The Evolution of AI in IT: A Historical Perspective
Artificial Intelligence has undergone a remarkable evolution within the information technology sector over the past several decades. What began as theoretical concepts in the 1950s has transformed into practical applications that now permeate virtually every aspect of modern IT infrastructure. The journey from simple rule-based systems to today’s sophisticated neural networks represents one of the most significant technological progressions in human history. Early AI systems were limited by computational constraints and narrow functionality, but the exponential growth in processing power coupled with breakthroughs in machine learning algorithms has catalyzed a revolution. This evolution didn’t happen overnight – it required persistent research, technological innovation, and visionary thinking from pioneers in both academia and industry. Organizations like MIT’s Computer Science and Artificial Intelligence Laboratory and companies such as IBM have been instrumental in pushing these boundaries, creating the foundation for today’s AI-powered information technology landscape where conversational AI for medical offices and other specialized applications are becoming commonplace.
Core Technologies Driving AI in Modern IT Systems
The remarkable capabilities of modern AI systems in information technology are powered by a constellation of core technologies working in concert. Deep learning stands at the forefront, utilizing multi-layered neural networks that can identify complex patterns in vast datasets with unprecedented accuracy. Natural Language Processing (NLP) enables computers to understand, interpret, and generate human language with increasing sophistication, forming the backbone of technologies like AI voice assistants. Computer vision systems now allow machines to "see" and interpret visual information with accuracy approaching or sometimes exceeding human capabilities. Meanwhile, reinforcement learning algorithms enable AI systems to optimize their performance through trial and error without explicit programming. These technologies don’t operate in isolation but rather form an interconnected ecosystem that continues to grow more sophisticated. The integration of these core technologies has enabled applications ranging from AI call centers to predictive analytics platforms that deliver actionable business intelligence. As noted by research from Stanford’s Human-Centered AI Institute, these technologies continue to advance at an accelerating pace, constantly pushing the boundaries of what’s possible in information technology.
AI-Powered Analytics: Transforming Data into Strategic Insights
In today’s data-driven business environment, AI-powered analytics represents perhaps the most transformative implementation of artificial intelligence within information technology frameworks. Organizations are now capable of processing petabytes of structured and unstructured data to extract meaningful insights that drive strategic decision-making. Unlike traditional analytics approaches, AI-powered systems can identify subtle patterns and correlations that might otherwise remain hidden to human analysts. These systems continuously learn from new data inputs, progressively refining their analytical models to deliver increasingly precise insights. The business impact is substantial – from predictive maintenance in manufacturing that prevents costly downtime to sophisticated customer behavior analysis that enables hyper-personalized marketing campaigns. Healthcare organizations are leveraging these capabilities to improve patient outcomes through predictive analytics, while financial institutions use them for risk assessment and fraud detection. The democratization of these tools through cloud-based platforms has made advanced analytics accessible even to smaller organizations without dedicated data science teams. As highlighted by Gartner’s research on AI in analytics, companies implementing AI-powered analytics consistently outperform competitors by making faster, more informed decisions based on comprehensive data analysis rather than intuition.
Conversational AI: Revolutionizing User Interactions
The rise of conversational AI represents one of the most visible manifestations of artificial intelligence in information technology, fundamentally changing how humans interact with digital systems. Modern conversational AI platforms combine natural language understanding, context awareness, and machine learning to create increasingly natural and effective interactions. These systems have evolved dramatically from simple rule-based chatbots to sophisticated platforms capable of understanding nuance, remembering context across conversations, and continuously improving through interactions. The business applications are diverse and growing rapidly – from AI phone agents that can handle customer inquiries with human-like understanding to virtual receptionists that manage appointment scheduling and basic customer service. In healthcare, conversational AI for medical offices is streamlining patient interactions and administrative workflows. The technology has become sophisticated enough that many users cannot distinguish between AI and human agents in certain contexts. This evolution is creating significant efficiency gains for organizations while simultaneously improving user satisfaction by providing immediate, 24/7 assistance. According to research from MIT Technology Review, organizations implementing conversational AI are seeing customer satisfaction improvements averaging 20% alongside significant operational cost reductions.
