The Genomic Revolution Meets Artificial Intelligence
The intersection of genomics and artificial intelligence represents one of the most groundbreaking developments in biomedical research today. Genomic data has exploded in both volume and complexity since the completion of the Human Genome Project in 2003, creating both unprecedented opportunities and formidable challenges for researchers. AI solutions for genomics are transforming how scientists analyze, interpret, and utilize genetic information to advance medical science. These computational tools can process and make sense of massive datasets that would be impossible for humans to manually analyze, accelerating discoveries that can lead to personalized medicine, novel treatments, and deeper understanding of human biology. As noted by the National Human Genome Research Institute, AI applications in genomics are helping researchers identify patterns within genetic data that human eyes might miss, potentially revolutionizing how we diagnose and treat diseases.
Decoding DNA: The Power of Machine Learning in Sequence Analysis
Machine learning algorithms have become essential tools for genomic sequence analysis, offering unprecedented accuracy and efficiency. These computational approaches excel at recognizing patterns within DNA sequences, identifying genetic variants, and predicting potential impacts of mutations. Deep learning models trained on vast genomic datasets can now identify functional elements within the genome with remarkable precision, including genes, regulatory regions, and structural variations. For example, tools like DeepVariant, developed by Google Brain, use neural networks to identify genetic variants with significantly higher accuracy than traditional methods. This technical advancement fundamentally changes how genetic variation is detected, enabling more precise identification of disease-causing mutations. Conversely, as discussed in a recent study in Nature Reviews Genetics, machine learning approaches are also being used to predict gene expression from DNA sequence alone, providing insights into how genetic information is translated into physical traits or disease predisposition.
Personalizing Medicine Through AI-Driven Genomic Profiles
The promise of AI voice assistants in healthcare extends to personalized medicine based on individual genetic profiles. By analyzing a patient’s genomic data, AI systems can identify genetic markers associated with disease risk, drug response, and treatment outcomes. This personalized approach allows clinicians to tailor treatments to a patient’s specific genetic makeup, potentially improving efficacy and reducing adverse effects. For instance, oncologists are now using AI-powered genomic analysis to identify specific mutations in cancer cells that can guide treatment selection, helping determine which patients might respond to targeted therapies or immunotherapies. Companies like Foundation Medicine and Tempus are pioneering this approach, analyzing tumor genomes and matching patients to clinical trials based on their genetic profiles. This level of personalization represents a significant departure from the traditional "one-size-fits-all" approach to medicine, potentially transforming how we treat complex diseases like cancer, diabetes, and heart disease.
Drug Discovery Reimagined: How AI Accelerates Genetic-Based Therapies
AI solutions are dramatically accelerating drug discovery processes by rapidly analyzing genomic data to identify potential therapeutic targets. Computational drug discovery powered by machine learning can analyze thousands of genetic targets and millions of chemical compounds to predict promising drug candidates, drastically reducing the time and cost of bringing new treatments to patients. Organizations like Recursion Pharmaceuticals are using AI to screen massive libraries of genetic and chemical data to identify novel treatment approaches for rare genetic diseases. Similarly, DeepMind’s AlphaFold has revolutionized protein structure prediction, a critical component in understanding how genetic mutations affect protein function and identifying potential drug targets. This AI-driven approach to drug discovery has particular significance for rare genetic disorders, where traditional drug development approaches often fall short due to limited market incentives and complex biological mechanisms. The integration of conversational AI technologies with genomic platforms is also creating more accessible ways for researchers to interact with complex genetic data.
Clinical Genomics: AI-Powered Diagnosis and Disease Prediction
In clinical settings, AI solutions for genomics are enhancing diagnostic capabilities and enabling early disease prediction. Machine learning algorithms can analyze a patient’s genomic data alongside their medical records to identify patterns associated with disease risk or progression. This approach is particularly valuable for complex genetic conditions where multiple genes may contribute to disease development. For example, companies like Fabric Genomics use AI to interpret whole genome sequences and exomes, helping clinicians identify potential disease-causing variants more quickly and accurately than traditional analysis methods. These tools are especially valuable in neonatal intensive care units, where rapid diagnosis of genetic disorders can be life-saving. As suggested by researchers at Stanford Medicine, AI systems can even analyze facial features alongside genomic data to diagnose rare genetic syndromes with greater accuracy than human specialists. The integration of genomic AI tools with AI call assistance systems could further enhance clinical workflows by facilitating communication between medical professionals regarding complex genetic findings.
