Unveiling the Power of Computer Vision
Computer vision represents a fascinating branch of artificial intelligence that trains computers to interpret and understand visual information from the world. This technology empowers machines to "see" and process images similarly to human vision but often with greater precision and consistency. Unlike traditional image processing, computer vision AI solutions leverage deep learning algorithms to extract meaningful insights from visual data, making decisions based on what they "see." The applications span across numerous industries—from retail analytics to healthcare diagnostics, manufacturing quality control to autonomous vehicles. As businesses generate unprecedented volumes of visual data daily, the need for sophisticated AI solutions for computer vision has never been more pressing to convert these visual assets into actionable business intelligence.
The Core Technologies Driving Computer Vision
At the heart of modern computer vision systems lies a sophisticated stack of technologies working in harmony. Convolutional Neural Networks (CNNs) form the backbone, specialized neural networks architecturally designed to process pixel data optimally. These networks are complemented by object detection frameworks like YOLO (You Only Look Once) and R-CNN (Region-based CNN) that enable systems to identify multiple objects in a single image with remarkable speed and accuracy. Transfer learning techniques allow developers to build upon pre-trained models, saving computational resources and development time. The technological ecosystem supporting computer vision has matured significantly, with hardware accelerators like GPUs and TPUs providing the computational muscle needed for real-time image processing. This convergence of specialized algorithms, efficient architectures, and purpose-built hardware has democratized access to powerful visual AI capabilities that were once restricted to research labs with supercomputing resources.
Real-World Applications in Retail and E-commerce
The retail sector has embraced computer vision technologies with remarkable enthusiasm, using these AI solutions to reinvent shopping experiences both online and in physical stores. In brick-and-mortar locations, smart shelving systems equipped with computer vision monitor inventory levels in real-time, automatically alerting staff when restocking is needed. Checkout-free stores, pioneered by Amazon Go, use networks of cameras and deep learning models to track what customers pick up and automatically charge their accounts when they leave. In the e-commerce realm, visual search capabilities allow shoppers to find products by uploading images rather than typing text descriptions, dramatically improving the discovery process. Behind the scenes, computer vision powers product recommendation engines by analyzing visual similarities between items, creating more intuitive shopping experiences. Companies like Syte and ViSenze have developed specialized retail computer vision platforms that can identify products, attributes, and even fashion trends from images, providing retailers with powerful tools to understand customer preferences and optimize their offerings.
Healthcare Innovations Through Visual Intelligence
In healthcare, computer vision AI is revolutionizing diagnostics, patient care, and medical research with unprecedented precision. Medical imaging analysis has been transformed by deep learning models that can detect subtle abnormalities in X-rays, MRIs, and CT scans, sometimes outperforming experienced radiologists in specific tasks. For instance, AI systems for retinal scanning can identify early signs of diabetic retinopathy, potentially saving millions from vision loss through early intervention. During surgical procedures, computer vision platforms provide real-time guidance and anatomical mapping, enhancing precision and reducing risks. In rehabilitation, motion analysis systems track patient movements to create personalized therapy programs and measure progress objectively. Perhaps most promising is the application in drug discovery, where visual analysis of microscopic cellular responses helps identify promising compounds and predict their effectiveness. The integration of these technologies with conversational AI systems enables more comprehensive patient monitoring and care coordination, creating a powerful ecosystem of complementary healthcare technologies.
Manufacturing Quality Control and Process Optimization
Manufacturing environments have become fertile ground for computer vision implementation, with visual inspection systems forming the cornerstone of modern quality assurance protocols. These AI-powered systems can inspect products at speeds and accuracy levels impossible for human inspectors, detecting microscopic defects in everything from microchips to automobile parts. Beyond simple defect detection, advanced computer vision platforms analyze entire production lines, identifying bottlenecks, predicting maintenance needs, and optimizing workflow efficiency. Companies like Toyota and Intel have reported substantial improvements in production yield and significant reductions in waste after implementing these technologies. Particularly noteworthy is the ability of these systems to learn continuously, adapting to new product variations and previously unseen defect types without requiring extensive reprogramming. This self-improving capability makes computer vision particularly valuable in manufacturing contexts with frequent product changes or customization requirements. For specialized manufacturing applications, platforms like Cognex and Landing AI provide industry-specific computer vision solutions designed to integrate seamlessly with existing production equipment.
