The New Frontier of Risk Management
Risk assessment has radically changed over the past decade, with artificial intelligence now standing at the forefront of this transformation. Companies across industries are abandoning traditional spreadsheet-based analysis in favor of sophisticated AI-powered risk intelligence systems that can process vast datasets in seconds. These technologies don’t just identify potential threats—they anticipate them through predictive analytics and pattern recognition capabilities that human analysts simply cannot match. According to research from Deloitte’s Risk Advisory practice, organizations implementing AI-driven risk assessment solutions report a 60% improvement in threat detection speed and a 45% reduction in false positives compared to conventional methods. This shift isn’t merely technological—it represents a fundamental reimagining of how businesses approach uncertainty in increasingly complex operating environments.
Understanding AI’s Role in Risk Identification
AI excels at spotting hidden connections and subtle patterns that might escape human observation during risk identification processes. Through natural language processing and machine learning algorithms, these systems can continuously scan internal databases, social media, news outlets, and regulatory updates to identify emerging threats before they materialize into crises. For example, financial institutions now employ sentiment analysis to monitor public perception shifts that could signal reputational risks, while manufacturing companies use computer vision to detect safety hazards in production facilities. The real breakthrough comes from AI’s ability to learn from each assessment, continuously refining its risk identification capabilities without explicit reprogramming. This self-improvement cycle enables organizations to build increasingly sophisticated risk profiles that adapt to changing business landscapes in real-time rather than waiting for quarterly review cycles.
Predictive Analytics: Forecasting Threats Before They Emerge
The true power of AI in risk assessment lies in its predictive capabilities. Unlike traditional methods that primarily review historical data, AI systems leverage advanced algorithms to forecast potential future scenarios based on current indicators. These solutions can simulate thousands of variables simultaneously, creating complex risk models that account for the interconnected nature of modern business challenges. A pharmaceutical company implementing AI predictive analytics might identify supply chain vulnerabilities months before they impact production by analyzing weather patterns, geopolitical developments, and transportation data. Similarly, insurance companies now deploy machine learning to predict claim frequencies with remarkable accuracy by processing customer behavior patterns alongside environmental risk factors. According to a McKinsey Global Institute study, organizations that adopt predictive risk technologies see a 30-50% reduction in unexpected losses across their operations.
Real-Time Monitoring and Continuous Assessment
Traditional risk assessment typically follows a periodic schedule—quarterly reviews, annual audits, or planned assessments. AI solutions have shattered this paradigm by enabling continuous monitoring and real-time risk evaluation. Modern systems use streaming analytics to process information as it becomes available, constantly reassessing threat levels and alerting management to emerging issues without delay. A retail bank implementing these technologies might receive instant alerts when unusual transaction patterns emerge across its network, potentially signaling fraud attempts. Manufacturing operations benefit from sensors connected to AI platforms that continuously evaluate equipment performance, predicting potential failures before they cause costly downtime. This shift from point-in-time assessment to persistent vigilance represents one of the most significant advances in risk management practice, allowing businesses to respond to threats within minutes rather than days or weeks after discovery.
Enhancing Decision-Making with AI Risk Insights
AI doesn’t just identify risks—it transforms how organizations make decisions based on risk intelligence. Through intuitive dashboards and automated reporting, these systems present complex risk data in accessible formats that enable faster, more informed choices. Executives receive personalized risk briefs tailored to their specific responsibilities, while operational teams get granular insights relevant to daily activities. For instance, a call center using AI voice analytics might automatically flag compliance risks in customer conversations, allowing supervisors to intervene before regulatory violations occur. Construction companies utilize AI-powered systems that analyze weather forecasts, material deliveries, and workforce availability to calculate project delay risks, enabling proactive schedule adjustments. The true transformation comes from AI’s ability to quantify previously subjective risk factors, providing numerical probabilities that allow for more precise cost-benefit analyses when evaluating business opportunities.
