Understanding the Analytics Revolution in B2B SaaS
The B2B SaaS sector has undergone a remarkable transformation in recent years, with advanced analytics emerging as a critical differentiator between thriving companies and those struggling to keep pace. Unlike traditional business intelligence, advanced analytics leverages sophisticated techniques like machine learning, predictive modeling, and natural language processing to uncover actionable insights from complex datasets.
For B2B SaaS leaders, implementing robust analytics capabilities isn’t merely about tracking metrics—it’s about developing a comprehensive understanding of customer behaviors, identifying emerging patterns, and making data-informed decisions that drive sustainable growth. According to research by McKinsey, companies that extensively use customer analytics outperform competitors by 126% in profit generation.
The value proposition of advanced analytics is particularly compelling for B2B SaaS organizations where recurring revenue models demand constant optimization of customer acquisition costs, lifetime value calculations, and churn prevention strategies. By harnessing the power of modern analytics tools, businesses can transform raw data into strategic assets that inform every aspect of their operations.
Innovative SaaS companies are now building dedicated analytics teams that combine statistical expertise with business acumen to extract maximum value from their data infrastructure. This cross-functional approach ensures that insights don’t remain isolated within technical silos but flow seamlessly into decision-making processes across marketing, sales, product development, and customer success departments.
Key Components of Advanced Analytics Systems
Constructing a comprehensive analytics infrastructure requires several essential components working in harmony. At its foundation lies data collection and integration—the process of gathering information from diverse sources like CRM systems, marketing automation platforms, product usage logs, and financial databases. This collection process must be both thorough and systematic to establish a single source of truth across the organization.
The next critical layer involves data processing and storage solutions, which may include data warehouses, data lakes, or hybrid architectures designed to handle structured and unstructured information. Modern cloud-based data platforms like Snowflake, Google BigQuery, and Amazon Redshift have dramatically reduced the technical barriers to implementing enterprise-grade analytics capabilities.
Advanced analytics tools then transform raw data into actionable insights through techniques like segmentation analysis, regression modeling, and cluster analysis. These tools range from specialized statistical packages to comprehensive business intelligence platforms that offer visualization capabilities and automated reporting functions.
Perhaps most importantly, effective B2B SaaS analytics systems include robust governance frameworks that ensure data quality, maintain security standards, and establish clear ownership responsibilities. Without proper governance, even the most sophisticated analytics tools will struggle to deliver reliable insights.
The final component involves insight activation—the processes and workflows that translate analytical findings into concrete business actions. This might include automated alert systems, decision support dashboards, or integration with operational systems that can execute recommended actions programmatically.
Customer Acquisition Analytics
Optimizing the customer acquisition process represents one of the most valuable applications of advanced analytics for B2B SaaS companies. By implementing sophisticated tracking and attribution models, businesses can identify which marketing channels, campaigns, and messages generate the highest quality leads at the lowest cost.
AI-powered lead scoring systems can analyze hundreds of variables—from firmographic data to engagement patterns—to predict which prospects are most likely to convert into paying customers. This enables sales teams to prioritize their efforts on high-potential opportunities, significantly increasing conversion efficiency.
Advanced analytics also helps SaaS companies understand the complete customer journey, revealing critical touchpoints that influence purchase decisions. For instance, natural language processing tools can analyze sales call transcripts to identify key phrases and objections that consistently appear in successful (or unsuccessful) conversations.
The timing of outreach activities can dramatically impact conversion rates, and predictive analytics can determine optimal engagement windows for different prospect segments. Some B2B SaaS companies have increased conversion rates by 30% simply by contacting prospects during their highest-probability purchase windows, as identified through behavioral pattern recognition.
Perhaps most importantly, acquisition analytics enables continuous experimentation and optimization through rigorous A/B testing frameworks. By systematically varying elements of marketing and sales approaches while carefully measuring outcomes, companies can gradually refine their acquisition strategies based on empirical evidence rather than intuition or industry conventions.