Autonomous Vehicles and Transportation Systems
The transportation industry stands at the cusp of a revolution driven largely by computer vision technologies. Self-driving vehicle systems rely on complex networks of cameras and sensors interpreted by sophisticated vision algorithms to navigate roads safely. These AI systems must perform multiple visual tasks simultaneously—identifying road markings, recognizing traffic signs, detecting pedestrians, and predicting the movements of other vehicles. Companies like Tesla, Waymo, and Mobileye have developed proprietary computer vision systems that continuously improve through the analysis of millions of real-world driving miles. Beyond personal transportation, computer vision enables smart traffic management systems that automatically adjust signal timing based on real-time traffic flow analysis, potentially reducing congestion by up to 25% in urban centers. In logistics and fleet management, visual monitoring systems track vehicle conditions, driver alertness, and cargo security, creating safer and more efficient transportation networks. The integration of these visual technologies with voice-based AI systems enables natural human-machine interfaces for drivers and passengers, enhancing both the utility and accessibility of these advanced transportation systems.
Security and Surveillance Enhancements
Computer vision has dramatically transformed security operations, enabling more intelligent and proactive surveillance capabilities. Advanced facial recognition systems can identify individuals in crowds with increasing accuracy, helping authorities locate persons of interest in public spaces. Behavior analysis algorithms detect suspicious activities or anomalous patterns, alerting security personnel before incidents escalate. In retail environments, theft prevention systems using computer vision have demonstrated the ability to reduce shrinkage by identifying shoplifting behaviors in real-time. For corporate security, these technologies provide sophisticated access control through biometric verification that’s more secure and convenient than traditional methods. The integration with AI communication systems allows for immediate notification and response coordination when security events are detected. However, this domain also raises significant ethical considerations regarding privacy, surveillance, and potential bias in recognition systems. Leading providers like Avigilon and Briefcam have developed privacy-conscious security platforms that balance powerful detection capabilities with responsible implementation practices, setting new standards for ethical deployment of visual security technologies.
Smart Cities and Urban Planning Applications
Urban environments present rich opportunities for computer vision deployment, creating safer, more efficient, and responsive city infrastructure. Traffic monitoring systems equipped with computer vision analyze vehicular and pedestrian flow patterns, optimizing signal timing and identifying areas prone to congestion or accidents. Smart parking solutions use overhead cameras to identify available spaces, directing drivers efficiently and reducing the estimated 30% of urban congestion caused by parking searches. In public safety applications, video analytics help emergency services respond more effectively by identifying incidents like fires, accidents, or flooding through camera networks. Environmental monitoring systems track air quality, waste management efficiency, and urban green space health using visual data. Cities like Singapore and Barcelona have implemented comprehensive urban visual intelligence platforms that integrate these various systems, creating unified city management dashboards that help officials make data-driven decisions. The combination of these visual technologies with conversational AI interfaces creates powerful citizen engagement tools, allowing residents to report issues, request information, and participate in urban planning processes through natural language interactions.
Agriculture and Environmental Monitoring
Agricultural operations increasingly rely on computer vision technologies to optimize crop management and resource utilization. Drone-based imaging systems equipped with multispectral cameras capture detailed field conditions, with AI algorithms analyzing the imagery to detect plant stress, disease outbreaks, and nutrient deficiencies before they become visible to the human eye. These early warning systems allow for targeted interventions rather than blanket applications of water, fertilizers, or pesticides—reducing costs while minimizing environmental impact. Harvesting robots use computer vision to identify ripe produce and determine optimal picking patterns, addressing labor shortages in many agricultural regions. Beyond farm boundaries, environmental scientists deploy similar technologies to monitor forest health, track wildlife populations, and assess ecosystem changes over time. Organizations like The Nature Conservancy use computer vision to analyze satellite imagery and identify deforestation, helping guide conservation efforts more effectively. The integration of these visual monitoring capabilities with automated communication systems enables timely alerts and coordinated responses to environmental threats, creating more resilient agricultural and ecological management systems.