Financial Risk Assessment Revolution
The financial sector has embraced AI risk solutions with particular enthusiasm, developing sophisticated systems that analyze market conditions, transaction patterns, and customer behaviors simultaneously. These platforms can detect potential fraud in milliseconds by comparing transactions against evolving behavioral models that learn from millions of examples. Credit risk assessment has been similarly transformed, with AI systems evaluating traditional metrics alongside non-traditional data points like social media activity and purchasing patterns to create more accurate default probability projections. Investment firms deploy natural language processing to analyze earnings calls, regulatory filings, and news reports, identifying sentiment shifts that might signal market movements. According to the Financial Stability Board, financial institutions implementing comprehensive AI risk frameworks report 40% fewer unexpected losses and 25% lower operational costs compared to competitors relying on conventional methods.
Cybersecurity Enhanced by AI Risk Assessment
The cybersecurity landscape has been permanently altered by AI-powered risk assessment tools that can identify vulnerabilities, detect anomalies, and respond to threats faster than human security teams. These systems establish baseline patterns of normal network behavior, then continuously monitor for deviations that might indicate breaches. Beyond simple rule-based detection, modern solutions use unsupervised learning to discover novel attack vectors that haven’t been previously documented. Healthcare organizations now employ AI cybersecurity platforms that analyze access patterns to detect potential patient data breaches before information leaves their networks. Financial services companies use behavioral biometrics—analyzing how users interact with devices—to spot account takeover attempts even when credentials appear legitimate. The integration of AI with traditional security information and event management (SIEM) platforms creates comprehensive threat intelligence systems that can correlate seemingly unrelated events across vast digital ecosystems, revealing coordinated attacks that might otherwise remain hidden.
Supply Chain Risk Mitigation Through Intelligence
Supply chain disruptions represent one of the most significant threats to modern business operations, and AI has emerged as the most effective tool for identifying vulnerabilities before they impact production. These systems monitor global events, transportation networks, supplier health metrics, and demand patterns simultaneously, creating comprehensive risk profiles for every link in the chain. A manufacturing company might receive automated alerts when political instability threatens a key supplier’s region, enabling procurement teams to source alternatives before shortages occur. Retail operations leverage AI voice agents to contact suppliers, verify shipment status, and identify potential delays through conversation analysis. The most advanced implementations create digital twins—virtual replicas of physical supply chains—that allow for scenario planning and stress testing without real-world disruption. According to research from the MIT Center for Transportation & Logistics, organizations implementing AI-driven supply chain risk management report 35% fewer disruptions and 28% faster recovery times when incidents do occur.
Regulatory Compliance and Risk Assessment
Navigating complex regulatory environments presents significant challenges for businesses across sectors, but AI solutions now offer powerful tools for compliance risk management. These systems continuously monitor regulatory changes across jurisdictions, automatically flagging new requirements relevant to specific business operations. Financial institutions deploy natural language processing to analyze thousands of pages of new regulations daily, extracting actionable compliance requirements without human review. Healthcare organizations use AI appointment schedulers and communication systems that automatically ensure patient interactions remain HIPAA-compliant while documenting consent. Beyond simple monitoring, advanced implementations can simulate regulatory examinations, identifying potential compliance gaps before actual audits occur. The most sophisticated solutions incorporate voice analytics to evaluate call center interactions, flagging potential compliance violations in real-time and enabling immediate intervention. According to Thomson Reuters’ Cost of Compliance Report, organizations utilizing AI compliance tools report 52% lower regulatory penalties and 40% reduced compliance staffing needs compared to manual approaches.
Human Resources Risk Intelligence
The human element remains a critical factor in organizational risk profiles, and AI now plays a crucial role in workforce risk assessment. These systems analyze employee data, communication patterns, and performance metrics to identify potential issues before they impact operations. Healthcare facilities use sentiment analysis to monitor staff communications for signs of burnout that might lead to patient care risks. Financial institutions deploy AI voice assistants that analyze trader conversations for potential policy violations or inappropriate behaviors. Beyond individual monitoring, these solutions provide workforce-wide insights that help organizations identify departments with elevated turnover risk or teams experiencing communication breakdowns that might compromise project delivery. The most advanced implementations incorporate predictive analytics to forecast staffing requirements, reducing the risk of critical skill shortages during peak business periods. According to research from HR analytics firm Visier, organizations implementing comprehensive workforce risk intelligence report 38% lower unexpected turnover and 42% fewer workplace incidents compared to those relying on traditional HR monitoring approaches.
