Understanding AI Conversational Training
The intersection of artificial intelligence and human conversation has created a fascinating new employment category: AI Train Conversation Jobs. These specialized positions focus on teaching AI systems how to interact naturally and effectively with humans, bridging the gap between technological capability and human-like communication. According to recent research from Stanford’s Human-Centered AI Institute, conversational AI training has emerged as one of the fastest-growing specializations in the tech industry, with market demand increasing by over 300% since 2020. This field represents a unique blend of linguistics, psychology, data science, and machine learning, making it an attractive career path for professionals from diverse backgrounds. The core responsibility involves developing training datasets and methodologies to help AI voice assistants interact more naturally in customer service, healthcare, education, and numerous other applications.
The Rise of AI Conversation Specialists
The demand for AI conversation training professionals has skyrocketed as more businesses implement conversational AI solutions across their operations. These specialists are responsible for teaching AI systems to understand context, detect emotional nuances, and respond appropriately to human queries. A report by Gartner predicts that by 2025, over 75% of customer service interactions will be handled by AI-driven conversation systems, creating substantial employment opportunities in this field. Companies like Google, Amazon, and Microsoft now maintain dedicated teams of conversational AI trainers to continuously improve their virtual assistants. This professional category includes roles such as conversation designers, dialog flow specialists, voice user interface designers, and AI training data specialists. The AI phone service industry in particular has seen tremendous growth, with specialized training required to make these systems sound natural and responsive.
Essential Skills for AI Conversation Trainers
Becoming proficient in AI conversation training requires a unique skill set that combines technical knowledge with strong communication abilities. Linguistic expertise stands at the forefront, as trainers must understand language nuances, regional dialects, and conversational patterns. Additionally, a solid foundation in machine learning concepts helps trainers comprehend how AI systems process and learn from conversational data. According to the World Economic Forum’s Future of Jobs Report, skills in natural language processing, data annotation, and conversational design are among the most valued competencies for AI training professionals. Many successful AI conversation trainers also bring expertise from fields like psychology, sociolinguistics, or customer service, providing valuable insights into human communication patterns. For those interested in starting an AI calling agency, these skills form the foundation of creating effective AI conversation systems.
Training Methodologies for Conversational AI
Developing effective training methodologies for conversational AI systems involves several sophisticated approaches. Supervised learning remains the cornerstone, where trainers provide labeled conversation examples that demonstrate ideal interactions. However, more advanced systems now incorporate reinforcement learning from human feedback (RLHF), allowing AI to improve through direct user interactions and ratings. This approach has revolutionized how AI call assistants learn and adapt. Another crucial methodology is adversarial training, where systems are intentionally presented with challenging or ambiguous queries to improve resilience and response accuracy. Companies like Twilio have developed specialized frameworks for conversation training that incorporate these approaches. Many leading AI labs supplement these methods with extensive human evaluation panels that provide qualitative feedback on conversation naturalness and appropriateness.
The Role of Prompt Engineering in AI Communication
Prompt engineering has emerged as a critical discipline within AI conversation training, focusing on crafting optimal instructions that guide AI systems toward desired conversation outcomes. This specialized skill involves designing prompts that elicit accurate, helpful, and contextually appropriate responses from large language models. A well-crafted prompt can dramatically improve an AI’s conversational abilities without requiring additional model training. The Journal of Artificial Intelligence Research notes that effective prompt engineering can improve AI response accuracy by up to 40% in complex conversations. Professionals in this field develop expertise in prompt engineering for AI callers and similar applications, creating structured frameworks that help AI systems maintain conversation coherence across various scenarios. This expertise is particularly valuable for businesses implementing AI call center solutions where consistent, high-quality interactions are essential.
Industry Applications of Trained Conversational AI
Trained conversational AI systems are transforming operations across numerous industries with their ability to handle complex customer interactions. In healthcare, conversational AI for medical offices has revolutionized appointment scheduling, prescription renewals, and basic patient triage. The retail sector leverages these technologies for personalized shopping assistance and reducing cart abandonment rates. Financial institutions employ conversational AI for account inquiries, fraud detection, and basic financial advisory services. Real estate agencies utilize AI calling agents to manage property inquiries and schedule viewings efficiently. The hospitality industry has implemented these systems for reservation management and concierge services. Each application requires specialized conversation training to ensure the AI understands industry terminology, common customer concerns, and appropriate response protocols for that specific domain.
