The Strategic Value of Predictive Maintenance
Predictive maintenance has emerged as a game-changing approach for manufacturing and industrial operations worldwide. Unlike traditional maintenance methods that rely on fixed schedules or react to breakdowns, predictive maintenance harnesses data analytics to forecast equipment failures before they occur. This forward-thinking strategy allows companies to service machinery precisely when needed, drastically reducing costly downtime while extending asset lifespan. According to a McKinsey report, organizations implementing effective predictive maintenance programs can reduce maintenance costs by 15-30% and eliminate breakdowns by 70-75%. The integration of predictive techniques with conversational AI systems creates powerful tools that can notify maintenance teams and coordinate responses, transforming how industrial operations manage their critical assets.
Historical Evolution: From Reactive to Predictive Models
The journey toward predictive maintenance reflects the broader industrial transformation over decades. Manufacturing facilities once relied exclusively on reactive maintenance—fixing equipment only after failure—resulting in production halts and emergency repair expenses. This evolved into preventive maintenance with regular service intervals based on time or usage metrics. While an improvement, these schedules often led to unnecessary maintenance or missed emerging issues. The digital revolution introduced condition-based monitoring, using sensors to track equipment health in real-time. Today’s predictive maintenance builds upon this foundation, adding sophisticated analytics that detect subtle performance patterns invisible to human observers. This progression demonstrates the industry’s shift from crisis management to strategic foresight, as outlined in IEEE’s comprehensive study on maintenance paradigm evolution.
The Technology Stack Powering Modern Systems
Implementing effective predictive maintenance requires a sophisticated technology ecosystem. At the foundation lie industrial IoT sensors that continuously gather operational data—vibration patterns, temperature fluctuations, power consumption, and acoustic signatures. This data feeds into specialized analytics platforms that process information in real-time, comparing current performance against historical baselines. Machine learning algorithms, particularly those focused on anomaly detection, identify subtle deviations in equipment behavior that often precede failures. Cloud infrastructure provides the necessary computing power and storage capabilities, while edge computing enables critical processing to occur directly on the factory floor for time-sensitive applications. The Industrial Internet Consortium notes that successfully deployed systems typically integrate multiple technologies rather than relying on standalone solutions, creating comprehensive monitoring networks that provide unprecedented visibility into operations.
Key Performance Indicators and Business Impact
The financial impact of predictive maintenance extends far beyond repair cost reduction. When properly implemented, these systems deliver measurable improvements across numerous business metrics. Overall Equipment Effectiveness (OEE) typically increases by 10-20% as unplanned downtime decreases and production efficiency improves. Mean Time Between Failures (MTBF) often extends 20-40%, while maintenance labor costs decrease 10-30% through more efficient resource allocation. Energy consumption often drops 5-15% as equipment operates at optimal efficiency. Perhaps most significantly, predictive technologies can extend asset lifecycle by 20-40%, dramatically improving return on capital investments. These figures, compiled from various industry studies by Aberdeen Group, demonstrate why predictive maintenance has become a cornerstone of digital transformation initiatives across manufacturing, energy, transportation, and utilities sectors.
Machine Learning Models Transforming Maintenance
The analytical engines driving predictive capabilities rely on various machine learning approaches tailored to specific maintenance challenges. Regression models excel at forecasting time-to-failure based on historical performance data, while classification algorithms identify equipment states (normal, degrading, or pre-failure). More sophisticated deep learning networks can detect complex patterns across multiple sensor inputs, particularly useful for equipment with numerous interdependent components. Natural Language Processing (NLP) systems analyze maintenance logs and technician notes to extract insights from unstructured data. Each approach offers distinct advantages, with most advanced implementations utilizing model ensembles that combine strengths of multiple techniques. A notable case study from MIT Technology Review documents how aerospace manufacturers use neural networks to analyze vibration data from jet engines, achieving 97% accuracy in predicting component failures up to 30 days in advance.