Customer Retention and Expansion Analytics
For subscription-based businesses, maintaining and growing existing customer relationships often represents a more cost-effective growth strategy than pursuing new acquisitions. Advanced analytics tools excel at identifying early warning signals of potential churn by monitoring product usage patterns, support interactions, and engagement metrics.
Sophisticated churn prediction models can integrate data from multiple sources to calculate individual risk scores for each customer, enabling proactive intervention before cancellation occurs. These models become increasingly accurate over time through machine learning algorithms that continuously refine their predictive capabilities based on observed outcomes.
Beyond retention, expansion analytics helps identify cross-sell and upsell opportunities within the existing customer base. By analyzing usage patterns, feature adoption, and growth indicators, companies can determine which customers are likely ready for additional services or higher-tier plans. For example, AI-powered virtual assistants can automatically detect when users are consistently reaching usage limits or accessing premium features during trial periods.
Customer health scoring represents another powerful application, combining multiple signals into comprehensive metrics that summarize relationship strength. These scores often incorporate factors like product adoption depth, support ticket frequency, executive engagement levels, and contractual details to provide a holistic view of account health.
Research by Bain & Company indicates that a 5% increase in customer retention can boost profits by 25% to 95%, highlighting the enormous financial impact of effective retention analytics programs. SaaS companies that excel in this area typically establish dedicated customer success operations teams that use analytics insights to guide their relationship management strategies.
Product Usage and Feature Adoption Analytics
Understanding how customers interact with your software represents a fundamental advantage of SaaS business models. Through event tracking and behavioral analytics, product teams can create detailed maps of user journeys, identifying both common paths and exceptional patterns.
Advanced feature usage analytics helps product managers determine which capabilities drive the most value and which might benefit from usability improvements or additional education efforts. By correlating feature adoption with retention and expansion metrics, companies can prioritize development resources on high-impact areas.
Cohort analysis—examining how different user groups engage with the product over time—provides crucial insights into the effectiveness of onboarding processes and feature rollouts. These analyses often reveal that users who adopt specific key features within their first few days show significantly higher long-term retention rates.
Many leading SaaS companies now employ product analytics platforms like Amplitude, Mixpanel, or Pendo to track detailed user interactions. These platforms can reveal potential friction points in the user experience through session recordings and heatmaps that show exactly how users navigate through the application.
When integrated with customer feedback data from surveys and support interactions, product usage analytics creates a comprehensive understanding of customer needs and pain points. This 360-degree view enables product teams to make informed decisions about roadmap priorities and UX improvements that will have the greatest business impact.
Revenue and Financial Analytics
For B2B SaaS companies, financial metrics require specialized analytical approaches due to the recurring revenue model’s complexity. Advanced analytics systems can track crucial metrics like Monthly Recurring Revenue (MRR), Annual Recurring Revenue (ARR), Customer Acquisition Cost (CAC), and Customer Lifetime Value (CLV) with granular precision.
Revenue forecasting models become significantly more accurate when they incorporate usage patterns, customer health scores, and market indicators alongside historical financial data. Machine learning algorithms can detect subtle patterns that traditional forecasting methods might miss, helping finance teams prepare for seasonal fluctuations or changing market conditions.
Unit economics analysis becomes particularly powerful when combined with customer segmentation, revealing which customer profiles generate the highest profit margins and which might actually be unprofitable despite generating revenue. This insight often leads to strategic shifts in targeting and pricing strategies.
Pricing optimization represents another high-impact application area, with advanced analytics helping determine optimal price points for different market segments and feature configurations. Some SaaS companies have implemented dynamic pricing models that respond to usage patterns, company size, and perceived value metrics collected through ongoing customer interactions.
By establishing automated financial dashboards that update in real-time, executives gain continuous visibility into business performance rather than waiting for monthly or quarterly reports. This accelerated feedback loop enables faster course corrections when key metrics begin trending in unexpected directions.
Operational Efficiency Analytics
Beyond customer-facing metrics, advanced analytics can dramatically improve internal operations within B2B SaaS organizations. Resource allocation optimization ensures that development, support, and sales efforts focus on activities with the highest return on investment.
