The Rising Challenge of Money Laundering in Financial Systems
Financial crime continues to plague global banking systems despite increasingly stringent regulatory frameworks. Money laundering transactions account for approximately 2-5% of global GDP annually, translating to a staggering $800 billion to $2 trillion according to United Nations estimates. This criminal activity has become more sophisticated with digital transformation, making traditional detection methods increasingly inadequate. Financial institutions face mounting pressure to implement robust anti-money laundering (AML) measures while simultaneously managing operational costs and avoiding customer friction. The complexity of modern financial networks, coupled with the ingenuity of money launderers who constantly adapt their methods, creates a perfect storm that traditional rule-based systems struggle to weather. As regulatory penalties for AML failures continue to climb into billions of dollars annually, financial organizations are turning to artificial intelligence as the most promising solution for this escalating battle against financial crime and terrorist financing networks.
Understanding Traditional AML Approaches and Their Limitations
For decades, financial institutions have relied on rule-based transaction monitoring systems that essentially operate on predefined thresholds and patterns. These legacy systems typically flag transactions based on rigid criteria such as unusually large deposits, structured transactions just below reporting thresholds, or unusual cross-border transfers. While these approaches have provided a baseline defense, they generate an overwhelming number of false positives—some institutions report false positive rates exceeding 95%. This creates a massive operational burden as compliance teams must manually investigate each alert, leading to significant resource allocation challenges. Furthermore, traditional systems offer limited adaptability to new laundering techniques, creating dangerous blind spots. Without contextual awareness, these systems fail to understand the full customer relationship and transaction history, missing complex money laundering schemes that deliberately operate across multiple accounts, institutions, or time periods to avoid detection. This fundamental gap in capability has driven the urgent need for AI-enhanced AML solutions that can bring intelligence and adaptability to financial crime detection.
How AI Is Redefining Anti-Money Laundering Practices
Artificial intelligence is revolutionizing AML operations by introducing sophisticated pattern recognition capabilities far beyond human capacity. Machine learning algorithms can analyze billions of transactions, establishing baseline behaviors for individual customers, business segments, and regional activities, then flagging genuine anomalies with remarkable precision. Unlike static rule-based systems, these AI models evolve through continuous learning, enabling them to identify new laundering techniques as they emerge. Natural language processing components can analyze unstructured data from news sources, social media, and other external datasets to provide crucial contextual intelligence about potential money laundering networks. Perhaps most significantly, AI systems can reduce false positive alerts by 60-80%, according to implementation data from major financial institutions, allowing compliance teams to focus on genuine threats rather than chasing phantom violations. These systems don’t merely flag suspicious activity—they prioritize alerts based on risk scoring, provide explanation frameworks for why transactions were flagged, and suggest investigation pathways. The integration of conversational AI capabilities further enhances the investigation process by enabling compliance analysts to query the system using natural language, dramatically accelerating case resolution timeframes.
Machine Learning Models at the Core of Effective AML Solutions
The true power of AI in anti-money laundering lies in the specific machine learning methodologies deployed. Supervised learning models establish patterns from historical data where outcomes are known, effectively identifying previously recognized laundering typologies. Meanwhile, unsupervised learning algorithms excel at detecting anomalies without prior examples, identifying unusual patterns that may indicate new money laundering techniques. The real breakthrough comes from deep learning networks that can analyze complex relationships across vast datasets, recognizing sophisticated laundering networks that operate across multiple institutions and jurisdictions. These models incorporate graph analytics to map relationships between entities, transactions, and accounts, visualizing complex criminal networks that would remain invisible to traditional systems. As noted in research from Stanford University’s AI Index, financial crime detection has seen some of the most promising real-world applications of advanced neural network architectures. What makes these models particularly valuable is their ability to incorporate temporal analysis—understanding how transaction patterns evolve over time—which is crucial because money launderers typically conduct operations across extended timeframes to avoid detection. The AI voice assistants being developed for financial compliance teams further enhance these capabilities by providing intuitive interfaces for exploring the intelligence generated by these complex models.
