The Digital Conversation Revolution
In today’s fast-paced digital marketplace, conversational AI tools have transformed how businesses interact with customers. The choice between specialized AI chatbots and ChatGPT represents a critical decision for companies seeking to enhance customer engagement. This distinction isn’t merely academic—it impacts efficiency, cost, and the overall customer experience. AI chatbots have evolved from simple rule-based systems to sophisticated conversation partners, while ChatGPT has emerged as a versatile language model capable of handling diverse interactions. Organizations now face the challenge of determining which solution best addresses their specific communication needs, considering factors like customization requirements, technical complexity, and integration capabilities. As conversational AI for medical offices demonstrates, the stakes are particularly high in specialized industries where accuracy and domain knowledge are paramount.
Understanding AI Chatbots: Purpose-Built Conversation Partners
AI chatbots represent purpose-designed conversation systems built to handle specific tasks within defined parameters. These specialized digital assistants excel in structured environments where the conversation paths are predictable and domain-specific. Unlike general-purpose AI, chatbots are typically engineered with particular business objectives in mind, such as appointment scheduling, customer support for specific products, or lead qualification in sales processes. Their strength lies in their focused approach—they’re optimized for efficiency in narrow applications, making them particularly valuable for businesses with clearly defined customer interaction needs. The technical architecture of chatbots often combines rule-based logic with machine learning components, allowing them to handle variations in user queries while maintaining consistent performance in their area of expertise. For instance, AI appointment schedulers represent a specialized implementation that demonstrates how chatbots can excel when built for specific functions.
ChatGPT: The Versatile Language Powerhouse
ChatGPT represents a fundamentally different approach to conversational AI. Developed by OpenAI, this large language model (LLM) leverages a massive neural network trained on diverse internet text to generate human-like responses across an extraordinary range of topics. Unlike traditional chatbots, ChatGPT wasn’t designed for any single task but instead possesses remarkable general-purpose capabilities that allow it to discuss philosophy, write code, explain complex concepts, or engage in casual conversation with equal facility. This versatility stems from its foundation as a text prediction engine that has absorbed patterns from billions of text examples, enabling it to generate contextually appropriate responses to virtually any prompt. ChatGPT’s ability to understand nuance, maintain context over extended conversations, and adapt to different communication styles has made it valuable across industries. Many businesses now integrate ChatGPT through AI voice assistants that combine language understanding with speech capabilities.
Technical Architecture: How They’re Built Differently
The architectural differences between standard AI chatbots and ChatGPT reveal fundamental distinctions in their design philosophy. Traditional chatbots typically employ a hybrid approach combining predefined conversation flows with natural language processing (NLP) components. These systems often use intent recognition algorithms to classify user queries, entity extraction to identify key information, and dialog management systems that track conversation state. In contrast, ChatGPT utilizes a transformer-based neural network architecture trained through both supervised learning and reinforcement learning from human feedback (RLHF). This architecture enables ChatGPT to process input tokens in parallel rather than sequentially, allowing it to maintain context across extended conversations. While specialized chatbots often incorporate business logic directly into their design, ChatGPT requires careful prompting and fine-tuning to adapt its general capabilities to specific business contexts. The implementation of these systems also differs—chatbots frequently connect to business databases and APIs directly, while ChatGPT integrations require additional engineering to bridge the gap between general language capabilities and specific business functions, as seen in AI calling solutions for businesses.
Use Case Specificity: Matching Tools to Tasks
When evaluating AI chatbots versus ChatGPT, use case alignment becomes a critical consideration. Specialized chatbots demonstrate superior performance in tightly defined scenarios where conversation paths follow predictable patterns. For example, an AI call center assistant designed specifically for appointment scheduling can masterfully handle time slots, rescheduling requests, and reminder confirmations with precision and efficiency. These purpose-built systems excel when the conversation scope remains within clearly defined parameters. Conversely, ChatGPT offers remarkable flexibility across unpredictable conversation territories, making it ideal for customer service scenarios requiring wide-ranging knowledge, creative problem-solving, or nuanced understanding of complex queries. Its ability to adapt to unexpected questions makes ChatGPT valuable for general customer support, content creation, or situations where conversation paths cannot be fully anticipated. However, this versatility sometimes comes at the cost of precision in highly specialized domains. According to research from Stanford’s Human-Centered AI Institute, task-specific chatbots consistently outperform general models in narrow applications, particularly in regulated industries like healthcare and financial services.