The Economics of AI Conversation Training
The financial aspects of AI conversation training present both opportunities and challenges for organizations implementing these systems. While the initial investment in developing and training conversational AI can be substantial, the long-term cost benefits are compelling. Research from Deloitte indicates that properly trained AI voice agents can reduce customer service operational costs by up to 70% while maintaining or improving service quality. The market for AI conversation training services is projected to reach $12 billion by 2026, according to Markets and Markets research. Companies must balance development costs with expected returns on investment, considering factors such as reduced staffing needs, improved customer satisfaction, and increased operational efficiency. For many businesses, partnering with specialized providers like Callin.io offers a cost-effective alternative to building in-house conversation training capabilities, allowing them to leverage pre-trained systems that can be customized for specific business needs.
Ethical Considerations in AI Conversation Training
Training AI conversational systems raises important ethical considerations that professionals in this field must address. Bias mitigation represents one of the most pressing concerns, as conversational AI can inadvertently perpetuate or amplify societal biases present in training data. Trainers must implement rigorous protocols to identify and eliminate biased language patterns and ensure equitable responses across different demographic groups. Privacy protection during the training process is equally critical, particularly when using real conversation data to improve systems. The IEEE Global Initiative on Ethics of Autonomous and Intelligent Systems provides frameworks for ethical AI development that many conversation trainers follow. Transparency about AI identity is another key ethical consideration—users should always understand when they’re interacting with an AI phone agent rather than a human. Organizations implementing these systems must establish clear ethical guidelines for their conversational AI training programs.
The Human Element in AI Conversation Training
Despite advances in automated learning techniques, the human element remains irreplaceable in training effective conversational AI. Human trainers bring cultural context, emotional intelligence, and ethical judgment that purely algorithmic approaches cannot replicate. These professionals provide crucial feedback on conversation quality, helping systems understand subtle aspects of human interaction like humor, empathy, and cultural references. According to research published in Nature Machine Intelligence, AI systems trained with significant human feedback demonstrate 60% higher user satisfaction scores compared to those trained through fully automated methods. The most successful implementations of AI voice conversations maintain this human-in-the-loop approach even after initial deployment, continuously incorporating human feedback to refine responses and adapt to changing communication norms. This collaborative approach between human trainers and AI systems represents the gold standard for developing natural, effective conversational interfaces.
Career Opportunities in AI Conversation Training
The expansion of conversational AI across industries has created diverse career opportunities for those interested in this field. Conversation designers focus on creating natural dialog flows and response patterns, while data annotators label and categorize conversation datasets to improve AI understanding. Voice user interface specialists work specifically on speech-based interactions for AI phone calls and similar applications. AI ethics specialists ensure conversational systems meet ethical standards and comply with regulations. According to LinkedIn’s Emerging Jobs Report, roles in AI conversation training have seen a 74% annual growth rate. Salaries in this field are competitive, with median compensation ranging from $85,000 for entry-level positions to over $150,000 for senior roles with specialized expertise. Many professionals enter this field from backgrounds in linguistics, psychology, data science, or customer experience, bringing valuable perspective to conversation training teams.
Training for Real-World Variability
One of the most significant challenges in AI conversation training involves preparing systems to handle the tremendous variability found in real-world conversations. Human communication is characterized by interruptions, topic shifts, slang, accents, and emotional variations that can confuse AI systems trained on idealized conversation examples. Robust AI training requires exposing systems to this diversity through carefully curated datasets that include examples of disfluencies, regional expressions, and conversational repairs. Companies like Bland AI have developed specialized training methodologies that incorporate realistic conversation variations. Testing in real-world environments is essential, often involving beta deployments where AI phone numbers handle actual customer interactions under supervision. This approach allows trainers to identify and address gaps in the AI’s conversational abilities before full deployment, ensuring the system can navigate the complexities of natural human communication effectively.