AI-Enhanced Cybersecurity: The New Frontier in Digital Protection
As digital threats grow in sophistication and scale, artificial intelligence has become an indispensable component of modern cybersecurity frameworks within information technology departments. AI-powered security systems offer capabilities that traditional rule-based approaches simply cannot match in today’s threat landscape. These systems excel at analyzing vast quantities of network traffic and user behavior to establish baseline patterns, then identifying anomalies that may indicate security breaches or attack attempts. The most advanced implementations utilize unsupervised learning to discover previously unknown threat patterns, essentially anticipating attack vectors before they’re widely exploited. This proactive approach represents a paradigm shift from the reactive security postures that dominated previous generations of IT security. Major corporations and government agencies are now deploying AI-based security operations centers that combine human expertise with artificial intelligence to create multilayered defense systems of unprecedented effectiveness. These integrated approaches are particularly valuable against sophisticated threats like advanced persistent threats (APTs) and zero-day exploits. The National Institute of Standards and Technology has recognized the transformative potential of AI in cybersecurity, publishing frameworks to guide implementation. For businesses looking to strengthen their security posture while managing costs, AI-based call center solutions incorporate security features that protect sensitive customer interactions.
Cloud Computing and AI: A Symbiotic Relationship
The relationship between cloud computing and artificial intelligence represents one of the most powerful technological synergies in modern information technology. Cloud platforms provide the scalable computing resources and storage capabilities that make advanced AI implementations feasible for organizations of all sizes. This democratization effect has accelerated AI adoption across industries by removing the capital expense barriers that previously limited sophisticated AI deployments to only the largest enterprises. Simultaneously, AI enhances cloud platforms through intelligent resource allocation, predictive maintenance, and automated optimization of complex cloud environments. The major cloud providers—AWS, Microsoft Azure, and Google Cloud Platform—have made AI services central to their offerings, providing pre-built models and development environments that simplify implementation. This convergence has enabled the rise of AI-as-a-Service (AIaaS) models where organizations can leverage sophisticated AI capabilities without building systems from scratch. The flexibility of cloud-based AI allows for rapid experimentation and iteration, significantly reducing the time-to-value for new AI initiatives. According to Deloitte’s research on cloud AI adoption, organizations leveraging cloud-based AI solutions are implementing production AI systems 2-3 times faster than those building on-premises solutions. For businesses exploring practical applications like AI appointment scheduling, cloud-based platforms provide the ideal foundation.
The Impact of AI on IT Infrastructure Management
Artificial intelligence is fundamentally transforming IT infrastructure management, introducing unprecedented levels of automation and predictive capability to operations that were previously manual and reactive. Modern AI systems can continuously monitor thousands of performance metrics across complex IT environments, identifying patterns that precede failures and automatically implementing corrective actions before users experience disruption. This shift from reactive to predictive operations represents a quantum leap in IT service delivery capabilities. AIOps platforms now integrate with traditional IT service management tools to create intelligent operational environments that significantly reduce mean time to resolution for incidents while simultaneously decreasing the total incident volume through preventive measures. Network operations have been particularly transformed, with AI systems dynamically optimizing traffic flows, security policies, and resource allocation based on real-time demands. The cost implications are substantial—organizations implementing comprehensive AI-driven infrastructure management typically report operational cost reductions of 15-40% according to Gartner research. These efficiencies extend beyond cost savings to improved service quality and reliability, creating competitive advantages for early adopters. For businesses looking to leverage AI in customer-facing operations, solutions like AI phone services can be seamlessly integrated with existing infrastructure through intelligent management platforms.