AI-powered support systems can analyze thousands of historical support tickets to identify common issues, determine optimal staffing levels across different time periods, and even suggest process improvements that might prevent problems before they occur. Many companies have reduced support costs by 20-30% through analytics-driven workflow optimizations.
Development operations (DevOps) analytics tracks deployment frequency, change failure rates, and recovery times to identify opportunities for improving the software delivery process. These metrics help engineering teams balance velocity and stability while maintaining high quality standards.
Sales operations benefits enormously from analytics that reveal territory effectiveness, sales cycle optimization opportunities, and performance patterns across representatives. By identifying the activities and approaches that consistently lead to successful outcomes, sales leaders can replicate winning strategies across their entire organization.
Customer onboarding represents another critical operational area where analytics drives significant improvements. By measuring time-to-value and detecting common obstacles during implementation, companies can systematically refine their onboarding processes to accelerate customer success.
Competitive Intelligence and Market Analytics
Understanding competitive positioning and market dynamics represents a critical application of advanced analytics for B2B SaaS companies. By monitoring competitor product changes, pricing strategies, and marketing messages, businesses gain valuable context for their own strategic decisions.
Web scraping tools combined with natural language processing can systematically track competitor websites, app stores, and social media channels to detect new features, positioning changes, or emerging messaging trends. This automated competitive intelligence provides early warning of potential threats or market opportunities.
Market trend analysis using large-scale data processing can identify emerging customer needs or technology shifts before they become obvious to the broader market. Companies that detect these early signals gain valuable lead time to adapt their product roadmaps accordingly.
Sentiment analysis of social media conversations, review sites, and forum discussions provides insights into how both your product and competitive offerings are perceived by actual users. These unfiltered perspectives often reveal important quality perceptions that might not surface through formal feedback channels.
Geographic expansion opportunities can be identified through analyses that combine market size data, competitive intensity measures, and cultural compatibility indicators. This data-driven approach to market prioritization helps companies focus their limited resources on regions that offer the highest probability of success.
Implementing an Effective Analytics Strategy
Successfully deploying advanced analytics requires careful planning and organizational alignment. The journey typically begins with establishing clear business objectives that the analytics program should address, ensuring alignment between technical capabilities and strategic priorities.
Data infrastructure readiness forms the foundation of any analytics initiative, requiring careful assessment of current systems and potential integration challenges. Many companies find that implementing a dedicated data platform separate from operational systems provides greater flexibility for analytical processing.
Team composition represents another critical consideration, as effective analytics programs require a blend of technical skills, domain expertise, and communication capabilities. Instead of isolating data scientists, leading organizations create cross-functional teams that include business stakeholders, engineers, and analytics specialists working collaboratively.
Analytics governance frameworks establish clear roles, responsibilities, and processes for data management, model validation, and insight deployment. Without proper governance, even sophisticated analytics initiatives can struggle to maintain data quality and organizational trust.
Phased implementation approaches typically yield better results than attempting comprehensive transformations. By starting with high-impact, manageable projects that demonstrate clear value, organizations build momentum and expertise gradually while establishing credibility for the analytics function.
Real-World Success Stories
Zendesk transformed its customer retention strategy by implementing an advanced analytics system that predicted churn risk with 83% accuracy three months in advance. By identifying at-risk customers early, their customer success teams could intervene proactively, resulting in a 14% reduction in overall churn rate within the first year of implementation.
DocuSign leveraged AI-based analytics to optimize their sales process by analyzing thousands of successful deals. The system identified specific engagement patterns—including optimal contact cadence, message content, and stakeholder involvement—that significantly increased conversion probability. After implementing these insights, they achieved a 28% increase in sales productivity.
Atlassian implemented product usage analytics that revealed surprising patterns in feature adoption across different customer segments. This analysis led to a complete redesign of their onboarding workflows tailored to specific user types, resulting in a 35% increase in new user activation rates and substantially improved long-term retention.
Salesforce used advanced revenue analytics to optimize their complex pricing structure across different market segments. By analyzing the relationship between feature utilization, perceived value, and willingness to pay, they redesigned their packaging strategy to better align with customer needs while simultaneously increasing average contract values by 18%.