Cost Considerations: Budget Impact Analysis
The financial implications of choosing between AI chatbots and ChatGPT warrant careful consideration for businesses of all sizes. Purpose-built chatbots typically involve higher upfront development costs but offer predictable operational expenses once deployed. These systems generally operate with fixed pricing structures based on conversation volume or user counts. For example, implementing an AI voice agent for a specific business function might require initial investment in design and training, but ongoing costs remain stable. ChatGPT deployments, conversely, typically follow consumption-based pricing models that charge for API calls or token usage. While this approach eliminates upfront development expenses, costs can rapidly escalate with increased usage, particularly for applications generating substantial conversation volume. Small businesses with specific, limited-scope requirements may find specialized chatbots more cost-effective long-term, while enterprises requiring flexibility across multiple departments might benefit from ChatGPT’s versatility despite potentially higher variable costs. A 2023 analysis by Gartner Research indicates that organizations implementing specialized chatbots for high-volume, routine interactions typically achieve cost savings of 30-40% compared to general-purpose AI solutions.
Customization Capabilities: Tailoring to Your Brand Voice
Establishing a consistent brand voice represents a crucial aspect of customer experience strategy, and the customization capabilities of different AI conversation systems significantly impact this dimension. Specialized AI chatbots offer extensive customization options, allowing businesses to precisely define conversation flows, response styles, and personality attributes that align with their brand identity. These systems can be meticulously programmed to use specific terminology, maintain particular tones across interactions, and even adjust communication styles based on customer segments or conversation contexts. Organizations implementing white label AI receptionists particularly benefit from this level of control. ChatGPT, while remarkably versatile, presents greater challenges in maintaining consistent brand voice across varied interactions. Though the model can be guided through careful prompting and fine-tuning, achieving the precise calibration of specialized chatbots typically requires more sophisticated engineering and ongoing prompt management. For businesses where brand consistency represents a critical competitive differentiator, the granular control offered by purpose-built chatbots often provides significant advantages, especially in customer-facing applications where tone and terminology directly impact perception and trust.
Integration Complexity: Connecting with Your Tech Stack
The practical implementation of AI conversation systems hinges significantly on their ability to seamlessly connect with existing business infrastructure. Specialized AI chatbots are typically designed with integration capabilities as a core feature, offering standardized connectors for common business systems like CRM platforms, appointment scheduling tools, inventory management systems, and payment processors. These purpose-built solutions often include pre-configured API endpoints specifically designed for business applications. For example, AI call center solutions frequently offer direct integration with telephony systems, customer databases, and ticketing platforms. ChatGPT implementations, by contrast, generally require more extensive custom development to bridge the gap between the language model’s capabilities and specific business systems. While OpenAI provides APIs for accessing ChatGPT functionality, substantial engineering work is typically necessary to transform its general language capabilities into actionable business processes connected to existing infrastructure. Organizations with limited technical resources may find specialized chatbots’ ready-made integration features more accessible, while enterprises with robust development teams can leverage ChatGPT’s flexibility through custom integration frameworks. According to IBM’s AI implementation research, integration complexity represents the second most significant challenge in deploying conversational AI solutions across enterprises.
Training Requirements and Learning Curves
The knowledge acquisition process differs fundamentally between specialized AI chatbots and ChatGPT, creating distinct implications for implementation timelines and performance. Purpose-built chatbots typically require explicit training on domain-specific knowledge, conversational patterns, and business rules. This structured learning approach demands significant upfront investment in knowledge engineering, conversation design, and scenario testing. However, once properly configured, these systems demonstrate consistent performance within their defined domains. Organizations implementing AI phone services often appreciate this predictability in customer-facing applications. ChatGPT, conversely, arrives pre-trained on vast amounts of general knowledge but requires fine-tuning and careful prompting to perform optimally in specific business contexts. While this approach reduces initial knowledge engineering requirements, it necessitates ongoing prompt optimization and potentially introduces variability in responses. The learning curve for managing ChatGPT effectively involves mastering prompt engineering techniques rather than traditional training methodologies. A study by MIT Technology Review found that organizations implementing specialized chatbots typically reach optimal performance within 3-6 months, while ChatGPT deployments continue showing performance improvements for 9-12 months as prompt engineering expertise develops.