Managing Genomic Big Data: Cloud Computing and Distributed Systems
The sheer volume of genomic data presents unprecedented storage and processing challenges that AI solutions are uniquely positioned to address. A single human genome contains approximately 3 billion base pairs, generating roughly 200 gigabytes of raw data—and research institutions often work with thousands or millions of genomes simultaneously. Cloud-based genomic platforms integrated with AI analysis tools offer scalable solutions to these data management challenges. Services like Google Cloud Healthcare API and DNAnexus provide secure, compliant environments for storing and analyzing massive genomic datasets, with built-in AI capabilities for interpretation. These platforms leverage distributed computing to process genomic information in parallel, dramatically reducing analysis time from weeks to hours. The integration of blockchain technology is also emerging as a solution for secure sharing of sensitive genomic data while maintaining patient privacy and data ownership. This infrastructure is crucial for large-scale initiatives like population genomics programs, which aim to sequence millions of individuals to build comprehensive genetic reference databases.
Ethical Implications: AI, Genomic Privacy, and Equity Concerns
The powerful combination of AI and genomics raises significant ethical questions around data privacy, consent, and equitable access to genomic medicine. Genomic information is inherently personal and potentially sensitive, containing insights about an individual’s disease risks, ancestry, and even behavioral tendencies. AI systems that process this data must incorporate robust privacy protections and transparent governance frameworks. As discussed by the Hastings Center, there are growing concerns about potential discriminatory uses of genetic information and the need for strong legal protections. Additionally, most genomic datasets currently overrepresent individuals of European ancestry, potentially creating bias in AI algorithms trained on this data. Researchers and companies must work to diversify genomic databases to ensure that AI-driven genomic medicine benefits all populations equally. Innovative approaches like federated learning, which allows AI models to be trained across multiple institutions without sharing raw data, may help address some privacy concerns while enabling collaborative research.
Population Genomics: AI Tools for Large-Scale Genetic Studies
Population genomics programs are increasingly employing AI tools to analyze genetic variations across large groups, providing unprecedented insights into human genetic diversity, migration patterns, and disease susceptibility. Projects like the UK Biobank, which contains genetic data from 500,000 individuals, rely on machine learning to identify associations between genetic variants and thousands of phenotypic traits. Similarly, the All of Us Research Program in the United States aims to collect genomic data from one million diverse Americans, using AI techniques to derive meaningful patterns from this massive dataset. These large-scale initiatives are uncovering rare genetic variants associated with disease risk that would be invisible in smaller studies, while also revealing the complex interplay between genetics and environmental factors. AI algorithms are essential for identifying subtle patterns within these datasets, such as gene-gene interactions or polygenic risk scores that involve hundreds or thousands of genetic markers. The insights gained from these population-level analyses are creating more accurate reference genomes and improving our understanding of genetic diversity across human populations.
Multi-Omics Integration: Beyond DNA Sequencing
While genomics focuses on DNA sequences, a complete understanding of biological systems requires integration of multiple "omics" data types—proteomics (proteins), transcriptomics (gene expression), metabolomics (metabolites), and epigenomics (chemical modifications to DNA). AI solutions excel at integrating these diverse data types to create comprehensive biological models. Machine learning algorithms can identify complex relationships between different layers of biological information, helping researchers understand how genetic variations ultimately lead to observable traits or disease phenotypes. For instance, researchers at the Broad Institute are using AI to integrate multi-omics data to better understand cancer biology and drug resistance mechanisms. This integrated approach provides a more complete picture of biological processes than genomics alone and has led to discoveries about how genetic variations influence disease through effects on protein function, gene regulation, or metabolic pathways. The computational challenges of multi-omics integration are substantial, requiring sophisticated AI models that can handle heterogeneous data types while accounting for their interrelationships.