Ethics and Governance of AI in Information Technology
As artificial intelligence becomes increasingly embedded in information technology systems, questions of ethics and governance have moved from theoretical discussions to practical imperatives. Organizations must now navigate complex ethical considerations including algorithmic bias, privacy implications, transparency of AI decision-making, and appropriate human oversight of autonomous systems. These concerns are particularly acute in applications where AI makes decisions that directly impact individuals, such as in hiring processes, credit determinations, or medical diagnosis assistance. Responsible AI frameworks have emerged as structured approaches to addressing these concerns, incorporating principles like fairness, accountability, transparency, and explainability. Leading technology companies and research institutions have developed governance models that balance innovation with ethical considerations while complying with evolving regulatory requirements. The European Commission’s Ethics Guidelines for Trustworthy AI has become an influential reference point for organizations worldwide. These governance frameworks incorporate technical safeguards, organizational processes, and continuous monitoring to ensure AI systems operate as intended and align with societal values. For businesses implementing customer-facing AI systems like AI voice conversations, adherence to ethical guidelines is essential for maintaining customer trust and regulatory compliance.
AI Skills Gap: The Challenge for IT Professionals
The rapid integration of artificial intelligence into information technology has created a significant skills gap that represents both a challenge for organizations and an opportunity for IT professionals. The demand for AI specialists with expertise in machine learning, data science, and AI implementation far exceeds the current supply, creating competitive recruitment landscapes and salary premiums for qualified professionals. This skills shortage is consistently cited as one of the primary barriers to AI adoption in enterprise environments. Organizations are responding through multiple approaches, including partnering with academic institutions, creating internal AI training programs, and leveraging AI platforms that reduce the specialized knowledge required for implementation. The skills requirements extend beyond purely technical capabilities to include understanding of ethical implications, business process integration, and change management associated with AI adoption. For existing IT professionals, the AI revolution necessitates continuous learning and adaptation, with roles increasingly focusing on the strategic application of AI rather than system management tasks that are becoming automated. According to IBM’s research on AI skills, over 120 million workers globally will need retraining or skills development as a result of AI and automation in the next three years. For businesses exploring AI implementation but concerned about internal capabilities, solutions like white label AI receptionists offer a path to adoption without requiring specialized in-house expertise.
AI-Driven Automation in IT Service Delivery
The implementation of AI-driven automation is transforming IT service delivery models across industries, creating unprecedented efficiency and scalability in operations previously constrained by human capacity. Intelligent automation now handles everything from basic helpdesk ticket routing to complex infrastructure provisioning, dramatically reducing fulfillment times while improving consistency and compliance. Robotic Process Automation (RPA) enhanced with AI capabilities has moved beyond simple task automation to intelligent process optimization that can adapt to changing conditions without human intervention. Service management platforms now incorporate predictive intelligence that can anticipate user needs, proactively address potential issues, and continuously optimize service delivery based on usage patterns and feedback. The most advanced implementations create self-healing IT environments where systems can detect, diagnose, and resolve many common issues without human intervention. For users, this translates to more responsive IT services with significantly reduced resolution times. Organizations implementing comprehensive AI-driven automation typically report 40-60% reductions in service fulfillment times and 30-50% decreases in service delivery costs according to McKinsey’s research on AI in IT operations. For businesses seeking practical applications, solutions like AI call assistants demonstrate how automation can transform customer interactions while reducing operational costs.
Edge AI: Intelligence at the Network Periphery
The convergence of artificial intelligence with edge computing represents one of the most significant architectural shifts in information technology, moving computational intelligence closer to data sources rather than centralizing processing in cloud environments. This evolution addresses critical limitations in traditional cloud-based AI models, including latency constraints, bandwidth limitations, privacy concerns, and connectivity requirements. Edge AI implementations enable real-time processing and decision-making at or near the point of data collection, opening new possibilities for applications ranging from autonomous vehicles to smart manufacturing systems that cannot tolerate the delays associated with cloud round-trips. The technology is particularly transformative for Internet of Things (IoT) deployments, where the volume of generated data makes centralized processing impractical or prohibitively expensive. Major technology providers are developing specialized hardware and software stacks optimized for AI workloads at the edge, including energy-efficient neural processing units and distributed training frameworks. This architectural approach also enhances privacy by enabling data processing without transmitting sensitive information to centralized repositories. According to IDC research on edge AI adoption, the market for edge AI hardware and software is growing at over 25% annually, reflecting the transformative potential of these technologies. For businesses exploring practical applications, solutions like AI voice agents demonstrate how intelligent processing can be deployed at the network edge to deliver responsive customer interactions.