Handling Multi-Turn Conversations: Depth and Context
The ability to maintain context across extended interactions represents a critical dimension in evaluating conversational AI solutions. Traditional AI chatbots have historically struggled with context maintenance across multiple conversation turns, often requiring users to repeat information or restart interactions when conversations become complex. While modern specialized chatbots have improved in this regard through sophisticated state tracking mechanisms, they typically excel in conversations with predictable structures rather than free-flowing exchanges. ChatGPT, by contrast, demonstrates remarkable capabilities for maintaining context across lengthy, multi-turn conversations. Its transformer architecture enables the model to reference earlier parts of the conversation when generating responses, creating more natural and coherent interactions. This capability proves particularly valuable in complex customer support scenarios, sales processes requiring nuanced needs discovery, or situations where contextual understanding significantly impacts outcome quality. For businesses implementing AI voice conversations, this context maintenance capability directly influences customer satisfaction and resolution effectiveness. Research from the Association for Computational Linguistics indicates that context retention in extended conversations represents the strongest predictor of customer satisfaction with AI interaction systems.
Industry-Specific Applications: Sector Analysis
Different industries present unique conversational requirements that influence the suitability of various AI approaches. In healthcare settings, specialized chatbots often excel due to their ability to incorporate strict compliance frameworks, clinical terminology, and precise procedural knowledge. These systems, like those described in conversational AI for medical offices, can be meticulously calibrated to follow healthcare regulations while handling specific functions like appointment scheduling or medication reminders. Financial services similarly benefit from specialized chatbots’ precise handling of transaction processes, compliance requirements, and security protocols. Conversely, industries with diverse customer inquiries often find value in ChatGPT’s flexibility. Retail and e-commerce businesses frequently leverage ChatGPT’s ability to discuss product features, make recommendations, and address varied customer concerns without requiring exhaustive scenario programming. Creative industries like marketing and content creation benefit from ChatGPT’s language generation capabilities for idea development and draft creation. Legal services represent an interesting hybrid case—while specialized chatbots excel in structured processes like document filing, ChatGPT’s comprehension of complex legal concepts makes it valuable for preliminary case assessment and research assistance. The International Association for Artificial Intelligence and Law notes that 78% of legal services now employ dual systems—structured chatbots for procedural matters and generative AI for conceptual analysis.
Security and Compliance Considerations
Data protection, regulatory compliance, and privacy safeguards represent critical evaluation factors when selecting conversational AI solutions. Specialized AI chatbots designed for regulated industries typically incorporate compliance frameworks directly into their architecture. These purpose-built systems often process data within controlled environments, maintain detailed audit trails, and implement industry-specific security protocols. For example, chatbots developed for financial institutions incorporate robust authentication mechanisms and PCI-DSS compliance features. Healthcare implementations, like those discussed in AI calling solutions for health clinics, build HIPAA compliance directly into their conversation handling. ChatGPT implementations present distinct security considerations. While OpenAI has strengthened its data handling practices, organizations must carefully engineer their ChatGPT integrations to ensure sensitive information receives appropriate protection. This often involves implementing additional security layers, data filtering mechanisms, and careful API configuration to maintain compliance requirements. For highly regulated industries, specialized chatbots frequently offer more straightforward compliance pathways, while ChatGPT deployments typically require more extensive security engineering. According to the National Institute of Standards and Technology, organizations should conduct thorough Data Protection Impact Assessments (DPIAs) before implementing any conversational AI system handling regulated information.
Accuracy and Error Handling Mechanisms
Reliability in information delivery and graceful management of misunderstandings significantly influences conversational AI effectiveness. Specialized AI chatbots excel in accuracy within their defined knowledge domains, delivering precise, verified information about specific products, services, or procedures. These systems typically incorporate explicit error handling pathways, recognizing common misunderstandings and providing structured remediation options. For customer-facing applications like AI sales representatives, this predictable accuracy within defined parameters enhances trust and reduces transaction abandonment. ChatGPT presents a more complex accuracy profile—while remarkably capable across diverse topics, it occasionally generates convincing-sounding but factually incorrect information (a phenomenon known as "hallucination"). These accuracy variations require implementation of verification mechanisms and careful system design to manage potential misinformation risks. However, ChatGPT demonstrates superior handling of unexpected queries, gracefully addressing questions outside typical conversation patterns rather than producing rigid "I don’t understand" responses. Research by the Association for Computing Machinery indicates that specialized chatbots typically achieve 92-98% accuracy within their designed domains but struggle with unexpected queries, while ChatGPT maintains 85-90% accuracy across broader topic ranges with greater adaptability to novel questions.