Real-Time Genomic Monitoring: From Lab to Clinic
Emerging AI technologies are enabling real-time genomic monitoring applications with profound implications for infectious disease surveillance, cancer treatment, and antibiotic resistance tracking. Portable DNA sequencing technologies, combined with AI analysis, allow genetic material to be analyzed outside traditional lab settings—even in remote locations. During the COVID-19 pandemic, real-time genomic surveillance was crucial for tracking viral variants and guiding public health responses. Similarly, in cancer treatment, liquid biopsies analyzed with AI can detect circulating tumor DNA, allowing oncologists to monitor treatment response and cancer evolution without invasive procedures. This real-time monitoring capability is particularly valuable for tracking emerging infectious diseases, as demonstrated by platforms like Nextstrain, which uses AI to analyze and visualize pathogen evolution as it happens. The integration of genomic monitoring with AI phone services could further enhance communication of important genomic findings to healthcare providers and public health officials, especially during disease outbreaks or pandemic situations.
Microbiome Analysis: AI Decoding Our Microbial Genomes
The human microbiome—the collection of trillions of microbial organisms living in and on our bodies—represents another frontier for AI-powered genomic analysis. These microbial communities significantly influence our health, playing roles in immunity, metabolism, and even mental health. AI tools can analyze complex microbial genomic data to identify patterns associated with disease states or treatment responses. Companies like Viome use machine learning to analyze gut microbiome composition and provide personalized dietary recommendations based on an individual’s unique microbial profile. Researchers at the Human Microbiome Project are employing AI techniques to understand how microbial communities change over time and respond to interventions. The complexity of microbiome data—often involving thousands of microbial species with millions of genes—makes it an ideal application for AI, which can identify subtle patterns and interactions that traditional analysis methods might miss. This emerging field of microbiome genomics, empowered by AI, promises new insights into conditions like inflammatory bowel disease, obesity, and autoimmune disorders.
Functional Genomics: Understanding Gene Function Through AI
Beyond identifying genetic variants, AI is revolutionizing functional genomics—the study of how genes actually operate within living systems. CRISPR gene editing combined with AI analysis enables researchers to systematically perturb genes and observe the effects, helping to decipher gene function at unprecedented scale. Machine learning models trained on large functional genomics datasets can predict how specific genetic changes might affect cellular processes, protein interactions, or phenotypic outcomes. The Connectivity Map project at the Broad Institute uses AI to analyze gene expression patterns in response to thousands of drug compounds and genetic perturbations, helping researchers identify unexpected connections between genes, diseases, and potential treatments. Similarly, the ENCODE project leverages machine learning to identify functional elements within the human genome, including enhancers, promoters, and other regulatory regions. These functional genomics approaches, enhanced by AI, are filling critical gaps in our understanding of how genetic information is translated into biological function—essential knowledge for developing targeted genetic therapies.
Agricultural and Environmental Genomics: AI Beyond Human Health
The applications of AI solutions for genomics extend well beyond human health into agriculture, conservation, and environmental monitoring. In agriculture, machine learning algorithms analyze crop and livestock genomes to identify genetic markers associated with desirable traits like drought resistance, nutritional content, or disease resistance. Companies like Benson Hill use AI-powered genomic tools to accelerate plant breeding programs, developing improved crop varieties without genetic modification. In conservation biology, genomic analysis aided by AI helps identify endangered populations, understand genetic diversity within species, and guide preservation efforts. Environmental genomics, or metagenomics, relies on AI to analyze the collective genetic material in environmental samples, providing insights into ecosystem health and function. These applications demonstrate the versatility of AI-genomic approaches across different biological systems and highlight their potential to address global challenges like food security and biodiversity loss.