Real-Time Monitoring and Risk Scoring: The AI Advantage
Traditional AML systems typically operate on batch processing, reviewing transactions after they’ve already occurred—often days later. AI-powered solutions enable real-time transaction screening, allowing suspicious activities to be identified and potentially blocked before completion. This capability transforms AML from a retrospective compliance exercise to a proactive crime prevention tool. Advanced risk scoring algorithms continuously evaluate customers and transactions across multiple dimensions, creating dynamic risk profiles that adjust based on behavior patterns and external intelligence. These systems can instantly recalculate risk scores when new information emerges or transaction patterns change, ensuring that high-risk activities receive immediate attention. According to implementation data from FinCEN, financial institutions utilizing AI-powered real-time monitoring have reported up to 30% improvement in suspicious activity detection rates. The integration of these systems with AI call center solutions allows immediate communication with customers when potentially suspicious activities are detected, reducing legitimate transaction friction while maintaining vigilance against criminal activity. This real-time capability represents a fundamental shift in how financial institutions approach money laundering prevention.
Enhanced Customer Due Diligence Through Intelligent KYC
Know Your Customer (KYC) procedures form the foundation of effective AML programs, but traditional approaches often create significant customer friction while still missing sophisticated criminal actors. AI-enhanced KYC solutions transform this critical process by automating document verification, conducting advanced identity authentication, and providing continuous customer monitoring rather than point-in-time checks. These systems can analyze identity documents in seconds, verify biometric data for authentication, and cross-reference information against multiple databases simultaneously. Beyond mere verification, AI algorithms assess the internal consistency of customer information, flagging discrepancies that might indicate synthetic identities or front companies. The Journal of Financial Crime reports that AI-powered KYC systems reduce onboarding times by up to 80% while simultaneously improving risk detection. When integrated with conversational AI for business, these systems can interact directly with customers to resolve information gaps or verification issues, creating a seamless experience for legitimate customers while maintaining robust defenses against money launderers. This continuous intelligence approach ensures that financial institutions maintain an accurate understanding of their customers throughout the relationship.
Network Analysis and Entity Resolution in Complex Cases
Money laundering schemes rarely operate in isolation—they typically involve networks of individuals, shell companies, and multiple financial institutions. AI excels at uncovering these complex relationships through advanced network analysis and entity resolution capabilities. These systems can identify connections between seemingly unrelated accounts, recognize when different identities likely represent the same entity, and map the flow of funds across complex networks. By applying graph theory and relationship analytics, AI solutions visualize money movement patterns that would remain hidden to conventional analysis. This network-centric approach is particularly effective at identifying professional money laundering operations that deliberately structure their activities to evade traditional detection methods. Financial institutions implementing these network analysis capabilities report up to 50% improvement in identifying coordinated money laundering rings, according to case studies published by the Financial Action Task Force. When combined with AI call assistants that enable investigators to explore these networks through conversational interfaces, financial institutions gain unprecedented visibility into complex criminal operations, dramatically improving their ability to disrupt laundering networks rather than merely flagging individual transactions.
Regulatory Compliance and Explainable AI in AML Solutions
As financial institutions deploy increasingly sophisticated AI systems for AML, regulatory expectations around transparency and explainability have intensified. Regulators demand that financial institutions understand and can explain how their AI models make decisions—creating a tension between advanced algorithms and compliance requirements. Modern AML solutions address this challenge through explainable AI frameworks that provide clear reasoning behind flagged transactions and risk assessments. These systems generate detailed documentation of decision pathways, feature importance metrics, and confidence scores that satisfy regulatory expectations while maintaining detection effectiveness. The Financial Conduct Authority in the UK has recognized explainable AI as a critical component of acceptable AML automation in its recent guidance. Beyond mere compliance, these explainability features provide practical benefits for investigative staff, who can understand why a transaction was flagged and focus their investigation accordingly. Financial institutions implementing AI phone agents with these explainability features report significantly improved collaboration between technology systems and human compliance analysts, creating an effective partnership that satisfies both operational and regulatory requirements.