Scalability Across Business Functions
The ability to expand AI conversational capabilities across multiple business functions represents an important strategic consideration. Specialized AI chatbots excel in depth but often present challenges in breadth—extending their capabilities to new business areas typically requires significant additional development work. Organizations frequently find themselves managing multiple distinct chatbot implementations across different departments, creating potential inconsistencies in customer experience and increased maintenance complexity. Implementations like AI phone agents often focus on specific functional areas rather than enterprise-wide deployment. ChatGPT offers superior horizontal scalability, with the same underlying model capable of addressing diverse business functions from customer support to content creation, product recommendations, and technical assistance. This versatility enables organizations to implement a consistent conversation layer across multiple departments while tailoring prompts for specific functions. However, this approach sometimes sacrifices the deep domain expertise of specialized solutions. Enterprises increasingly adopt hybrid approaches, deploying specialized chatbots for critical high-volume functions while leveraging ChatGPT for broader, less predictable interaction scenarios. According to Forrester Research, organizations implementing coordinated conversational AI strategies across multiple departments report 26% higher customer satisfaction scores than those with fragmented implementations.
Personality and Customer Experience Impact
The perceived personality of AI conversation systems significantly influences customer experience, brand perception, and engagement metrics. Specialized AI chatbots offer precise control over personality attributes, enabling businesses to craft specific conversation styles that align with their brand positioning. These systems can be engineered to demonstrate particular character traits, communication preferences, and interaction styles consistently across all customer touchpoints. For applications like AI call assistants, this personality calibration directly impacts customer comfort and conversation effectiveness. ChatGPT demonstrates remarkable flexibility in adopting different personalities through prompt engineering, allowing it to shift from professional to casual, technical to approachable, or formal to conversational based on implementation requirements. However, maintaining consistent personality characteristics across diverse interactions with ChatGPT requires sophisticated prompt design and ongoing management. Research from the Customer Experience Professionals Association indicates that perceived warmth in AI conversations increases customer satisfaction by 37%, while perceived competence increases resolution rates by 42%—making personality engineering a crucial consideration regardless of the technical approach selected.
Real-Time Learning and Adaptation
The capacity for continuous improvement through interaction data represents an important differentiator between conversational AI approaches. Traditional AI chatbots typically follow structured improvement cycles, with performance data collected and analyzed before implementing system updates during scheduled releases. This approach ensures stability and predictability but may delay adaptation to emerging conversation patterns or customer needs. Modern specialized chatbots increasingly incorporate feedback mechanisms that enable gradual adjustment of response patterns based on conversation outcomes and explicit user feedback. Solutions for AI sales calls often include performance analytics that identify specific conversation points requiring optimization. ChatGPT implementations benefit from OpenAI’s continuous model improvements but face challenges in incorporating business-specific learning without comprehensive fine-tuning. Organizations increasingly implement feedback loops where successful ChatGPT interactions get captured for future prompt refinement, creating semi-automated improvement cycles. Research by MIT Sloan Management Review indicates that conversational systems implementing structured learning frameworks demonstrate 31% performance improvement after six months of deployment, compared to 14% improvement in systems without formalized learning mechanisms.
Multilingual Capabilities and Global Deployment
For organizations operating across geographic regions, language support represents a critical evaluation factor in selecting conversational AI solutions. Specialized AI chatbots historically required distinct development for each supported language, creating significant resource requirements for global implementations. Modern specialized systems increasingly incorporate multilingual capabilities, but typically require language-specific training and optimization to maintain consistent performance across languages. These implementations often demonstrate uneven capabilities, with primary languages receiving more comprehensive development than secondary markets. ChatGPT demonstrates remarkable multilingual capabilities, supporting dozens of languages with relatively consistent performance across major world languages. This linguistic flexibility makes ChatGPT particularly valuable for global organizations seeking to implement consistent conversation experiences across diverse markets without developing separate systems for each region. However, language-specific nuances and cultural references may still require prompt optimization for optimal performance in specific markets. For implementations like AI voice agent whitelabel solutions, multilingual support significantly impacts deployment scope and market reach. According to the International Association for Machine Translation, organizations implementing multi-region conversational AI report 40% higher customer engagement in non-English markets when native language support achieves near-human quality levels.