Reputational Risk Management in the Digital Age
In today’s hyperconnected environment, reputational damage can spread instantly through social media and digital channels, making AI-powered reputation monitoring essential for risk management. These systems continuously scan online platforms, news outlets, and customer feedback channels to identify potential threats to brand perception. Retail companies deploy sentiment analysis to evaluate thousands of customer reviews daily, flagging emerging product issues before they generate widespread negative publicity. Financial institutions use AI phone services to monitor call center interactions for customer dissatisfaction that might lead to public complaints. The most sophisticated implementations incorporate predictive analytics to forecast how specific incidents might impact brand value, enabling more informed crisis management decisions. Beyond monitoring, these systems can automatically generate response recommendations based on successful past interventions, ensuring consistency in reputation management across the organization. According to the Reputation Institute, companies using AI-powered reputation risk management recover from negative incidents 60% faster and experience 45% less severe financial impacts compared to those relying solely on human monitoring.
Environmental and Sustainability Risk Assessment
Environmental risks have moved from peripheral concerns to central business considerations, with AI offering unprecedented capabilities for sustainability risk assessment. These systems integrate climate data, regulatory requirements, and operational metrics to create comprehensive environmental risk profiles. Energy companies now deploy satellite imagery analysis powered by machine learning to monitor facilities for potential environmental compliance issues. Manufacturing operations use AI-powered sensors to detect subtle changes in emissions patterns that might indicate equipment failures before they cause regulatory violations. Beyond compliance, these solutions help organizations understand how climate change might impact their operations, identifying facilities at increasing flood risk or supply chains vulnerable to water scarcity. The most advanced implementations create scenario models that simulate how different sustainability initiatives might affect both environmental impact and financial performance, enabling more strategic resource allocation. According to CDP (formerly Carbon Disclosure Project), organizations implementing AI-driven environmental risk assessment report 55% fewer unexpected compliance issues and 30% lower environmental remediation costs compared to traditional approaches.
Integrating AI Risk Assessment with Business Strategy
The true potential of AI risk assessment emerges when these tools move beyond threat identification to become integral components of strategic planning. Forward-thinking organizations now incorporate risk intelligence directly into decision-making frameworks, creating a continuous feedback loop between risk assessment and strategic initiatives. Retail companies leverage AI sales analytics to evaluate market expansion opportunities against potential competitive and economic risks, optimizing site selection based on quantified risk-reward calculations. Healthcare systems use predictive risk modeling to evaluate potential service line expansions, forecasting both patient demand and potential regulatory challenges before committing resources. The most sophisticated implementations create integrated planning environments where executives can instantly see how potential decisions might affect risk profiles across the organization, enabling more nuanced strategy development. According to Boston Consulting Group research, companies that fully integrate risk intelligence into strategic planning outperform peers by 25% in long-term shareholder returns while experiencing 40% fewer strategic initiative failures.
Ethical Considerations in AI-Driven Risk Assessment
While AI offers powerful risk management capabilities, these systems introduce their own ethical considerations that organizations must address. Algorithmic bias represents a significant concern, as risk assessment models trained on historical data might perpetuate existing prejudices or create new forms of discrimination. Financial institutions must ensure that AI credit risk models don’t disadvantage certain demographic groups based on correlations rather than causation. Healthcare organizations need transparency in how AI systems flag patient risk factors to prevent reinforcing existing healthcare disparities. Beyond bias, privacy considerations require careful attention, as risk assessment often involves analyzing sensitive personal or proprietary information. Organizations implementing these technologies should establish clear governance frameworks that define appropriate data usage, algorithmic transparency requirements, and human oversight protocols. The most responsible implementations incorporate regular ethical audits that evaluate how AI risk assessments impact various stakeholders, ensuring these powerful tools enhance rather than undermine organizational values. According to the AI Now Institute, organizations that implement comprehensive ethical frameworks for AI risk assessment experience 65% fewer reputational incidents related to algorithm deployment compared to those without formal ethical guidelines.