Measuring Conversational AI Quality
Establishing objective metrics for conversational AI performance presents unique challenges compared to other AI applications. While technical metrics like word error rate and response latency provide valuable information, they fail to capture the subjective experience of conversation quality. Comprehensive evaluation frameworks typically combine quantitative measures (task completion rates, conversation length) with qualitative assessments from human evaluators. The widely-used ACUTE-Eval methodology, developed by Facebook AI Research, helps quantify conversation naturalness and engagement through comparative human judgments. Organizations implementing call center voice AI solutions often track metrics like customer satisfaction scores, resolution rates, and escalation frequency to gauge system effectiveness. Ongoing evaluation is crucial, as conversational patterns and expectations evolve over time, requiring continuous refinement of both the evaluation metrics and the AI systems themselves.
Multimodal Conversation Training
The frontier of AI conversation training is expanding beyond text and voice to include multimodal communication that incorporates visual cues, gestures, and other non-verbal elements. This approach trains AI systems to understand and respond to the full spectrum of human communication channels, creating more natural and effective interactions. Research from MIT’s Media Lab demonstrates that multimodal systems can achieve up to 35% higher accuracy in understanding user intent compared to audio-only systems. Applications include video-based customer service, healthcare consultations, and educational tutoring where visual cues provide crucial context. Systems like Retell AI are pioneering these integrated approaches. Training for multimodal conversation requires specialized datasets that synchronize audio, text transcriptions, and visual information, presenting both technical challenges and exciting opportunities for creating more human-like AI conversational experiences.
Domain-Specific Conversation Training
The most effective AI conversation systems receive specialized training for their intended application domains, rather than relying solely on general conversational abilities. Domain-specific training involves teaching AI systems industry terminology, common scenarios, and appropriate response protocols for particular fields. For example, AI sales representatives require training on product knowledge, objection handling, and sales methodologies, while AI appointment schedulers must understand scheduling protocols and confirmation processes. This specialization dramatically improves performance in targeted applications, with domain-trained systems showing 3-4 times higher task completion rates compared to general conversational AI. Organizations often build these capabilities by fine-tuning large foundation models with carefully curated domain-specific conversations and knowledge bases. Companies like SynthFlow AI specialize in creating these customized conversation models for specific industries.
Cross-Cultural Conversation Training
As conversational AI systems deploy globally, cross-cultural training has become essential for ensuring effective communication across diverse linguistic and cultural contexts. Training AI to understand cultural references, communication styles, and regional expressions requires specialized datasets and cultural expertise. According to research from the International Journal of Human-Computer Studies, users report 45% higher satisfaction when interacting with AI systems trained to match their cultural communication patterns. Organizations implementing international conversational AI must consider factors like directness versus indirectness, formality levels, and culture-specific politeness norms. Specialized voice models, such as the German AI voice for German-speaking markets, provide authenticity for specific languages. Effective cross-cultural training often involves collaboration with cultural consultants and native speakers to ensure appropriate and respectful AI interactions across diverse global markets.
The Evolution of Voice Synthesis in AI Conversation
Voice quality significantly impacts user perception of conversational AI systems, making advanced voice synthesis a crucial component of the training process. Modern approaches have progressed beyond robotic-sounding speech to create remarkably natural voices with appropriate prosody, emotional inflection, and conversational rhythm. Technologies like ElevenLabs and Play.ht have revolutionized text-to-speech capabilities, enabling more engaging AI voice conversations. The comprehensive guide to voice synthesis technology explores these advances in detail. Training conversational AI now includes voice customization for brand alignment, with organizations selecting voices that reflect their brand personality and target audience preferences. Voice synthesis must also account for timing factors like appropriate pauses and speech rate variations that mirror human conversation patterns, creating a more natural and engaging interaction experience for users.
Implementing Trained AI in Call Centers
The integration of trained conversational AI into call center operations represents one of the most significant applications of this technology. Modern AI call center companies deploy sophisticated systems that can handle tier-1 customer inquiries, route calls intelligently, and provide agents with real-time assistance during complex interactions. The implementation process typically begins with analyzing existing call data to identify common customer needs and appropriate AI use cases. Organizations must then select the right technological foundation, with options ranging from Twilio AI solutions to white-label AI call center platforms that can be customized with company branding. Successful implementations involve extensive testing, agent training, and careful integration with existing CRM and knowledge management systems. Change management represents a critical success factor, as organizations must help both customers and employees adapt to new interaction models that combine AI and human expertise.
