology—critical factors in achieving the 95%+ comprehension rates necessary for effective automation. Companies like Airbnb have created specialized cultural adaptation teams that modify automated interactions based on region-specific communication norms, such as adjusting directness levels, formality, and problem-solving approaches to align with local preferences. Regulatory requirements vary dramatically across jurisdictions, necessitating configurable compliance frameworks that accommodate differences in data protection laws, disclosure requirements, and consumer rights provisions. Time zone management represents another crucial consideration, with sophisticated implementations employing follow-the-sun strategies that shift automation resources based on global activity patterns. For organizations planning international deployments, exploring specialized solutions like German AI voice technology can provide insights into the localization capabilities necessary for successful multi-market implementation.
Emerging Technologies Shaping Future Call Center Automation
The rapid evolution of adjacent technologies is continuously expanding the capabilities and applications of call center robotic automation. Quantum computing developments promise to revolutionize natural language processing capabilities by enabling real-time analysis of conversational nuances that currently exceed computational limits. Meanwhile, advances in brain-computer interfaces at companies like Neuralink suggest future possibilities for thought-directed service interactions that bypass traditional communication channels entirely. Extended reality (XR) technologies are beginning to enable hybrid automated service experiences where visual information supplements voice interactions, with companies like Ikea experimenting with automated support systems that can visually guide customers through product assembly or troubleshooting. Federated learning approaches are addressing privacy concerns by enabling AI systems to learn from distributed data without centralizing sensitive information, allowing more personalized automation while maintaining robust privacy protections. Ambient computing concepts are extending automated service beyond designated channels into environmental contexts, with voice assistants increasingly acting as gateways to service automation throughout customers’ daily activities. For organizations seeking to position themselves at the cutting edge of these developments, exploring technologies like conversational AI platforms can provide a foundation for incorporating these emerging capabilities as they mature and become commercially viable.
Transforming Your Business with Call Center Automation
Implementing robotic automation in your call center represents a transformative opportunity to simultaneously enhance customer experience, reduce operational costs, and create strategic advantages in your market. The technology has matured beyond experimental applications to become a proven business accelerator with well-established implementation methodologies. Companies across industries have demonstrated that thoughtfully deployed automation can reduce average handling times by 40-65% while improving customer satisfaction scores by 15-30% through consistent, high-quality service delivery. The competitive implications are significant—organizations that successfully implement automation typically achieve cost structures 20-35% lower than competitors relying on traditional approaches, creating substantial pricing flexibility or margin advantages. Beyond the direct benefits, automated systems generate unprecedented quantities of interaction data that enable continuous service optimization and reveal valuable customer insights that can inform product development and marketing strategies. For businesses ready to embark on this transformation journey, Callin.io offers a comprehensive platform that combines cutting-edge AI technology with simple implementation processes. Their solution allows organizations to rapidly deploy sophisticated AI voice agents without extensive technical expertise or infrastructure investment, providing an accessible entry point to call center automation benefits regardless of organization size.
Taking the Next Step with Intelligent Automation Solutions
If you’re ready to transform your business communications with the power of intelligent automation, Callin.io offers the perfect entry point into this revolutionary technology. Their platform provides AI-powered phone agents capable of handling inbound and outbound calls autonomously, managing appointments, answering frequently asked questions, and even closing sales with natural, human-like conversations.
Getting started is remarkably straightforward with Callin.io’s free account option, which includes an intuitive interface for configuring your AI agent, test calls to experience the technology firsthand, and a comprehensive task dashboard to monitor all interactions. For businesses requiring advanced functionality like Google Calendar integration and built-in CRM capabilities, premium plans start at just $30 per month.
By implementing Callin.io’s intelligent automation solution, you’ll join forward-thinking companies that have dramatically reduced operational costs while improving customer satisfaction through consistent, 24/7 service availability. Don’t let your business fall behind in the automation revolution—visit Callin.io today to discover how intelligent call center automation can create lasting competitive advantages for your organization.