These success stories highlight a common pattern—companies that achieve exceptional results from analytics initiatives typically maintain a relentless focus on translating insights into concrete actions rather than simply generating interesting reports or dashboards.
Common Challenges and Solutions
Despite its transformative potential, implementing advanced analytics presents several significant challenges for B2B SaaS companies. Data quality issues frequently undermine analytics initiatives, as inconsistent or incomplete information leads to unreliable insights. Successful organizations address this challenge by establishing data governance committees and implementing systematic data validation processes.
Integration complexity across disparate systems often creates technical obstacles, particularly in companies that have grown through acquisitions or built their technology stack incrementally. Leading companies overcome this challenge by implementing modern data integration platforms with pre-built connectors and establishing clear data exchange standards.
Skills gaps represent another common barrier, as the analytics talent market remains highly competitive. Forward-thinking organizations address this challenge through multi-faceted approaches including internal training programs, strategic hiring, and partnerships with specialized analytics service providers.
Organizational resistance often emerges when analytics insights challenge established practices or intuitions. Overcoming this resistance requires deliberate change management approaches including executive sponsorship, early stakeholder involvement, and careful communication of the reasoning behind analytics-driven recommendations.
AI model transparency has emerged as a growing concern, particularly for customer-facing analytics applications where algorithmic decisions might affect service levels or pricing. Leading companies address these concerns by implementing explainable AI approaches that provide clear rationales for model recommendations.
Future Trends in B2B SaaS Analytics
As we look toward the horizon, several emerging trends promise to reshape advanced analytics for B2B SaaS companies. Artificial intelligence augmentation will increasingly automate routine analysis tasks while enhancing human analytical capabilities, enabling teams to focus on strategic interpretation rather than data manipulation.
Real-time analytics processing will continue accelerating, with insights generated and deployed within seconds rather than hours or days. This capability will enable truly dynamic responses to customer behaviors and market shifts, creating competitive advantages for companies with advanced technical infrastructures.
Natural language interfaces will democratize access to analytics insights, allowing non-technical users to query complex datasets through conversational interactions. These interfaces will dramatically expand the number of employees who can directly leverage data for decision-making across organizations.
Embedded analytics will increasingly blur the line between operational systems and analytical capabilities, with insights and recommendations appearing directly within workflow tools rather than in separate dashboards or reports. This integration will accelerate the translation of insights into actions.
Privacy-preserving analytics techniques will gain importance as regulatory requirements and customer expectations around data protection continue evolving. Technologies like federated learning, differential privacy, and homomorphic encryption will enable powerful analytics capabilities while maintaining strong privacy safeguards.
Tools and Technologies for Advanced Analytics
The B2B SaaS analytics ecosystem encompasses a diverse array of specialized tools addressing different requirements. Data integration platforms like Fivetran, Stitch, and Airbyte streamline the process of collecting information from multiple sources while maintaining consistency and reliability.
Data storage and processing solutions include cloud data warehouses like Snowflake and BigQuery, which offer virtually unlimited scalability for analytical workloads without requiring complex infrastructure management.
Business intelligence platforms such as Tableau, Looker, and Power BI provide visualization capabilities that transform complex datasets into intuitive dashboards accessible to non-technical users throughout the organization.
Specialized SaaS analytics tools like ChartMogul, ProfitWell, and Baremetrics offer pre-built metrics specifically designed for subscription businesses, eliminating the need to build custom calculations for common SaaS measurements.
Machine learning platforms including DataRobot, H2O.ai, and cloud-native services from major providers enable predictive modeling capabilities without requiring deep data science expertise. These platforms increasingly offer automated machine learning (AutoML) features that handle much of the model development process.
Customer data platforms (CDPs) like Segment, mParticle, and Rudderstack help create unified customer profiles combining information from multiple touchpoints, enabling truly comprehensive analytics across the entire customer journey.
Building an Analytics-Driven Culture
Technology represents only one aspect of successful analytics implementation—organizational culture ultimately determines whether insights translate into business impact. Creating an analytics-driven culture starts with leadership commitment that consistently reinforces the importance of data-informed decision making.