AI and DevOps: Accelerating Software Development Cycles
The integration of artificial intelligence into DevOps practices is revolutionizing software development and deployment processes, creating what industry leaders now term "AIOps" or AI-enhanced DevOps. This convergence is addressing the fundamental challenge of developing, testing, and deploying software at the speed required by modern business while maintaining quality and reliability. AI algorithms now assist developers through intelligent code completion, automated bug detection, and predictive testing that identifies potential issues before they impact users. Automated code review systems powered by machine learning can analyze thousands of code changes daily, identifying potential security vulnerabilities and quality issues with accuracy exceeding traditional approaches. The deployment phase has been similarly transformed, with AI systems monitoring releases in real-time, automatically detecting anomalies, and in advanced implementations, autonomously rolling back problematic deployments before significant user impact occurs. For operations teams, AI provides predictive capacity planning that anticipates resource requirements based on historical patterns and projected growth, ensuring optimal performance during peak demand periods. According to research from DevOps Research and Assessment (DORA), organizations implementing comprehensive AIOps practices deliver software updates 200% more frequently with 70% lower change failure rates compared to traditional approaches. For businesses looking to improve operational efficiency, AI-powered call center solutions demonstrate how these principles can be applied to customer service operations.
Natural Language Processing in Enterprise IT
The maturation of Natural Language Processing (NLP) is creating transformative capabilities within enterprise IT environments, enabling more intuitive and efficient interactions between humans and technology systems. Advanced NLP algorithms now power a range of applications that understand, interpret, and generate human language with remarkable accuracy. Conversational interfaces have evolved from novelties to production-grade business tools, enabling employees to interact with complex enterprise systems using natural language rather than learning specialized interfaces. Document processing has been revolutionized by NLP capabilities that can automatically extract, categorize, and summarize information from unstructured documents, transforming mountains of text into searchable, analyzable data assets. In customer service applications, NLP enables systems like AI phone calls that can understand customer inquiries, provide appropriate responses, and escalate complex issues when necessary. The technology has advanced sufficiently that many enterprise NLP deployments now include sentiment analysis capabilities that can detect emotional nuances in text and speech, enabling more appropriate responses to customer or employee communications. According to Gartner’s analysis of NLP in enterprise, organizations implementing comprehensive NLP strategies are seeing productivity improvements of 15-30% in document-intensive processes while significantly improving employee and customer satisfaction through more natural interaction models.
AI-Enhanced Decision Support Systems in IT Management
Artificial intelligence has fundamentally transformed decision support systems within IT management, creating platforms that augment human judgment with data-driven insights of unprecedented depth and accuracy. These systems aggregate and analyze vast quantities of operational data, identifying patterns and correlations that would be impossible for human analysts to discover independently. Cognitive decision support platforms now provide IT leaders with scenario modeling capabilities that can simulate the potential impacts of strategic decisions before implementation, significantly reducing risk in complex transformational initiatives. Resource allocation has been particularly transformed, with AI systems optimizing the distribution of financial and human resources across multiple competing priorities based on organizational goals and constraints. In crisis situations, these systems can provide real-time decision support by instantly analyzing similar historical incidents and recommending response strategies with the highest probability of success. According to Harvard Business Review research on AI in decision making, organizations that effectively leverage AI-enhanced decision support report 20-30% improvements in decision quality and 60% reductions in decision time compared to traditional approaches. For businesses exploring practical applications, AI voice agent whitelabel solutions demonstrate how decision support capabilities can be embedded in customer-facing systems to improve response quality while maintaining brand identity.