Behavioral Analytics: Understanding the Human Elements of Money Laundering
The psychological and behavioral dimensions of money laundering often provide crucial indicators that pure transaction analysis might miss. Advanced AI solutions incorporate behavioral analytics that establish baseline patterns for customers and detect meaningful deviations that could indicate criminal activity. These systems analyze interaction patterns across multiple channels, including online banking behavior, customer service interactions, and transaction timing. By understanding typical business cycles, income patterns, and spending behaviors, these models identify subtle anomalies that might indicate account takeover or money mule activity. Research from Carnegie Mellon University demonstrates that incorporating behavioral indicators can improve money laundering detection rates by up to 35% compared to transaction-only analysis. Financial institutions using AI voice conversation tools gain additional behavioral insights through voice pattern analysis and conversation content, providing another dimension of intelligence for AML programs. This holistic understanding of customer behavior enables more accurate risk assessment while reducing disruptions to legitimate customer activities, striking the crucial balance between security and service that defines successful AML programs.
Integrating External Data Sources for Contextual Intelligence
Money laundering detection significantly improves when transaction data is enriched with external contextual information. Advanced AI solutions incorporate diverse data sources including news feeds, corporate registries, court records, property databases, and social media intelligence to create comprehensive risk assessment. Natural language processing capabilities analyze unstructured text data to identify potential reputational issues, political exposure, or other risk factors that might not appear in traditional structured data. These systems can automatically monitor thousands of news sources in multiple languages, identifying emerging risks associated with customers or their business associates. According to ACAMS (Association of Certified Anti-Money Laundering Specialists), financial institutions integrating external data sources report approximately 40% higher suspicious activity identification rates. This contextual intelligence approach is particularly valuable for identifying ultimate beneficial owners and complex corporate structures designed to obscure ownership. When combined with AI phone services that can proactively contact customers for verification when potential issues are identified, these systems create a powerful framework for identifying sophisticated money laundering operations that traditional methods would miss.
Case Management and Investigation Acceleration with AI Assistance
Even with advanced detection systems, AML programs ultimately require human investigation and judgment. AI dramatically enhances the effectiveness of these investigation processes through intelligent case management tools that prioritize alerts, suggest investigation pathways, and automate evidence gathering. These systems analyze historical case outcomes to recommend the most effective investigation strategies for similar cases, helping investigators focus their efforts productively. Automated evidence gathering capabilities pull relevant information from multiple systems, assembling comprehensive case files that would take hours to compile manually. Natural language generation features automatically draft preliminary investigation reports, allowing investigators to focus on analysis rather than documentation. Financial institutions implementing these AI investigation tools report productivity improvements of 40-60% in case processing times, according to industry benchmarks. When integrated with virtual receptionists and AI calling solutions, these systems enable seamless communication between investigation teams and other stakeholders, ensuring that suspicious activity receives prompt attention from the appropriate specialists.
Detecting Emerging Threats: Cryptocurrency and Digital Payment Laundering
The rapid expansion of cryptocurrencies and digital payment platforms has created new challenges for AML programs as criminals exploit these systems for money laundering. AI solutions help financial institutions address these emerging threats through specialized monitoring capabilities designed for digital asset transactions. These systems analyze blockchain transactions to identify suspicious patterns, recognize mixing services that attempt to obscure the source of funds, and track cross-chain activities that might indicate laundering. Advanced risk scoring algorithms assess cryptocurrency wallets based on transaction history and connection to high-risk entities, providing an effective framework for managing digital asset risk. According to the Financial Action Task Force’s Virtual Assets Red Flag Indicators, AI-powered detection systems demonstrate significantly higher accuracy in identifying cryptocurrency-based money laundering than conventional approaches. Financial institutions implementing AI bot solutions for customer interaction about digital asset transactions gain additional intelligence about potential risks while providing seamless service for legitimate customers. This technology-focused approach ensures that AML programs remain effective as financial services continue their digital transformation.