Future Trends in AI Conversation Training
The future of AI conversation training points toward increasingly sophisticated systems with enhanced capabilities for natural, contextual interaction. Multimodal understanding will expand as systems learn to process and respond to combinations of text, voice, images, and video simultaneously. Emotional intelligence in AI conversations will improve through advanced training in sentiment analysis and appropriate emotional responses. According to projections from Gartner Research, by 2026, conversational AI will handle up to 85% of customer service interactions in leading organizations. The development of specialized conversation models like Cartesia AI and similar systems will accelerate, creating purpose-built conversational capabilities for specific industry applications. The increasing availability of white-label AI bot solutions will democratize access to these technologies, allowing smaller organizations to implement sophisticated conversational AI without extensive in-house expertise. Training methodologies will continue to evolve, with greater emphasis on reinforcement learning from real-world interactions.
The Business Case for AI Conversation Jobs
The economic rationale for investing in AI conversation training positions continues to strengthen as organizations recognize the substantial ROI these specialists can generate. A well-trained conversational AI system can reduce operational costs while improving customer satisfaction and enabling 24/7 service availability. According to data from Forrester Research, businesses implementing properly trained conversational AI report average cost reductions of 30-50% in customer service operations while simultaneously improving response times by over 60%. The AI for call centers sector demonstrates particularly compelling economics, with trained systems handling routine inquiries at a fraction of human agent costs. Organizations increasingly view conversation training specialists not as technology expenses but as strategic investments that drive competitive advantage through enhanced customer experience. For businesses considering these investments, creating an LLM with specialized conversational capabilities represents an approach with substantial potential returns.
Technical Infrastructure for Conversation Training
Building effective AI conversation systems requires robust technical infrastructure specifically designed for this specialized task. Training environments must support efficient processing of massive conversation datasets while enabling rapid experimentation with different training approaches. Many organizations leverage cloud-based platforms that provide scalable computing resources and specialized AI tools. The technical stack typically includes data annotation tools, natural language understanding components, dialog management systems, and voice processing technologies. Reliable SIP trunking forms the foundation for AI phone agents that need to connect with existing telecommunications infrastructure. Organizations must select appropriate SIP trunking providers that offer the reliability and call quality necessary for AI voice applications. For larger implementations, custom infrastructure with dedicated processing capabilities may be necessary to handle the computational demands of training sophisticated conversation models with millions of parameters and diverse training examples.
Harnessing AI Conversation for Business Growth
The strategic implementation of trained conversational AI offers transformative potential for businesses seeking growth and competitive advantage. Organizations that successfully deploy these systems can significantly enhance customer experiences while reducing operational costs. Proactive engagement represents one powerful application, with systems like AI cold callers reaching out to prospects with personalized conversations that generate qualified leads. Customer service operations benefit from AI call answering services that provide immediate, consistent responses regardless of call volume or time of day. Sales processes can incorporate AI sales pitch generators that create customized presentations based on customer profiles and needs. For entrepreneurs exploring this space, guides on how to start an AI calling business provide valuable insights into market entry strategies. The most successful implementations integrate conversational AI throughout the customer journey, creating seamless experiences that combine the efficiency of automation with thoughtfully designed human touchpoints.
Transform Your Business Communication with Intelligent AI Conversation
As we’ve explored throughout this article, AI train conversation jobs represent a growing opportunity in a rapidly evolving technological landscape. If you’re ready to implement these powerful communication tools in your own organization, Callin.io offers an ideal starting point. Our platform allows you to easily deploy AI phone agents that can handle incoming and outgoing calls autonomously. With Callin’s innovative AI phone agents, you can automate appointment setting, answer frequently asked questions, and even close sales through natural-sounding conversations with your customers.
Getting started with Callin.io is simple with our free account option, which includes an intuitive interface for configuring your AI agent, trial calls, and access to our task dashboard for monitoring interactions. For businesses needing advanced capabilities like Google Calendar integration and built-in CRM functionality, our subscription plans start at just $30 USD monthly. Discover how AI conversation technology can transform your business operations today by visiting Callin.io.