AI in IT Security Operations Centers
The integration of artificial intelligence into Security Operations Centers (SOCs) represents a quantum leap in cybersecurity defense capabilities within information technology environments. Modern threat landscapes generate volumes of security data that far exceed human analytical capacity, creating an environment where AI-augmented security has become a necessity rather than a luxury. AI-enhanced security information and event management (SIEM) platforms now correlate events across thousands of data sources, applying machine learning to distinguish genuine threats from benign anomalies with unprecedented accuracy. User and entity behavior analytics (UEBA) powered by AI establishes baseline activity patterns for users and systems, then identifies deviations that may indicate compromise even when traditional signature-based systems would detect nothing unusual. Threat hunting has been transformed from a largely manual process to an AI-augmented discipline where human analysts partner with intelligent systems to proactively seek out sophisticated adversaries within networks. The most advanced implementations now incorporate autonomous response capabilities that can take defensive actions against identified threats within milliseconds, containing potential damage before human analysts could even begin to respond. According to Ponemon Institute research on AI in cybersecurity, organizations with AI-augmented security operations detect and contain breaches 60% faster than those using traditional approaches, with average cost savings of $3.5 million per breach. For businesses implementing customer-facing systems, solutions like Twilio AI assistants incorporate security features that protect sensitive customer data during interactions.
Cognitive Computing in Enterprise IT Architecture
Cognitive computing represents the next evolutionary step beyond traditional AI approaches in enterprise IT architecture, creating systems that can mimic human thought processes including reasoning, learning, and self-correction. Unlike conventional AI that excels at specific, well-defined tasks, cognitive systems can handle ambiguity, understand context, and adapt to changing information environments. Enterprise knowledge graphs powered by cognitive computing now serve as the foundation for organizational intelligence across multiple applications, connecting disparate information sources into unified knowledge representations that become more valuable over time. These systems excel at contextual understanding—recognizing not just keywords but the broader meaning and implications of information within specific business contexts. Decision support applications built on cognitive platforms can evaluate multiple competing hypotheses simultaneously, providing nuanced recommendations that consider ethical implications, risk factors, and business constraints. The technology demonstrates particular value in complex regulatory environments where rules frequently change and have intricate interdependencies. According to IBM Research on cognitive computing, organizations implementing comprehensive cognitive computing strategies report 40% improvements in knowledge worker productivity and 50% reductions in time spent searching for information. For businesses exploring practical applications, AI cold calling solutions demonstrate how cognitive capabilities can transform outbound sales by understanding prospect needs and adapting conversations in real-time.
Quantum Computing and AI: The Next Frontier
The convergence of quantum computing and artificial intelligence represents the horizon of innovation in information technology, promising computational capabilities that could fundamentally transform what’s possible in AI systems. Quantum computers leverage quantum mechanical phenomena such as superposition and entanglement to perform calculations that would be practically impossible for classical computers, particularly in domains like optimization, simulation, and certain types of pattern recognition that are foundational to advanced AI. Quantum machine learning algorithms are being developed that could exponentially accelerate training for complex neural networks, potentially reducing processes that currently take weeks to minutes or seconds. While practical quantum computing at scale remains several years away, major technology companies and research institutions are already developing quantum-ready AI frameworks that can begin leveraging these capabilities as quantum hardware matures. The potential applications span virtually every industry—from discovering new pharmaceutical compounds through quantum-accelerated molecular modeling to optimizing global logistics networks with previously impossible precision. According to McKinsey’s analysis of quantum computing impact, the combination of quantum computing with AI could create between $450 billion and $850 billion in value annually by 2040. Organizations exploring the practical implementation of AI should consider how their strategies might eventually leverage quantum capabilities, even as they focus on immediate value creation through solutions like AI phone numbers and conversational AI platforms.