Case Study: Financial Services Risk Transformation
The banking sector provides compelling examples of AI risk assessment’s transformative impact. A leading global bank implemented an integrated AI risk platform that analyzes transaction patterns, customer interactions, and external threat intelligence simultaneously. The system processes over 5 million transactions daily, evaluating each against evolving fraud models that adapt to new criminal tactics without manual updates. Voice analysis technology examines customer service calls for potential social engineering attempts, flagging suspicious interactions for security team review. Beyond fraud prevention, the platform monitors global economic indicators, regulatory announcements, and competitor activities to create comprehensive strategic risk assessments for executive leadership. This implementation reduced fraud losses by 72% while simultaneously decreasing false positive rates by 65%, improving both security and customer experience. Perhaps most significantly, the bank reports 40% faster time-to-market for new products due to streamlined risk evaluation processes that can assess compliance implications in hours rather than weeks. The organization estimates its $15 million investment in AI risk technologies generates over $120 million in annual value through loss prevention, efficiency gains, and accelerated innovation.
Case Study: Manufacturing Risk Intelligence
A multinational manufacturing company demonstrates how AI risk assessment transforms industrial operations. The organization implemented a comprehensive solution that integrates production data, supply chain monitoring, and facility sensors into a unified risk intelligence platform. Machine learning algorithms analyze equipment performance patterns to predict potential failures before they cause production interruptions, reducing unplanned downtime by 58% across global operations. AI phone agents automatically contact suppliers when order patterns deviate from predictions, verifying delivery timelines without human intervention. Environmental sensors connected to the platform monitor emissions and waste management systems, alerting environmental compliance teams to potential regulatory issues before violations occur. Beyond operational concerns, the system analyzes geopolitical developments, currency fluctuations, and commodity price trends to create market risk assessments for each product category. This implementation reduced lost production time by 42% while simultaneously decreasing quality incidents by 35% through earlier intervention in potential manufacturing issues. The company estimates its AI risk platform generates $85 million in annual value through improved operational efficiency, reduced compliance penalties, and enhanced supply chain reliability.
Implementation Challenges and Success Factors
Despite clear benefits, implementing AI risk assessment solutions presents significant challenges that organizations must address for successful deployment. Data quality stands as perhaps the most critical factor, as even sophisticated algorithms produce misleading results when trained on incomplete or inaccurate information. Organizations should conduct thorough data readiness assessments before implementation, identifying gaps in collection processes and establishing data governance frameworks that ensure ongoing integrity. Cultural resistance often emerges as executives accustomed to intuition-based decision making question algorithmic recommendations. Successful implementations typically include comprehensive change management programs with executive training on AI capabilities and limitations. Technical integration challenges arise when connecting risk platforms with existing enterprise systems, requiring careful planning and potentially phased implementations. Organizations that achieve the greatest success typically establish clear ownership of AI risk initiatives, whether through dedicated teams or by empowering existing risk functions with technical resources. According to Gartner research, implementations that include formal data governance, executive sponsorship, and dedicated AI oversight achieve positive ROI 3.5 times more frequently than projects lacking these elements.
Future Trends in AI Risk Assessment
The evolution of AI risk assessment continues at a remarkable pace, with several emerging trends poised to further transform organizational security practices. Explainable AI represents a critical development, as newer algorithms provide transparent reasoning behind risk assessments rather than functioning as inscrutable "black boxes." This advancement allows risk managers to understand why specific threats were flagged, enabling more nuanced responses and greater trust in automated recommendations. Federated learning enables organizations to train risk models collaboratively without sharing sensitive data, creating more robust algorithms while maintaining privacy and competitive boundaries. Conversational AI platforms now allow non-technical users to query risk systems through natural language, democratizing access to sophisticated risk intelligence throughout organizations. Quantum computing promises to eventually revolutionize risk modeling by enabling simulations of unprecedented complexity, potentially identifying subtle interaction effects between risk factors that current systems cannot detect. Perhaps most significantly, AI risk assessment increasingly incorporates principles from complex adaptive systems theory, recognizing that modern business environments behave as interconnected ecosystems rather than collections of independent variables. According to Stanford University’s AI Index, organizations implementing these advanced approaches report 70% higher satisfaction with risk management outcomes compared to those using first-generation AI solutions.