Augmented and Virtual Reality Integration
The fusion of computer vision with augmented and virtual reality technologies has created compelling new interaction paradigms. AR applications rely heavily on computer vision for environmental understanding—tracking spatial features to properly anchor virtual objects in the physical world. This spatial awareness enables applications ranging from furniture placement visualization in retail to complex maintenance guidance in industrial settings. In mixed reality environments, computer vision algorithms continuously map the user’s surroundings, identifying objects, surfaces, and spaces to create seamless blending of virtual and physical elements. Major platforms like Apple’s ARKit and Google’s ARCore provide developers with sophisticated computer vision capabilities packaged as accessible tools for creating immersive experiences. Educational applications particularly benefit from these technologies, allowing students to interact with virtual models of complex systems—from anatomical structures to architectural designs—placed contextually in their actual environment. When combined with voice interaction capabilities, these systems create natural multimodal interfaces that dramatically reduce the learning curve for complex technologies, making advanced tools more accessible to non-technical users.
The Role of Computer Vision in Content Moderation
Content moderation represents one of the most challenging applications of computer vision, with platforms processing billions of user-uploaded images and videos daily. AI-powered visual moderation systems identify problematic content—from explicit material to graphic violence, hate symbols to copyright infringements—with increasing accuracy. These systems handle the overwhelming volume of content that would be impossible to review manually, filtering obvious violations automatically while flagging borderline cases for human review. Companies like TikTok and Instagram rely heavily on computer vision to maintain platform standards while managing exponential content growth. Beyond explicit policy violations, sophisticated sentiment analysis of visual content helps identify subtle forms of harassment or bullying that might otherwise go undetected. Content categorization capabilities also enable better recommendation systems and more effective content discovery, improving user experience. The integration with textual analysis AI creates comprehensive moderation systems that understand content in context, reducing false positives and improving overall accuracy. As social platforms become increasingly visual and video-centric, these technologies have become essential infrastructure for maintaining healthy online communities.
Computer Vision for Financial Services and Fraud Detection
Financial institutions have discovered valuable applications for computer vision in enhancing security and streamlining customer experiences. Biometric authentication systems using facial recognition provide secure account access while reducing friction compared to traditional passwords or security questions. Check processing has been revolutionized by computer vision systems that can automatically extract payment information, verify signatures, and detect potential forgeries more accurately than manual processing. In physical locations, visual analytics track customer journeys through branches, helping optimize layout and staffing based on traffic patterns and service utilization. For fraud detection, computer vision analyzes ATM and banking hall footage to identify suspicious behaviors or known fraudsters. Credit card companies use similar technologies to detect unusual patterns in transaction-related imagery that might indicate compromised terminals or skimming devices. These capabilities complement the AI voice agents that many financial institutions have deployed for customer service, creating multilayered security and service enhancement systems. Leading providers like Jumio have developed specialized financial services computer vision platforms that combine these various capabilities while maintaining compliance with stringent financial industry regulations.
Challenges in Data Collection and Annotation
Building effective computer vision systems requires massive datasets of properly annotated images—a requirement that presents significant practical challenges. Data collection strategies must ensure sufficient quantity while maintaining quality, diversity, and representativeness. The annotation process itself is labor-intensive, with even moderately complex vision systems requiring millions of labeled examples for training. Companies often leverage specialized annotation services or crowd-sourcing platforms to manage this workload, though quality control remains challenging. Synthetic data generation has emerged as a promising alternative, using 3D modeling and rendering to create perfectly labeled training images without manual annotation. However, the "reality gap" between synthetic and real-world imagery can limit effectiveness if not carefully managed. Privacy concerns further complicate data collection, particularly for applications involving human subjects or personal environments. The AI voice technology sector faces similar challenges with audio data collection and annotation, creating opportunities for shared solutions and best practices between these related fields. Organizations like Scale AI and Labelbox have developed specialized platforms to streamline the annotation workflow, incorporating quality assurance mechanisms and leveraging AI assistance to accelerate the process while maintaining accuracy.