AI and the Future of IT Workforce
The integration of artificial intelligence into information technology is fundamentally reshaping the IT workforce, simultaneously eliminating certain job categories while creating entirely new roles that didn’t exist a decade ago. This transformation requires both individual professionals and organizations to adapt their skills development and workforce planning strategies to remain relevant. AI augmentation rather than wholesale replacement has emerged as the dominant paradigm, with the most successful implementations pairing human creativity and judgment with AI-driven analytics and automation. New job categories including AI ethics officers, machine learning operations specialists, and human-AI collaboration designers are emerging to address the unique challenges of managing intelligent systems. IT education is evolving in response, with increasing emphasis on uniquely human skills such as critical thinking, creative problem-solving, and ethical decision-making alongside technical capabilities. According to World Economic Forum research on AI and employment, while AI will displace approximately 85 million jobs by 2025, it will simultaneously create 97 million new roles—representing a net positive but requiring significant workforce transition. For businesses navigating this changing landscape, solutions like AI call center white label offerings provide paths to modernization that complement rather than replace human capabilities.
Regulatory Frameworks for AI in Information Technology
As artificial intelligence becomes increasingly embedded in critical information technology systems, regulatory frameworks are evolving worldwide to address the unique challenges these technologies present. Organizations implementing AI must navigate a complex and rapidly changing compliance landscape that varies significantly by geography, industry, and application type. AI governance regulations are focusing on core concerns including privacy protection, algorithmic transparency, accountability for automated decisions, and requirements for human oversight of critical AI systems. The European Union’s AI Act represents the most comprehensive regulatory approach to date, establishing a risk-based framework that imposes different obligations based on the potential impact of specific AI applications. In the United States, a sector-specific approach has emerged with different regulations for AI in healthcare, financial services, and other regulated industries. These frameworks create both compliance challenges and competitive opportunities for organizations that effectively integrate regulatory considerations into their AI strategies. According to Deloitte’s analysis of AI regulation, organizations that proactively address regulatory requirements in their AI implementations achieve faster approval cycles and higher user acceptance rates than those taking reactive approaches. For businesses implementing customer-facing AI systems, solutions like prompt engineering for AI callers enable compliance with regulatory requirements while maintaining effective customer interactions.
Measuring ROI from AI Investments in IT
As artificial intelligence moves from experimental projects to production implementations in information technology, organizations face the challenge of accurately measuring return on investment from these initiatives. Traditional ROI models often prove inadequate for AI investments, which typically deliver value through multiple mechanisms including cost reduction, productivity enhancement, quality improvement, and entirely new capabilities. AI valuation frameworks now incorporate both quantitative metrics like reduced operating costs or increased revenue and qualitative benefits such as improved decision quality or enhanced customer experience. The most effective approaches establish clear baseline measurements before AI implementation, then track incremental improvements across multiple dimensions. Organizations are increasingly recognizing that AI ROI should be measured at the portfolio rather than individual project level, as some initiatives will inevitably deliver lower returns while establishing foundational capabilities for future high-value applications. According to MIT Sloan Management Review research on AI ROI, organizations with mature AI ROI measurement frameworks achieve 3-5 times greater business value from their AI investments than those using traditional evaluation approaches. For businesses considering practical implementations, solutions like AI appointment setters demonstrate measurable ROI through reduced staffing requirements, extended service hours, and improved conversion rates.
Elevate Your Business Communication with AI-Powered Solutions
The integration of artificial intelligence into information technology has created unprecedented opportunities for businesses to transform their operations, enhance customer experiences, and gain competitive advantages. The technologies discussed throughout this article represent not just theoretical possibilities but practical tools that organizations of all sizes can leverage today. By implementing solutions like AI voice conversations and AI call centers, businesses can simultaneously improve service quality while reducing operational costs. If you’re ready to harness the power of AI in your communication strategy, Callin.io offers a comprehensive platform for implementing AI-powered phone agents that can handle inbound and outbound calls autonomously. Their solution enables you to automate appointments, answer frequently asked questions, and even close sales through natural-sounding AI interactions. With Callin.io’s free account, you can access an intuitive interface for configuring your AI agent, with test calls included and a task dashboard to monitor interactions. For advanced features like Google Calendar integration and CRM connectivity, subscription plans start at just $30 per month. Discover how Callin.io can transform your business communications by visiting their website today.