Cost Reduction and Efficiency Gains in AML Operations
The financial burden of AML compliance has become substantial, with large institutions spending hundreds of millions annually on financial crime prevention. AI implementation delivers significant cost efficiencies while simultaneously improving detection effectiveness. By reducing false positive alerts by 60-80%, these systems dramatically decrease the investigative workload, allowing financial institutions to manage compliance requirements without proportional staffing increases. Automated case documentation and evidence gathering eliminate hours of manual work per case, further improving operational efficiency. These systems enable risk-based approaches to compliance, focusing resources on genuine high-risk activities rather than spreading attention evenly across all transactions. According to McKinsey & Company, financial institutions implementing comprehensive AI-powered AML programs report cost reductions of 20-30% within the first year while improving detection rates. When combined with AI sales capabilities that can explain compliance requirements to customers, these systems transform AML from a pure cost center to a component of customer relationship management. This economic advantage creates compelling return on investment for financial institutions implementing AI-powered AML solutions.
Privacy Preservation and Data Protection in AI-Powered AML
As AI systems analyze increasingly diverse data for AML purposes, privacy concerns have become a critical consideration for financial institutions. Advanced solutions incorporate privacy-preserving technologies that enable effective analysis while protecting sensitive customer information. These approaches include federated learning models that analyze data without centralizing it, differential privacy techniques that add statistical noise to protect individual records, and homomorphic encryption that allows analysis of encrypted data without decryption. These privacy-enhancing technologies are particularly important for cross-institutional cooperation against money laundering, allowing financial organizations to collaborate without sharing raw customer data. The European Union Agency for Cybersecurity has recognized these technologies as essential for balancing effective AML with data protection requirements. Financial institutions implementing AI calling bots with these privacy safeguards can gather additional verification information when needed while maintaining robust data protection. This privacy-centric approach ensures that AML programs comply with regulations like GDPR and CCPA while still leveraging the full power of artificial intelligence for financial crime detection.
Implementing AI Solutions: Integration with Existing AML Infrastructure
For most financial institutions, implementing AI for AML represents an enhancement to existing systems rather than a complete replacement. Successful implementation requires thoughtful integration with legacy transaction monitoring systems, case management tools, and customer databases. Modern solutions offer flexible deployment models including cloud-based options that minimize infrastructure requirements, hybrid approaches that keep sensitive data on-premises while leveraging cloud computing power, and fully integrated enterprise implementations. API-driven architectures enable seamless connections to existing systems, allowing financial institutions to enhance their AML capabilities without disruptive rip-and-replace projects. According to implementation case studies from major financial technology providers, institutions following phased implementation approaches typically achieve positive ROI within 6-12 months. When combined with white-label AI solutions that can be customized to match institutional requirements and branding, these integration strategies enable financial organizations of all sizes to access sophisticated AI capabilities for AML. This flexible approach makes advanced AI accessible across the financial services spectrum, from global banks to community credit unions and fintech startups.
Model Governance and Ongoing Optimization of AI AML Systems
Deploying AI for anti-money laundering is not a one-time project but an ongoing program requiring robust governance frameworks. Effective model governance includes regular performance monitoring, periodic revalidation, bias detection and mitigation, and continuous retraining with new data. These governance processes ensure that AI systems maintain their effectiveness as money laundering techniques evolve and transaction patterns change. Audit trails document all model changes, validations, and performance metrics, creating comprehensive records for regulatory review. Financial institutions implementing these governance frameworks report significantly higher regulatory acceptance of their AI initiatives, according to Deloitte’s Risk Advisory practice. Ongoing optimization processes continuously refine models based on investigation outcomes, incorporating feedback from compliance analysts to improve future detection accuracy. When supported by AI call center solutions that facilitate communication between technology teams and compliance staff, these governance frameworks create sustainable, continuously improving AML programs that adapt to emerging threats while maintaining regulatory compliance.