Ethical Considerations and Bias Mitigation
The deployment of computer vision systems raises important ethical questions that developers and organizations must address proactively. Algorithmic bias presents a significant concern, as vision systems may perform unequally across different demographic groups due to imbalanced training data or flawed algorithm design. Testing for fairness across diverse populations has become an essential development step, with tools emerging to help identify and mitigate such biases. Privacy implications are equally important, as the proliferation of visual analysis capabilities raises questions about appropriate limits on surveillance and data collection. Transparency represents another critical consideration—users should understand when they’re being analyzed by visual AI systems and how that information will be used. Organizations like the Partnership on AI have developed frameworks for responsible AI deployment that specifically address computer vision applications. These ethical considerations parallel those in voice AI development, where issues of consent, privacy, and representation similarly require thoughtful approaches. Leading computer vision providers increasingly incorporate ethical review processes in their development pipelines, recognizing that long-term success depends not just on technical performance, but on building systems that earn and maintain public trust.
Edge Computing and On-Device Vision Processing
The shift toward edge computing has transformed computer vision deployment, enabling sophisticated visual analysis directly on cameras and devices rather than requiring cloud processing. This architectural approach offers several advantages: reduced latency for time-sensitive applications, continued functionality during network outages, and enhanced privacy as sensitive visual data remains local. Mobile vision frameworks like TensorFlow Lite and CoreML allow developers to deploy optimized models on smartphones and tablets, enabling applications from real-time translation of text in camera viewfinders to skin condition analysis in healthcare apps. Purpose-built vision processors from companies like Intel’s Movidius and Google’s Edge TPU provide dedicated hardware acceleration for vision tasks in IoT devices, security cameras, and industrial equipment. This edge-focused approach complements similar trends in voice AI processing, where on-device speech recognition reduces latency and privacy concerns. The resulting ecosystem enables powerful multimodal AI applications that combine vision and voice capabilities without requiring continuous cloud connectivity, expanding the potential deployment contexts for these technologies beyond environments with reliable high-bandwidth connections.
Small Business Applications of Computer Vision
While enterprise implementations often dominate discussions of computer vision, small businesses are finding accessible entry points to leverage this technology. Retail analytics systems using basic computer vision can provide valuable customer insights without massive infrastructure investments. Simple camera setups with cloud-based analysis can track store traffic patterns, determine display effectiveness, and measure customer engagement with products. For restaurants and cafes, similar systems help optimize seating arrangements, analyze menu board engagement, and improve service timing. Service businesses like salons and spas use visual tracking to manage appointment flows and identify operational bottlenecks. E-commerce entrepreneurs leverage product recognition APIs to automatically categorize inventory and generate consistent product attributes. These smaller-scale implementations often provide substantial ROI through operational efficiencies and enhanced customer experiences. The integration of these visual capabilities with AI phone services creates powerful business enhancement systems accessible even to businesses with limited technical resources. Solutions providers like Plainsight and Roboflow have developed platforms specifically targeting small business use cases, with simplified interfaces and pre-built models that require minimal technical expertise to implement.
Computer Vision for Accessibility and Inclusion
Computer vision technologies have created powerful tools to enhance accessibility for individuals with disabilities, demonstrating the profound social impact potential of these AI systems. Visual assistance applications help blind and visually impaired users navigate environments, identify objects, read text, and recognize people. Microsoft’s Seeing AI and Google’s Lookout exemplify these capabilities, turning visual information into audio descriptions. For deaf and hard-of-hearing individuals, computer vision powers sign language recognition systems and real-time captioning of in-person conversations. In educational settings, these technologies help create more inclusive learning environments by automatically generating alternative formats of visual materials. For individuals with mobility impairments, vision-based gesture recognition enables new forms of environmental control and computer interaction. These accessibility applications highlight how computer vision can serve humanitarian purposes beyond commercial objectives. The combination of these visual accessibility tools with voice AI technologies creates comprehensive accessibility solutions that address multiple sensory channels, providing redundant information pathways that enhance usability for diverse users with varying abilities and preferences.
Future Trends: Multimodal AI and Beyond
The future of computer vision lies in deeper integration with other AI modalities, creating systems that interpret the world through multiple complementary perceptual channels. Multimodal AI systems combining vision, language, and audio understanding can develop more comprehensive scene understanding and contextual awareness than any single modality alone. Research directions like visual question answering (VQA) and image captioning demonstrate the power of these combined approaches. The integration of computer vision with robotics will continue accelerating, enabling more capable physical agents that can navigate complex environments and manipulate objects with increasing dexterity. Emerging work in few-shot and zero-shot learning promises to reduce the massive data requirements currently limiting deployment in specialized domains. Looking further ahead, neuromorphic vision systems inspired by the human visual cortex may enable more efficient processing with dramatically lower power requirements. These advancements will expand the application possibilities while making sophisticated vision capabilities accessible to smaller devices and broader use cases. The intersection with conversational AI technologies will be particularly transformative, enabling natural human-machine interactions based on both visual and verbal information—similar to how humans communicate with each other through multiple coordinated channels.