Variant Interpretation: Making Sense of Genetic Differences
One of the most significant challenges in genomics is interpreting the clinical significance of the millions of genetic variants that exist in human populations. AI systems are becoming increasingly sophisticated at distinguishing disease-causing mutations from benign genetic differences. Machine learning models trained on databases of known pathogenic variants can predict the potential impact of newly discovered variants with increasing accuracy. Platforms like Varsome and Franklin incorporate AI to help clinicians interpret genetic test results and make informed decisions about patient care. These tools consider multiple lines of evidence—conservation across species, structural changes to proteins, population frequency data—to provide comprehensive variant assessments. The integration of AI call center technologies could further enhance genetic counseling services, helping communicate complex genetic information to patients and families. As our knowledge of genetic variants continues to grow, AI systems can continuously improve their interpretative accuracy, creating a virtuous cycle of better genomic medicine.
Single-Cell Genomics: AI Revealing Cellular Heterogeneity
Traditional genomic analysis typically examines DNA from mixed cell populations, potentially masking important differences between individual cells. Single-cell sequencing technologies now allow researchers to examine the genetic information of thousands of individual cells simultaneously, revealing previously hidden cellular heterogeneity. However, the resulting data is massive and complex, making AI tools essential for meaningful analysis. Machine learning algorithms can identify distinct cell types, developmental trajectories, and rare cell populations within complex tissues. This approach has been particularly valuable in cancer research, where it has revealed unexpected cellular diversity within tumors that may explain treatment resistance and recurrence. Projects like the Human Cell Atlas are creating comprehensive maps of all human cell types, using AI to analyze single-cell genomic data from millions of cells across different tissues and organs. These highly detailed cellular maps are transforming our understanding of human development, aging, and disease processes at unprecedented resolution.
Long-Read Sequencing: AI Tackling Structural Variation
Most genomic sequencing to date has relied on short-read technologies that break DNA into small fragments, making it difficult to detect large structural variations like insertions, deletions, or rearrangements. New long-read sequencing technologies generate continuous DNA sequences of thousands or even millions of base pairs, but produce more complex data that requires sophisticated analysis. AI algorithms are being developed specifically to interpret long-read sequencing data, enabling better detection of structural variants that may contribute to disease. Companies like Pacific Biosciences and Oxford Nanopore Technologies are incorporating machine learning into their analysis workflows to improve accuracy and extract maximum information from long-read data. This technological advancement is particularly important for understanding complex genetic diseases where structural variations play a significant role, such as certain neurological disorders and cancers. The integration of long-read sequencing data with other genomic information presents computational challenges that AI is uniquely positioned to address through its pattern recognition capabilities and ability to handle heterogeneous data types.
Pharmacogenomics: Personalizing Drug Therapy Through AI
Pharmacogenomics—the study of how genetic variations affect drug response—is being transformed by AI solutions that can predict individual responses to medications. Genetic differences can significantly impact how patients metabolize drugs, affecting both efficacy and side effect profiles. Machine learning models trained on large pharmacogenomic datasets can identify genetic markers associated with drug response, helping clinicians select the most appropriate medications and dosages for each patient. The Clinical Pharmacogenetics Implementation Consortium provides guidelines for using genetic information in prescribing, and AI tools are making these guidelines more accessible and actionable for healthcare providers. For example, AI systems can analyze a patient’s genomic data and medication list to flag potential gene-drug interactions or suggest dosage adjustments. This approach has particular relevance for medications with narrow therapeutic windows, such as warfarin, where genetic factors significantly influence optimal dosing. The integration of pharmacogenomic AI with electronic health records and clinical decision support systems promises to make precision prescribing increasingly accessible in everyday medical practice.
Digital Twins: AI-Powered Genomic Simulations
The concept of digital twins—virtual replicas of physical entities—is being applied to genomics through AI-powered simulations of genetic systems. These computational models integrate genomic data with other biological information to create virtual representations of cells, tissues, or even entire physiological systems that can be used to predict responses to perturbations. For example, companies like Unlearn.AI are developing digital twins of patients for clinical trials, incorporating genomic information to improve predictions of disease progression and treatment response. These models can potentially reduce the need for control groups in clinical trials and accelerate the development of personalized treatments. Similarly, researchers are creating digital twins of cancer patients based on their tumor genomics, which can be used to virtually test different treatment regimens before administering them to the actual patient. As these simulation technologies advance, they may enable truly personalized treatment planning based on an individual’s unique genetic profile, potentially improving outcomes while reducing adverse effects.