Collaborative Defense: AI-Powered Information Sharing Between Institutions
Money laundering networks intentionally operate across multiple financial institutions to avoid detection, making cross-institutional cooperation essential for effective prevention. Advanced AI solutions enable secure information sharing through privacy-preserving technologies that allow collaborative defense without compromising customer confidentiality. These systems create secure environments where financial institutions can jointly analyze transaction patterns, share typologies, and identify connected activities across organizational boundaries. Secure multi-party computation allows risk scoring without revealing underlying customer data, while zero-knowledge proofs enable verification without sharing sensitive information. According to the Wolfsberg Group, financial institutions participating in these collaborative frameworks report up to 25% improvement in complex money laundering network detection. When enhanced with AI voice agents that facilitate secure communication between compliance teams at different institutions, these collaborative systems create powerful defenses against sophisticated money laundering operations. This industry-wide approach represents the future of financial crime prevention, transforming AML from isolated institutional efforts to a coordinated network defense.
The Human-AI Partnership in Modern AML Programs
Despite the power of artificial intelligence, the most effective AML programs create thoughtful partnerships between AI systems and human experts rather than pursuing full automation. These hybrid approaches leverage AI for data analysis, pattern recognition, and alert generation while reserving human judgment for complex decision-making, contextual understanding, and customer interaction. Training programs ensure that compliance teams understand AI capabilities and limitations, enabling effective collaboration where each partner contributes unique strengths. Human feedback loops continuously improve AI performance by incorporating investigator insights into model refinement. According to research from the MIT Sloan Management Review, organizations implementing these collaborative human-AI frameworks achieve approximately 30% better outcomes than either fully manual or fully automated approaches. Financial institutions using AI appointment setters and similar tools to manage communication between human experts and customers report significant improvements in both compliance effectiveness and customer satisfaction. This balanced approach ensures that financial institutions leverage the full power of artificial intelligence while maintaining the human judgment essential for complex AML decisions.
Measuring Success: KPIs and Performance Metrics for AI-Enhanced AML
Quantifying the effectiveness of AML programs presents significant challenges, as the true positive rate for money laundering detection remains unknown—successful launderers, by definition, avoid detection. Despite this fundamental challenge, financial institutions can implement comprehensive performance frameworks that evaluate their AI-enhanced AML programs across multiple dimensions. These frameworks typically include operational metrics such as false positive reduction, alert-to-SAR conversion rates, and investigation efficiency improvements. Regulatory metrics track examination outcomes, remediation requirements, and compliance with regulatory expectations. Financial impact metrics quantify cost reductions, resource optimization, and potential penalty avoidance. Customer experience metrics measure legitimate customer friction reduction and account opening efficiency. According to Accenture’s Financial Crime Analytics practice, organizations implementing these comprehensive measurement frameworks demonstrate approximately 35% better optimization of their AML programs over time compared to those using limited metrics. When supported by AI sales representatives that can explain AML requirements to customers, these measurement frameworks create a balanced approach that optimizes both compliance effectiveness and customer experience.