Performance Optimization and Model Efficiency
As computer vision applications proliferate across industries, optimizing model performance and efficiency has become increasingly important. Model compression techniques like quantization, pruning, and knowledge distillation allow developers to significantly reduce model size and computational requirements without substantial accuracy losses. These approaches are particularly critical for edge deployments where processing power, memory, and energy may be severely constrained. Hardware-aware model design considers the specific capabilities of target processors during the architecture development process, rather than treating optimization as an afterthought. Automated neural architecture search (NAS) algorithms help identify optimal model structures for specific tasks and deployment environments, often discovering designs that outperform human-created architectures. For production systems, techniques like model compilation and runtime optimization further enhance performance by taking advantage of specific hardware capabilities. Organizations must carefully balance accuracy requirements against resource constraints, particularly in applications like AI calling systems where multiple AI modalities must share limited computational resources. Tools like ONNX (Open Neural Network Exchange) and TVM have emerged as important elements of the optimization ecosystem, enabling model portability and performance across diverse deployment targets.
Implementation Strategies for Business Leaders
For business leaders evaluating computer vision investments, developing a structured implementation strategy increases the likelihood of successful deployment and positive ROI. The process should begin with problem identification—clearly defining specific business challenges where visual data analysis could provide meaningful insights or process improvements. Evaluation of existing solutions should consider both commercial off-the-shelf options and customized approaches based on organization-specific requirements. Pilot projects with clearly defined success metrics help validate concepts before broader implementation, while providing valuable insights for larger deployments. Cross-functional teams including both technical specialists and business stakeholders ensure solutions address actual business needs rather than showcasing technology for its own sake. Integration planning must consider how computer vision systems will connect with existing business processes, data systems, and decision-making frameworks. For organizations already utilizing AI communication systems, identifying integration opportunities between visual and conversational AI can create particularly valuable combined capabilities. Change management strategies must address potential workforce concerns and provide appropriate training for employees interacting with or affected by these new systems. A phased implementation approach typically yields better results than attempting wholesale transformation, allowing organizations to build internal expertise while delivering incremental value.
Harness the Power of Visual Intelligence for Your Business
The potent combination of computer vision and other AI technologies is reshaping how businesses operate across virtually every industry. From retail analytics to manufacturing quality control, healthcare diagnostics to customer service enhancement, these visual intelligence systems convert raw image and video data into actionable insights and automated processes. As the technology continues maturing, implementation barriers have decreased while capabilities have expanded dramatically. Organizations that strategically deploy these technologies gain significant competitive advantages through improved efficiency, enhanced customer experiences, and data-driven decision making supported by previously inaccessible visual information. Whether you’re considering your first computer vision implementation or looking to expand existing capabilities, the key to success lies in identifying specific business problems where visual analysis creates measurable value, then building solutions proportionate to your operational needs and technical capabilities. Like other transformative technologies, the greatest benefits come not from the technology itself, but from thoughtful application to meaningful business challenges.
Transform Your Communication Strategy with AI-Powered Solutions
If you’re looking to leverage AI for business communication in ways similar to how computer vision transforms visual data, Callin.io offers powerful solutions worth exploring. This platform enables you to implement AI-powered phone agents that handle incoming and outgoing calls autonomously, complementing visual intelligence systems with sophisticated voice interaction capabilities. With Callin.io’s AI phone agents, you can automate appointment scheduling, answer frequent customer questions, and even close sales through natural-sounding conversations that enhance the customer experience.
Creating your account on Callin.io provides access to an intuitive interface for configuring your AI agent, with test calls included and a comprehensive task dashboard to monitor interactions. For businesses requiring advanced functionality, such as Google Calendar integration and built-in CRM capabilities, subscription plans start at just 30USD monthly. By combining visual intelligence systems with AI-powered communication tools, you can create a comprehensive ecosystem that addresses multiple aspects of customer interaction and business operations. Discover more about these complementary AI capabilities at Callin.io.