Future Horizons: Quantum Computing and Synthetic Genomics
Looking ahead, emerging technologies like quantum computing promise to further revolutionize AI applications in genomics. Quantum computers could potentially solve complex genomic problems that remain intractable with classical computing approaches, such as protein folding predictions or comprehensive modeling of gene regulatory networks. Companies like IBM and Google are already exploring quantum applications in computational biology. Meanwhile, synthetic genomics—the design and creation of new DNA sequences—represents another frontier where AI is making significant contributions. Machine learning approaches can design novel genetic sequences with specific properties, potentially enabling creation of synthetic organisms for biomanufacturing, environmental remediation, or medical applications. The combination of AI with technologies like CRISPR gene editing also opens possibilities for precise genetic modifications to treat inherited diseases. These cutting-edge applications demonstrate how AI solutions for genomics continue to push the boundaries of what’s possible in biological science.
Convergence of Voice AI and Genomic Tools: Enhancing Biomedical Research
The integration of AI voice agents with genomic analysis platforms represents an emerging trend that could transform how researchers and clinicians interact with complex genetic data. Voice-activated genomic research assistants could allow scientists to query massive datasets using natural language, making sophisticated genomic analysis more accessible to researchers without extensive computational training. These conversational interfaces could help clinicians quickly access relevant genetic information during patient consultations, enhancing clinical decision-making. For example, a doctor could verbally request information about specific genetic variants identified in a patient’s test results, receiving immediate synthesized insights about their clinical significance. Similarly, researchers could use voice commands to explore genomic databases, compare sequences, or initiate complex analyses without writing code. This convergence of voice AI with genomic tools aligns with broader trends toward more intuitive human-computer interaction in scientific research and could significantly accelerate the translation of genomic insights into clinical practice.
Harnessing Genomic Intelligence for Improved Healthcare
The transformative potential of AI solutions for genomics cannot be overstated. From unraveling the mysteries of our genetic code to delivering personalized medical treatments, these technologies are reshaping healthcare and biological research at a fundamental level. As genomic data continues to grow exponentially, AI approaches will become increasingly essential for extracting meaningful insights that can improve human health and advance scientific understanding. However, realizing the full potential of genomic AI requires thoughtful attention to issues of data privacy, algorithmic transparency, and equitable access. By addressing these challenges proactively, we can ensure that the benefits of AI-powered genomics are broadly shared across diverse populations.
Advancing Your Genomic Research with Intelligent Communication Tools
If you’re working in genomic research or healthcare and looking to enhance your organization’s communication capabilities, Callin.io offers innovative solutions that complement your technical infrastructure. Our AI phone agents can streamline appointment scheduling for genetic counseling services, facilitate communication between research teams, and help disseminate important findings to healthcare providers. Unlike traditional phone systems, Callin.io’s AI agents can understand complex conversations, schedule appointments automatically, and provide consistent responses to common inquiries about your genomic services or research initiatives.
The free account on Callin.io includes an intuitive interface for configuring your AI agent, with test calls included and access to the task dashboard for monitoring interactions. For more advanced features like Google Calendar integration and built-in CRM functionality, subscription plans start at just $30 per month. As genomic medicine becomes increasingly mainstream, having intelligent communication tools that can effectively explain complex genetic concepts and coordinate patient care will become essential components of the healthcare ecosystem. Learn more by visiting Callin.io today.