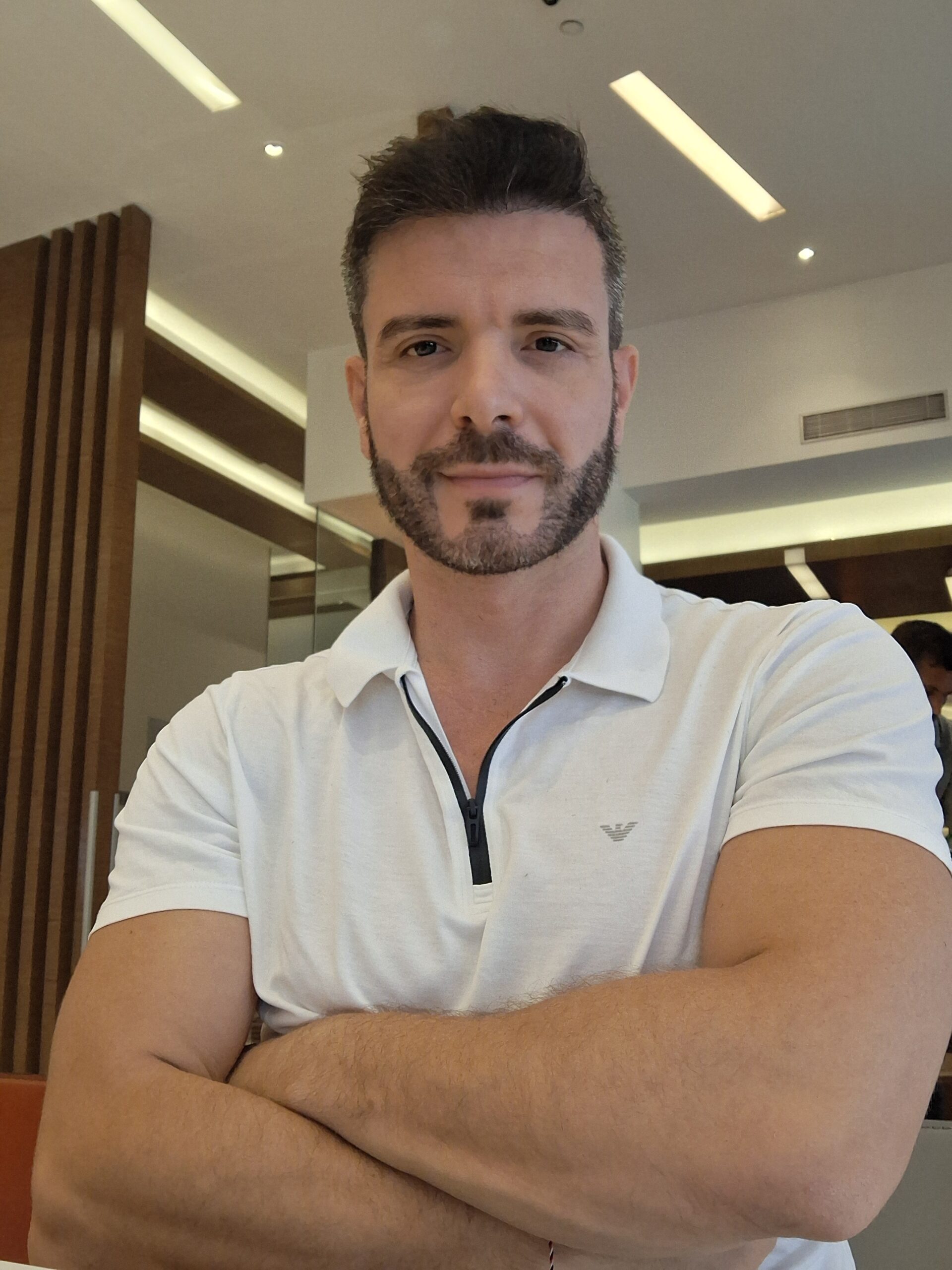
Helping businesses grow faster with AI. 🚀 At Callin.io, we make it easy for companies close more deals, engage customers more effectively, and scale their growth with smart AI voice assistants. Ready to transform your business with AI? 📅 Let’s talk!
Vincenzo Piccolo
Chief Executive Officer and Co Founder