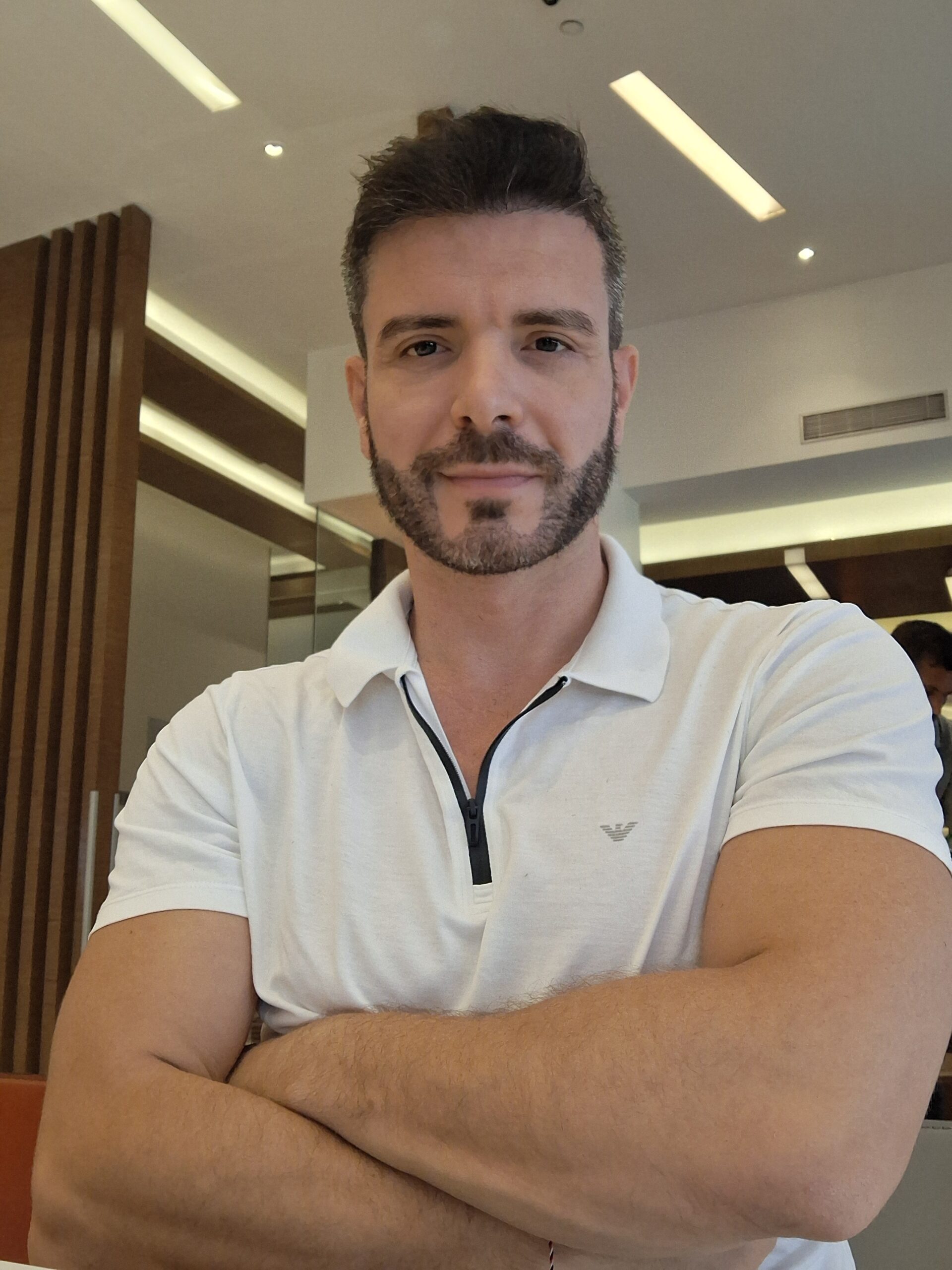
Helping businesses grow faster with AI. 🚀 At Callin.io, we make it easy for companies close more deals, engage customers more effectively, and scale their growth with smart AI voice assistants. Ready to transform your business with AI? 📅 Let’s talk!
Vincenzo Piccolo
Chief Executive Officer and Co Founder