Analytics and Performance Measurement
The ability to extract actionable insights from AI conversations significantly impacts business value realization. Specialized AI chatbots typically incorporate purpose-built analytics features designed to track specific business outcomes, conversation patterns, and performance metrics aligned with their primary functions. These systems often provide detailed dashboards highlighting key performance indicators like resolution rates, conversion metrics, or efficiency measures directly relevant to their implementation context. For applications like AI appointment setters, these analytics directly connect conversation performance to business outcomes. ChatGPT implementations frequently require additional development work to create meaningful analytics frameworks, as the base model doesn’t include built-in business intelligence features. Organizations implementing ChatGPT often develop custom analytics layers that capture interaction patterns, track successful conversation paths, and identify opportunities for prompt optimization. The most sophisticated implementations incorporate A/B testing frameworks that systematically compare different conversation approaches to identify optimal patterns. Research from the Business Application Research Center indicates that organizations implementing comprehensive conversational analytics frameworks achieve 28% higher ROI from their AI investments compared to those with limited measurement capabilities.
Hybrid Approaches: Combining Strengths
Rather than viewing the choice between specialized chatbots and ChatGPT as binary, forward-thinking organizations increasingly implement hybrid architectures that leverage the strengths of both approaches. These integrated systems typically use specialized chatbots for high-volume, structured interactions where precision and efficiency are paramount, while deploying ChatGPT for handling complex, unpredictable queries that benefit from its flexibility and broad knowledge base. For example, an AI calling agency might implement specialized conversation systems for appointment scheduling and order processing while using ChatGPT for complex product inquiries or troubleshooting scenarios. Technically, these hybrid implementations often employ orchestration layers that route conversations to the appropriate system based on intent recognition, conversation complexity, or specific trigger patterns. Some advanced architectures implement handoff protocols where conversations begin with specialized systems and transition to ChatGPT when queries exceed the specialized system’s capabilities. According to Deloitte’s AI implementation research, organizations implementing coordinated hybrid conversation architectures report 34% higher customer satisfaction and 29% better resolution rates compared to those using either approach exclusively.
Future Trends: The Evolving Conversation Landscape
The conversational AI landscape continues advancing rapidly, with emerging developments reshaping the comparative advantages of different approaches. Specialized AI chatbots increasingly incorporate components from large language models while maintaining their purpose-built focus, creating systems that combine the precision of specialized solutions with improved flexibility. Meanwhile, fine-tuning capabilities for models like ChatGPT continue advancing, enabling more precise adaptation to specific business contexts without sacrificing broad capabilities. Emerging frameworks for prompt engineering are dramatically improving organizations’ ability to shape ChatGPT behavior for specific business applications. Multimodal conversation capabilities—incorporating voice, images, and eventually video alongside text—represent another frontier transforming implementation considerations. Organizations planning long-term conversational AI strategies should consider how these technological trajectories will influence the relative advantages of different approaches. The most forward-thinking implementations establish flexible architectural foundations that can incorporate new capabilities as they emerge while maintaining consistent customer experiences. According to PwC’s Future of AI survey, 73% of technology executives believe the distinction between specialized and general-purpose AI systems will blur significantly by 2025 as technologies converge and implementation frameworks mature.
Making the Right Choice for Your Business Needs
Selecting between specialized AI chatbots and ChatGPT ultimately requires careful evaluation of your specific business requirements, technical resources, and strategic objectives. Organizations should consider factors including conversation complexity, domain specificity, integration requirements, and budget constraints when making this decision. For businesses with clearly defined, structured conversation needs in specific functional areas, purpose-built chatbots often deliver superior performance with more predictable costs and straightforward implementation paths. Organizations requiring flexible conversation capabilities across diverse topics or departments frequently find ChatGPT’s versatility advantageous despite potentially higher implementation complexity. Many businesses benefit from starting with focused implementations addressing specific high-value use cases before expanding to broader deployment. Pilot programs with clear success metrics help organizations build internal expertise while delivering measurable business value. Regardless of the technical approach selected, successful implementations share common characteristics: they start with clear business objectives rather than technology preferences, incorporate ongoing optimization frameworks, and establish consistent measurement approaches that connect conversation performance to business outcomes. Tools like conversational AI platforms provide frameworks for evaluating different approaches against specific business requirements.
Transform Your Business Communications with Intelligent AI Solutions
Ready to revolutionize how your business handles customer communications? Callin.io offers a comprehensive solution for implementing AI-powered phone agents that can manage both inbound and outbound calls autonomously. Whether you’re looking to implement specialized chatbot functionality or leverage the power of advanced language models like ChatGPT, our platform provides the flexibility and tools you need. Our AI phone agents engage naturally with customers, handling everything from appointment scheduling and FAQ responses to sales conversations with human-like understanding.