Future Directions: Quantum Computing and Advanced AI in AML
The future of anti-money laundering technology promises even more sophisticated capabilities as quantum computing and advanced AI techniques mature. Quantum computing offers potentially revolutionary capabilities for analyzing complex financial networks and identifying patterns invisible to classical computing approaches. Emerging AI techniques including neuro-symbolic systems that combine machine learning with logical reasoning, self-supervised learning models that require minimal labeled training data, and advanced synthetic data generation for improved model training are already showing promising results in research environments. These technologies will enable financial institutions to detect increasingly sophisticated laundering schemes while further reducing false positives and operational costs. The World Economic Forum’s Future of Financial Crime Prevention initiative forecasts that these technologies could reduce global money laundering success rates by 50% within the coming decade. Financial institutions investing in AI sales generation and similar technologies position themselves to adopt these advanced capabilities as they mature. This forward-looking approach ensures that AML programs continue to evolve alongside both criminal techniques and technological capabilities.
Regulatory Evolution: How AI Is Reshaping AML Compliance Expectations
Regulatory frameworks for anti-money laundering are increasingly acknowledging and even encouraging the use of artificial intelligence, creating new opportunities and requirements for financial institutions. Forward-thinking regulators including the Financial Conduct Authority (UK), the Monetary Authority of Singapore, and FinCEN in the United States have established regulatory sandboxes and innovation programs specifically focused on AI applications in financial crime prevention. These programs provide safe harbors for testing new approaches while ensuring appropriate oversight. Regulatory expectations are evolving from purely process-based compliance to effectiveness-focused outcomes, creating incentives for financial institutions to implement advanced detection capabilities. According to Thomson Reuters Regulatory Intelligence, over 70% of financial regulators globally now explicitly acknowledge AI as an acceptable component of AML programs when implemented with appropriate governance. Financial institutions leveraging AI phone numbers and similar technologies for regulatory communications report improved engagement with supervisory authorities. This regulatory evolution creates a supportive environment for continued AI innovation in anti-money laundering, benefiting both financial institutions and the integrity of the global financial system.
Empowering Your AML Strategy with Advanced AI Solutions
The battle against money laundering requires sophisticated technology matched with thoughtful implementation and governance. As financial crimes grow increasingly complex, AI-powered solutions have become essential rather than optional for effective detection and prevention. By implementing advanced machine learning models, real-time monitoring systems, and intelligent investigation tools, financial institutions can dramatically improve their defense capabilities while simultaneously reducing costs and enhancing customer experience. The most successful implementations thoughtfully integrate AI capabilities with existing systems and human expertise, creating flexible frameworks that adapt to emerging threats and regulatory expectations. As you evaluate AI enhancement opportunities for your AML program, focus on solutions that offer explainability, privacy preservation, and sustainable performance improvement rather than black-box approaches that may create regulatory concerns. The financial institutions that thrive in this challenging environment will be those that leverage these technologies to transform AML from a compliance burden into a strategic advantage that protects both their reputation and the integrity of the global financial system.
Strengthen Your Financial Security with Callin.io’s Intelligent Solutions
Ready to revolutionize your financial institution’s approach to anti-money laundering? Callin.io provides cutting-edge AI phone agents that can seamlessly integrate with your existing compliance framework, enhancing your AML capabilities while reducing operational costs. Our intelligent communication platform enables your compliance teams to manage investigations more efficiently, with AI-powered calling systems that can handle routine verification tasks, gather additional information when suspicious activities are detected, and document all interactions for regulatory purposes.
Callin.io’s free account offers an intuitive interface for configuring your AI agent, with test calls included and access to the comprehensive task dashboard for monitoring all interactions. For organizations requiring advanced capabilities like CRM integration and custom compliance workflows, our subscription plans start at just 30USD monthly. Discover how Callin.io can transform your financial crime prevention efforts while improving customer experience and operational efficiency. Explore Callin.io today and take the first step toward a more secure, efficient anti-money laundering program.