Data literacy programs help employees across functions develop the skills needed to interpret and apply analytical insights effectively. These programs range from basic dashboard training to more advanced statistical concepts depending on role requirements.
Insight sharing processes ensure that valuable analytics discoveries propagate throughout the organization rather than remaining isolated within specific teams. Regular insight review sessions, centralized knowledge repositories, and analytics newsletters can all contribute to broader awareness.
Experimentation frameworks establish systematic approaches for testing hypotheses and measuring outcomes, creating a scientific approach to business innovation. These frameworks typically include standardized methodologies for designing experiments, determining sample sizes, and evaluating statistical significance.
Perhaps most importantly, analytics-driven cultures celebrate and reward decisions based on data rather than intuition or seniority. By publicly acknowledging successful data-driven initiatives and creating career advancement paths for analytically-skilled employees, organizations reinforce the behaviors that drive analytical excellence.
Strategic Recommendations for Implementation
For B2B SaaS companies beginning their advanced analytics journey, several strategic recommendations can increase the probability of success. Start by conducting a comprehensive data audit to understand what information assets already exist across the organization and where critical gaps might limit analytical capabilities.
Prioritize high-impact use cases with clear business value rather than pursuing technical sophistication for its own sake. Projects that address pressing business challenges while demonstrating tangible returns help build organizational momentum and secure continued investment.
Build for scalability from the beginning by establishing data architectures and governance processes that can accommodate growing data volumes and evolving analytical needs. Short-term workarounds often create technical debt that becomes increasingly difficult to address as analytics programs mature.
Invest in change management alongside technical implementation to ensure that insights actually influence decision-making processes. This includes stakeholder engagement, communication planning, and workflow integration to seamlessly incorporate analytics into daily operations.
Start small but think big by implementing initial projects that demonstrate quick wins while maintaining a long-term strategic vision for analytics capabilities. This balanced approach allows organizations to gain experience and build confidence while working toward more sophisticated applications.
Leveraging Advanced Analytics for Transformative Results
The strategic application of advanced analytics represents a defining competitive advantage for forward-thinking B2B SaaS companies. By systematically collecting, analyzing, and acting upon customer data, these organizations build deeper relationships, deliver more personalized experiences, and identify growth opportunities with unprecedented precision.
The most successful implementations share common characteristics: they align analytics initiatives with core business objectives, prioritize actionability over complexity, and create organizational structures that enable rapid translation of insights into operational decisions. They also recognize that analytics maturity develops gradually, with capabilities evolving through continuous learning and refinement.
For executives considering investments in advanced analytics, the business case extends far beyond operational efficiencies. The true strategic value emerges when analytics capabilities enable entirely new business models, previously impossible product offerings, or revolutionary customer experiences that differentiate the company in meaningful ways.
As the B2B SaaS marketplace grows increasingly competitive, companies that develop sophisticated analytical capabilities gain sustainable advantages in customer acquisition, retention, and expansion. These advantages compound over time as analytical insights drive continuous optimization across all aspects of the business.
Harness the Power of AI-Driven Communication with Callin.io
If you’re looking to transform your business communications using advanced analytics principles discussed throughout this article, Callin.io offers an ideal solution. Their AI-powered phone agents provide a practical application of analytics-driven automation, handling incoming and outgoing calls autonomously while gathering valuable customer interaction data.
Callin.io’s platform lets you implement intelligent virtual receptionists that schedule appointments, answer common questions, and even close sales by engaging with customers in natural conversation. These interactions generate rich datasets that can inform your broader analytics strategies and customer experience improvements.
Getting started is straightforward with Callin.io’s free account option, which includes a user-friendly interface for configuring your AI agent, complimentary test calls, and access to the comprehensive task dashboard for monitoring performance. For businesses requiring more advanced capabilities such as Google Calendar integration or built-in CRM functionality, premium plans start at just $30 per month.
Discover how Callin.io can help you implement practical analytics-driven communication strategies by visiting their website today.