Callin.io’s free account gives you access to an intuitive interface for configuring your AI agent, with test calls included and comprehensive dashboards for tracking interactions. For businesses needing advanced features like Google Calendar integration and built-in CRM functionality, our subscription plans start at just $30 per month. The battle between specialized AI chatbots and ChatGPT doesn’t have to be a choice you make alone—our AI call center solutions can help you implement the perfect approach for your specific business needs. Discover how Callin.io can transform your business communications today.
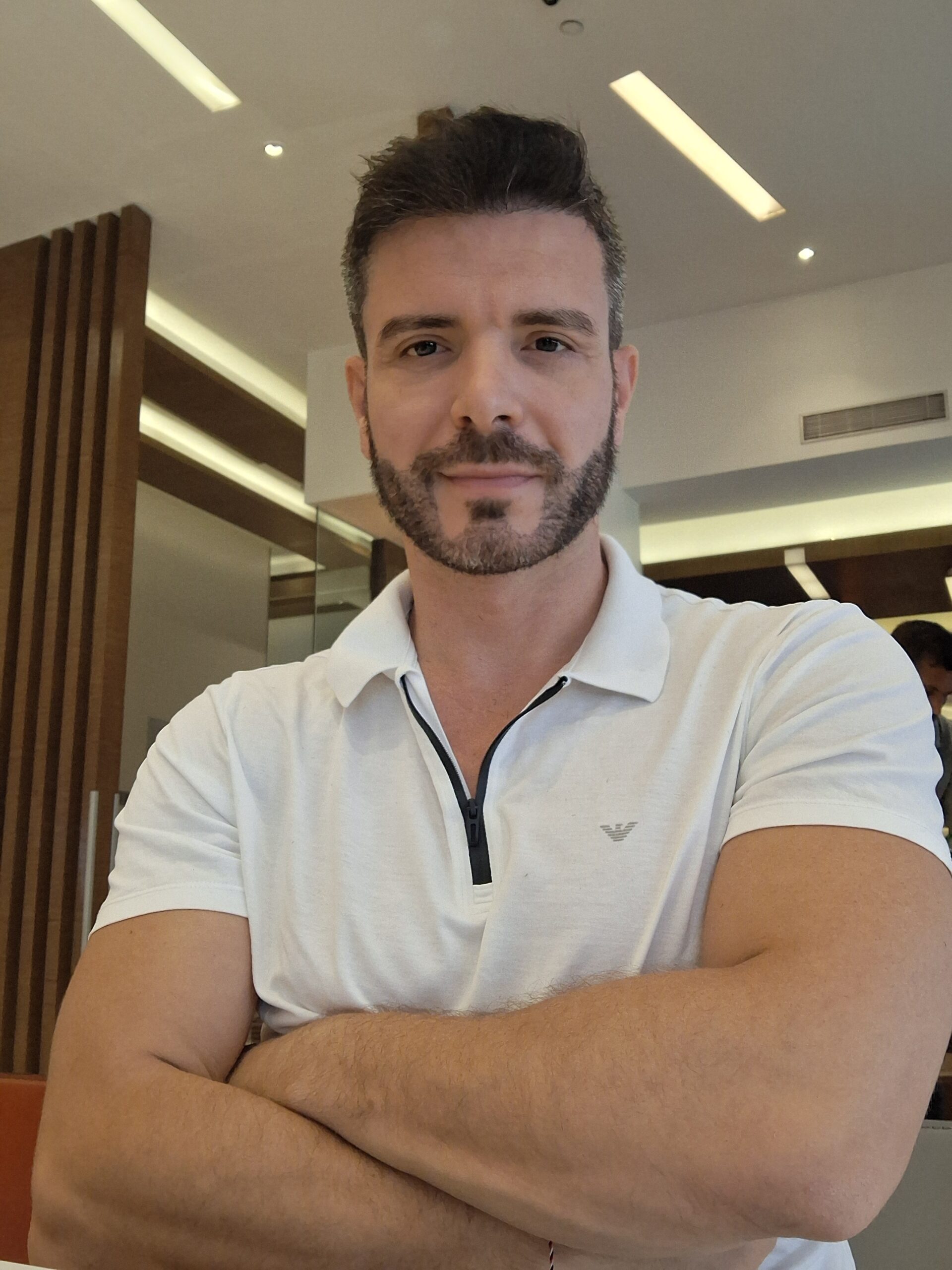
Helping businesses grow faster with AI. 🚀 At Callin.io, we make it easy for companies close more deals, engage customers more effectively, and scale their growth with smart AI voice assistants. Ready to transform your business with AI? 📅 Let’s talk!
Vincenzo Piccolo
Chief Executive Officer and Co Founder