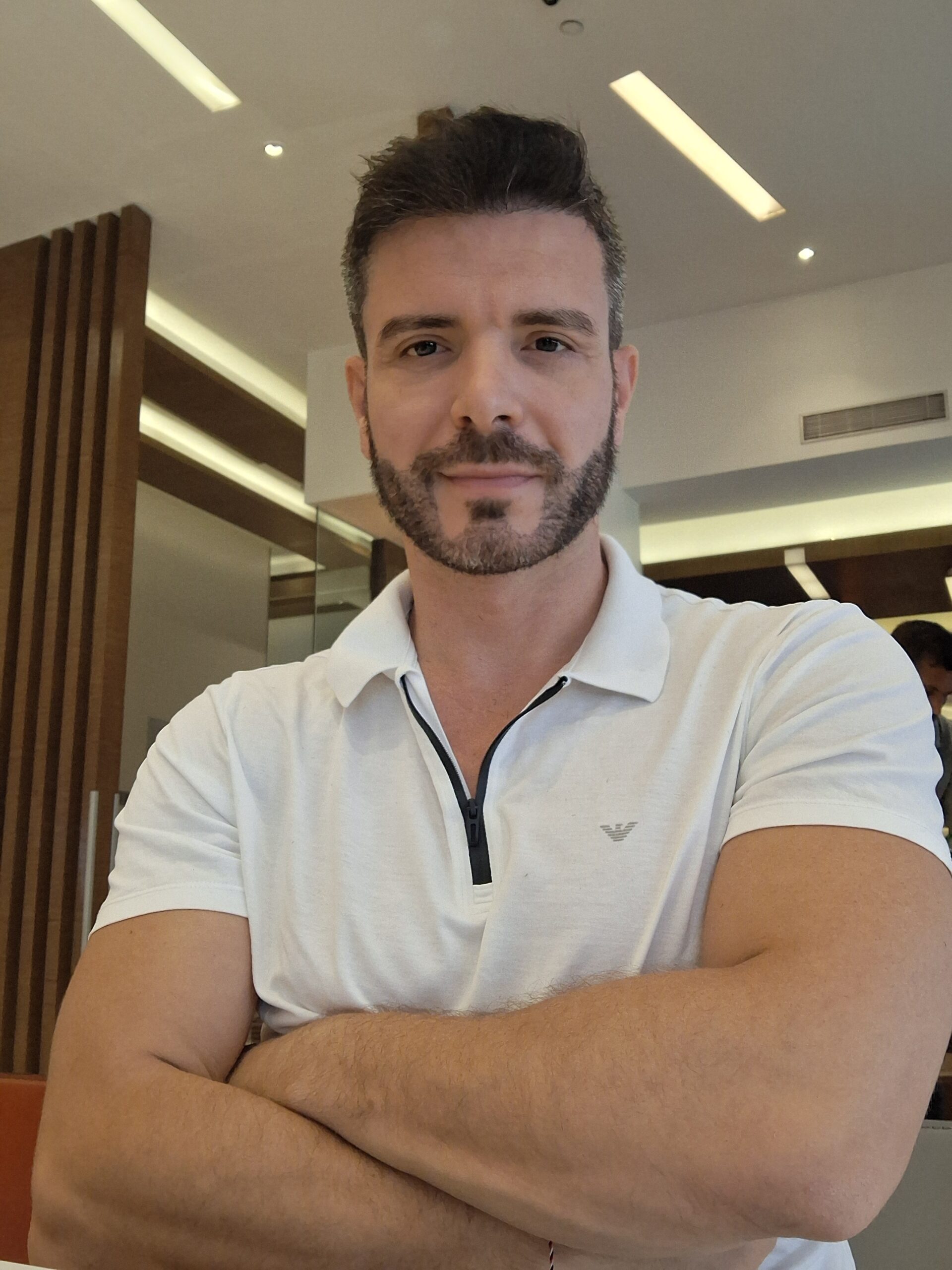
Helping businesses grow faster with AI. 🚀 At Callin.io, we make it easy for companies close more deals, engage customers more effectively, and scale their growth with smart AI voice assistants. Ready to transform your business with AI? 📅 Let’s talk!
Vincenzo Piccolo
Chief Executive Officer and Co Founder