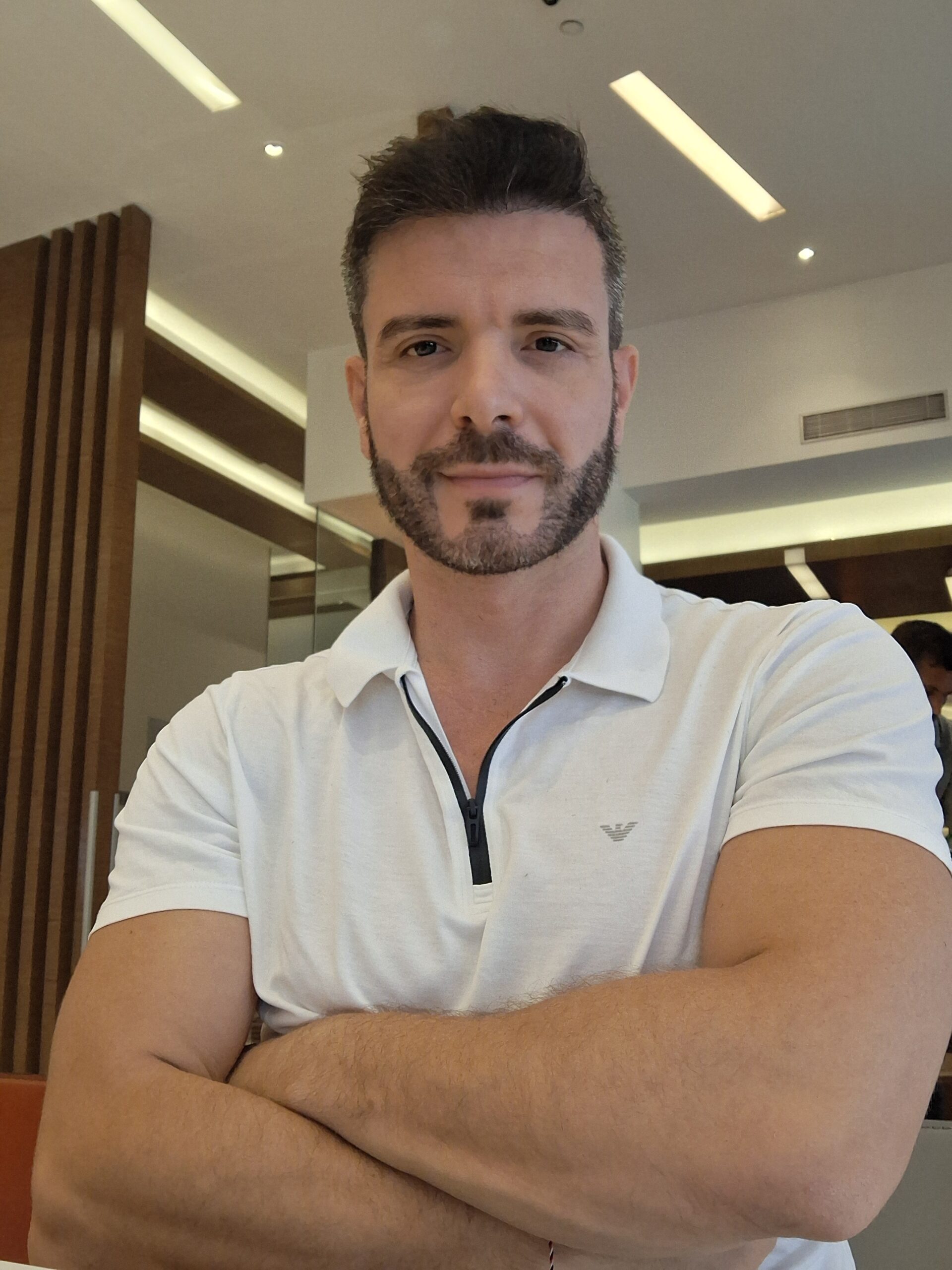
Helping businesses grow faster with AI. 🚀 At Callin.io, we make it easy for companies close more deals, engage customers more effectively, and scale their growth with smart AI voice assistants. Ready to transform your business with AI? 📅 Let’s talk!
Vincenzo Piccolo
Chief Executive Officer and Co Founder