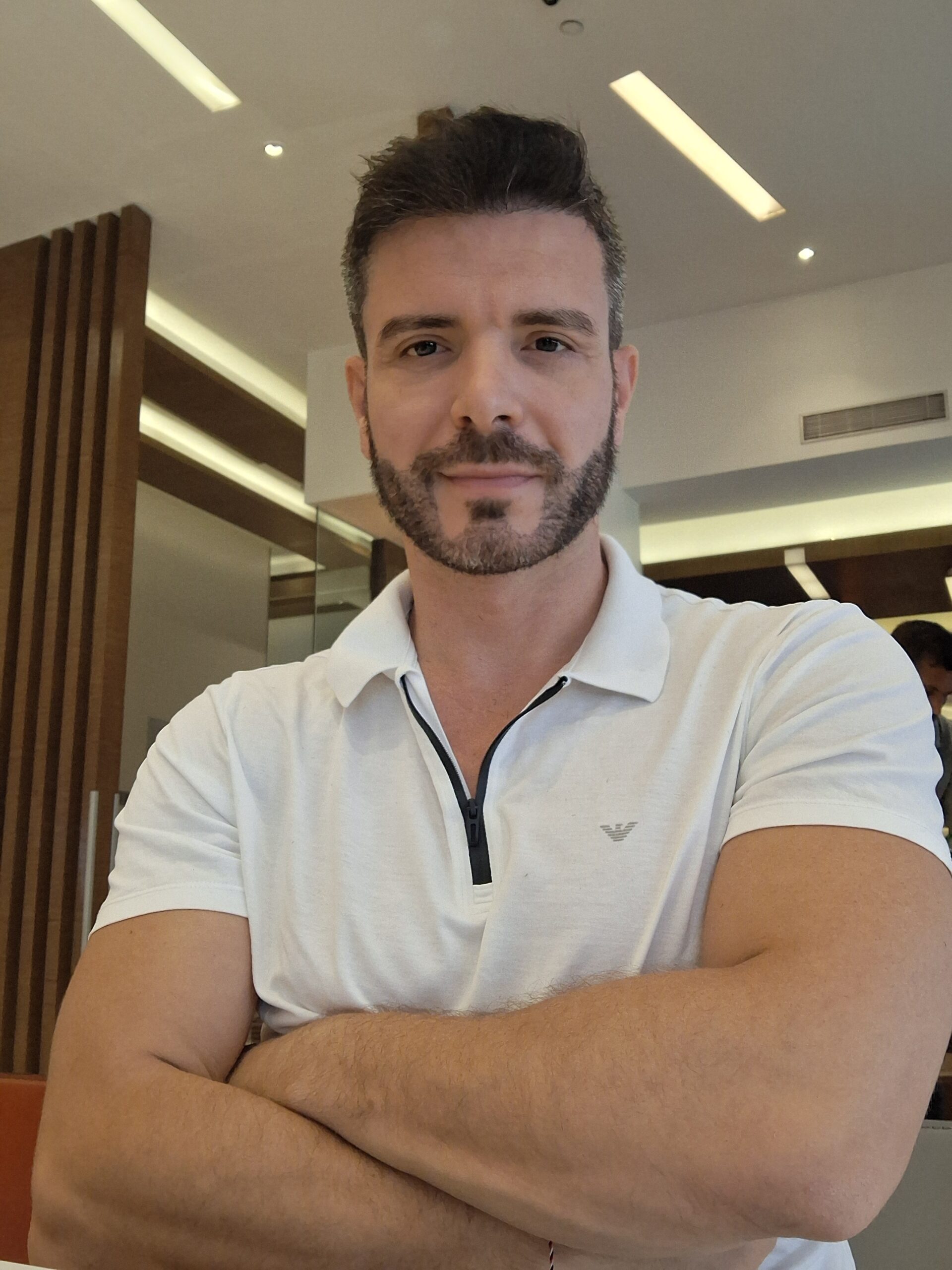
Helping businesses grow faster with AI. 🚀 At Callin.io, we make it easy for companies close more deals, engage customers more effectively, and scale their growth with smart AI voice assistants. Ready to transform your business with AI? 📅 Let’s talk!
Vincenzo Piccolo
Chief Executive Officer and Co Founder