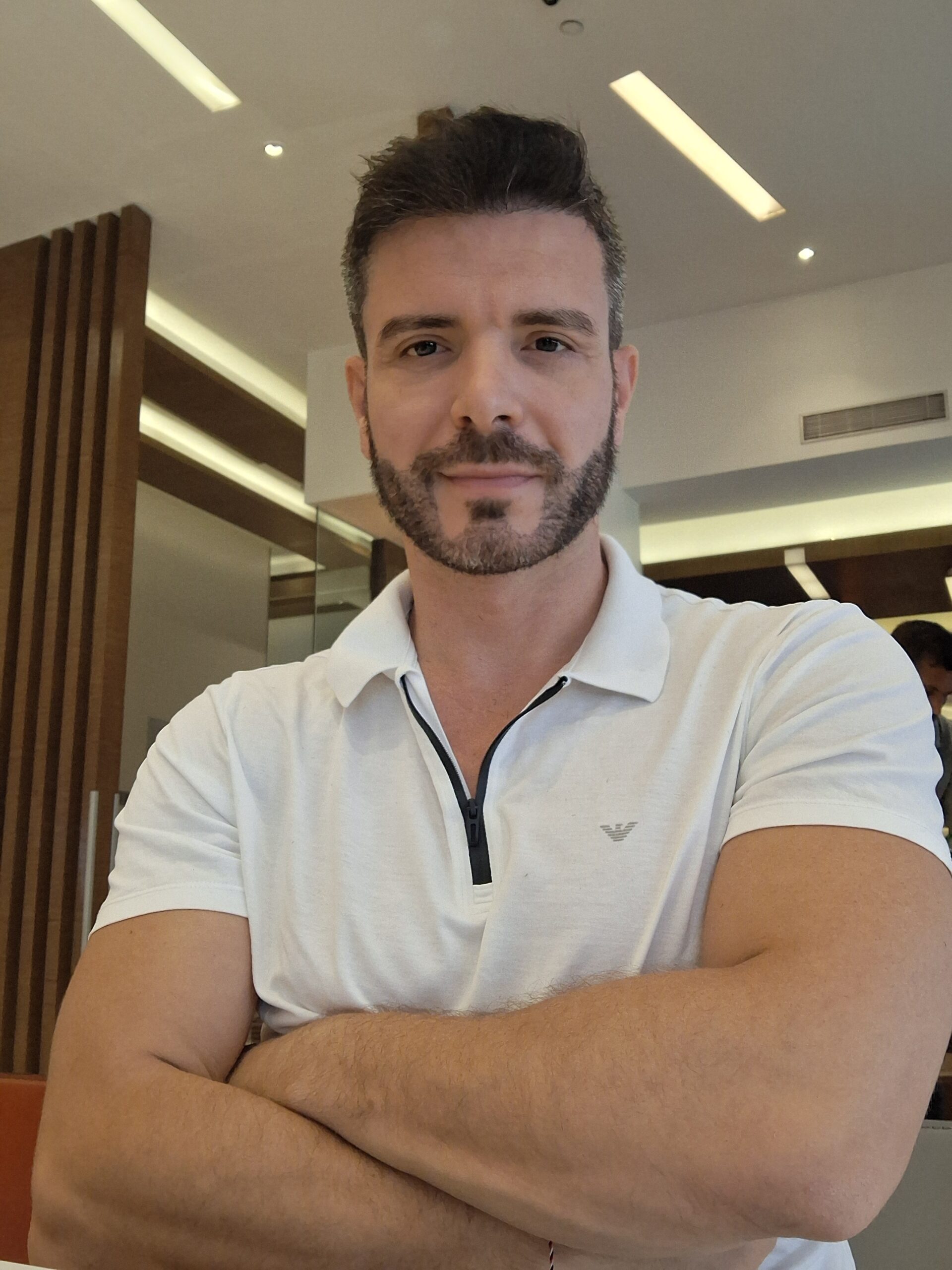
Helping businesses grow faster with AI. 🚀 At Callin.io, we make it easy for companies close more deals, engage customers more effectively, and scale their growth with smart AI voice assistants. Ready to transform your business with AI? 📅 Let’s talk!
Vincenzo Piccolo
Chief Executive Officer and Co Founder