Selecting the Right AI Risk Assessment Platform
Choosing appropriate AI risk solutions requires careful evaluation against specific organizational needs rather than simply selecting the most technically advanced options. Organizations should begin by identifying their highest-priority risk domains—whether cybersecurity, compliance, financial stability, or operational reliability—and select solutions with demonstrated strength in these areas. Integration capabilities represent another crucial consideration, as risk assessment provides greatest value when connected to existing enterprise systems like ERP platforms, CRM databases, and communication tools like AI phone systems. Data requirements deserve careful attention, as some solutions demand extensive historical information while others can generate valuable insights from more limited datasets. Smaller organizations might prefer cloud-based solutions that minimize infrastructure requirements, while enterprises with sensitive information may require on-premises deployments for regulatory compliance. Beyond technical considerations, evaluation should include vendor stability assessment, as risk management represents a long-term commitment that depends on ongoing support and development. Organizations should request detailed implementation timelines and resource requirements, as sophisticated platforms often demand significant configuration before delivering value. According to Forrester Research, organizations that conduct formal needs assessments before selecting AI risk platforms report 68% higher satisfaction with implementation outcomes compared to those making decisions based primarily on vendor demonstrations.
Measuring ROI from AI Risk Solutions
Quantifying the return on investment from AI risk assessment requires thoughtful analysis that captures both direct savings and opportunity costs avoided. Organizations should establish baseline metrics before implementation, documenting current expenditures on risk-related incidents, compliance penalties, security breaches, and operational disruptions. Direct cost reductions often appear in areas like fraud prevention, where AI systems may immediately decrease losses through improved detection. Efficiency gains typically emerge through automation of routine risk monitoring tasks, allowing highly skilled analysts to focus on complex assessments rather than data gathering. The most significant returns often come from avoiding major incidents altogether—a manufacturing company that prevents a week-long production shutdown through early risk detection might save millions in lost revenue and recovery costs. Some organizations calculate "decision quality improvement" by comparing outcomes from AI-augmented decisions against historical results from conventional approaches. Comprehensive ROI frameworks should include both financial metrics and operational indicators like reduced cycle times for risk assessments, improved risk detection rates, and decreased false positives that cause unnecessary interventions. According to PwC research, organizations using formal ROI methodologies for AI risk investments achieve 2.3 times greater return on these initiatives compared to those using ad hoc evaluation approaches.
Transforming Your Risk Management Strategy with Callin.io
As you consider enhancing your organization’s risk assessment capabilities, implementing the right communication tools becomes essential for effective risk monitoring and response. Callin.io offers a revolutionary approach to business communications that seamlessly integrates with your risk management strategy. Our AI-powered phone agents can conduct regular check-ins with suppliers to identify potential supply chain risks, contact customers about unusual account activities, and even perform automated regulatory compliance checks—all while maintaining natural, human-like conversations.
The platform’s advanced voice analytics capabilities can identify stress patterns in calls that might indicate emerging risks, while automatically documenting all interactions for compliance purposes. With Callin.io’s AI call center solution, your risk management team receives real-time alerts about potential issues without manually monitoring every conversation. This proactive approach transforms how quickly your organization can respond to emerging threats.
Getting started with Callin.io is straightforward and risk-free. Create your free account today to access our intuitive interface, test calls, and comprehensive dashboard for tracking interactions. For organizations ready to fully integrate AI communications into their risk management framework, our premium plans starting at just $30 per month provide Google Calendar integration, CRM connectivity, and customized risk notification protocols. Discover how Callin.io can strengthen your risk assessment capabilities while streamlining communication processes across your organization.