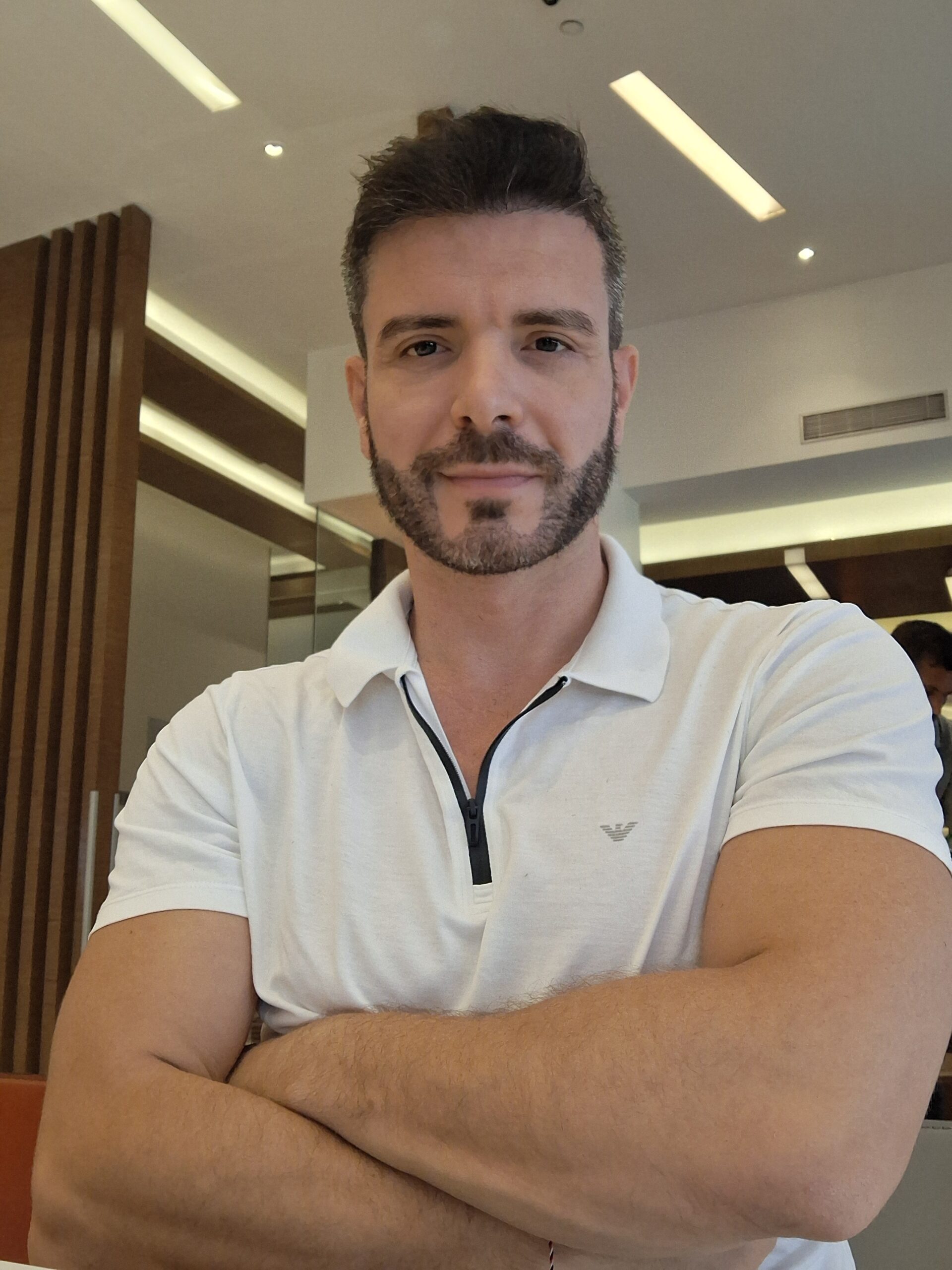
Helping businesses grow faster with AI. 🚀 At Callin.io, we make it easy for companies close more deals, engage customers more effectively, and scale their growth with smart AI voice assistants. Ready to transform your business with AI? 📅 Let’s talk!
Vincenzo Piccolo
Chief Executive Officer and Co Founder