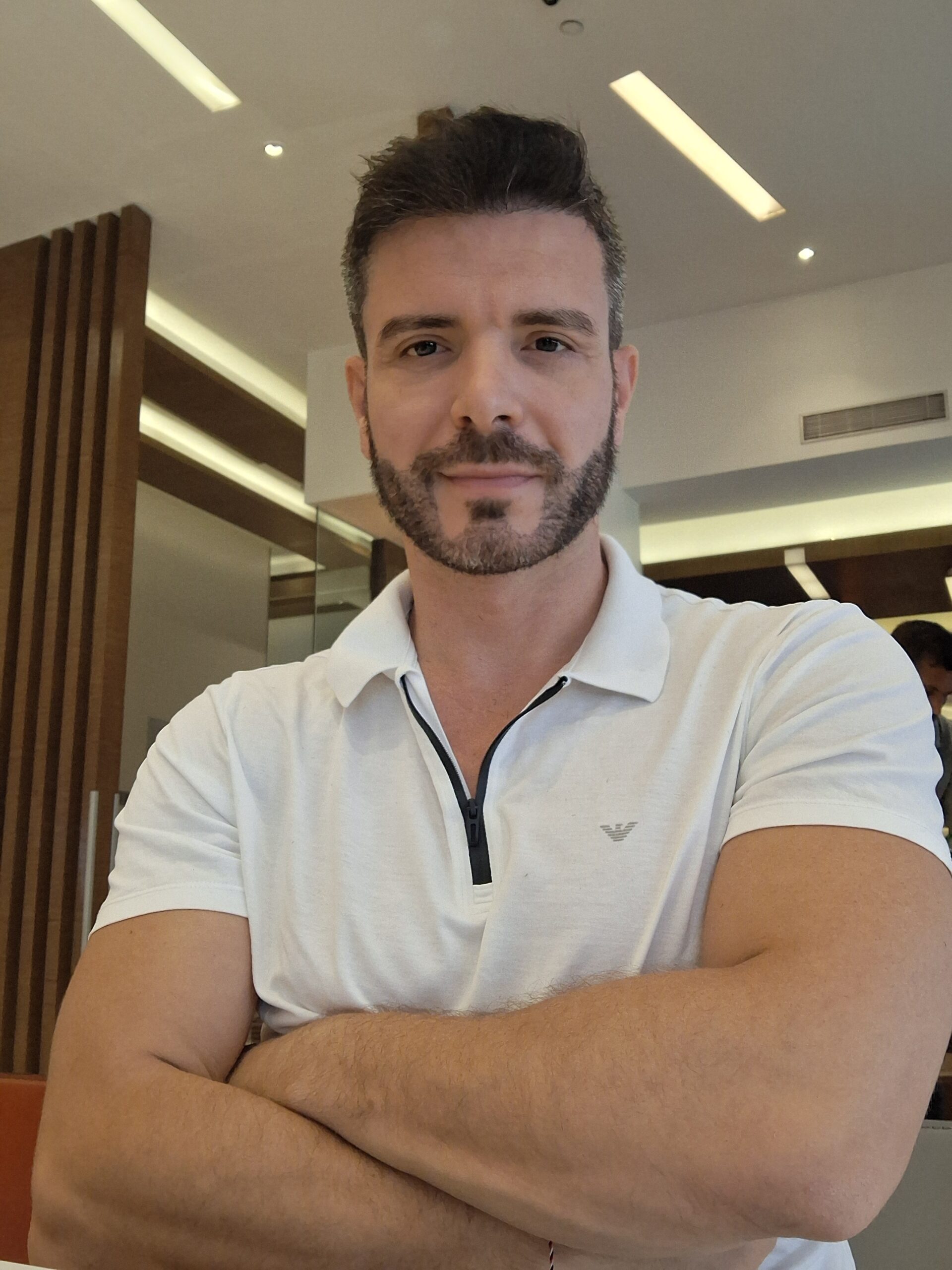
Helping businesses grow faster with AI. 🚀 At Callin.io, we make it easy for companies close more deals, engage customers more effectively, and scale their growth with smart AI voice assistants. Ready to transform your business with AI? 📅 Let’s talk!
Vincenzo Piccolo
Chief Executive Officer and Co Founder