Real-World Implementation: Sensors and Data Collection
The foundation of any predictive maintenance system begins with comprehensive data collection through strategically deployed sensors. Vibration analyzers detect subtle changes in rotational equipment, while thermal imaging cameras identify overheating components before visible damage occurs. Ultrasonic sensors can detect pressure leaks or bearing issues through sound pattern analysis, and power monitoring tools track electrical consumption anomalies that often precede motor failures. The challenge lies not just in sensor selection but in thoughtful deployment—balancing coverage against cost while considering environmental factors like heat, moisture, and dust that affect sensor reliability. Manufacturers must create robust data pipelines that process thousands of data points per second without overwhelming storage systems. As noted in Control Engineering, leading implementations focus on installing sensors at critical failure points identified through Failure Mode and Effects Analysis (FMEA), maximizing ROI while minimizing deployment complexity.
Industry 4.0 and the Connected Factory Ecosystem
Predictive maintenance represents one of the most mature applications within the broader Industry 4.0 framework. In today’s connected factory environment, maintenance systems don’t operate in isolation but integrate with numerous other operational technologies. Manufacturing Execution Systems (MES) coordinate maintenance activities with production scheduling to minimize disruption. Enterprise Resource Planning (ERP) platforms automatically generate parts orders based on predicted failures. Digital twins create virtual models of physical equipment, allowing engineers to simulate maintenance interventions before physical execution. These integrations create a networked ecosystem where information flows seamlessly between systems, enabling coordinated responses to developing issues. The World Economic Forum has identified these interconnected systems as essential elements of next-generation manufacturing, forecasting productivity improvements of 30-50% for facilities that successfully implement comprehensive digital strategies incorporating predictive capabilities.
Case Study: Automotive Manufacturing Transformation
The automotive industry provides compelling examples of predictive maintenance’s transformative impact. A leading European automobile manufacturer implemented a factory-wide solution focused on critical robotic welding equipment across twelve production lines. By analyzing motor current signatures, vibration patterns, and temperature data, the system identified developing issues in servo motors and control systems before failures occurred. Within eighteen months, unplanned downtime decreased by 37%, maintenance costs dropped 29%, and production capacity increased without additional capital investment. Particularly impressive was the system’s ability to differentiate between normal wear patterns and problematic degradation, reducing false alarms that had plagued earlier monitoring attempts. The manufacturer is now expanding the program to include predictive quality analysis, using the same sensor infrastructure to identify potential defects in finished products. This integration of maintenance and quality systems, detailed in Automotive Manufacturing Solutions, represents the next evolution in predictive technologies.
Challenges in Implementation and Adoption
Despite its compelling benefits, predictive maintenance faces significant implementation barriers in many organizations. Data quality issues frequently undermine analytical accuracy, with incomplete histories and sensor inconsistencies creating blind spots. Legacy equipment often lacks built-in monitoring capabilities, requiring retrofit solutions that increase deployment costs. Many facilities struggle with technical expertise gaps as traditional maintenance teams adapt to data-driven methodologies. Organizational resistance can emerge when established maintenance processes are disrupted by new approaches. Integration challenges with existing systems further complicate implementation. Companies successfully overcoming these obstacles typically start with limited pilot programs focused on critical assets before expanding, using initial successes to build organizational support. They also invest in training programs that bridge the skill gap between traditional maintenance expertise and digital competencies, as highlighted in a PwC industrial insights report.
The Human Element: Workforce Transformation
The shift toward predictive maintenance fundamentally transforms maintenance roles and team structures. Traditional reactive maintenance teams focused primarily on rapid response and repair skills. Today’s predictive maintenance specialists combine mechanical knowledge with data interpretation abilities, understanding both equipment operation and performance analytics. Reliability engineers now work alongside data scientists to refine prediction models and establish maintenance protocols. Technicians increasingly use augmented reality tools that overlay diagnostic information and repair guidance onto physical equipment. This evolution requires thoughtful workforce development strategies, including targeted training programs and often job restructuring. Organizations like ASME report that companies successfully navigating this transition typically involve maintenance teams in technology selection and implementation, recognizing that worker engagement directly correlates with successful adoption.
Cost-Benefit Analysis and ROI Considerations
Financial justification remains critical for organizations considering predictive maintenance investments. Implementation costs vary widely based on scale and complexity, typically ranging from $50,000 for limited deployments to several million for enterprise-wide systems. Key expenditure categories include sensor hardware (15-25% of budget), analytics platforms (20-30%), integration services (25-35%), and staff training (10-15%). Return on investment analysis must consider both direct savings (maintenance cost reduction, decreased spare parts inventory) and indirect benefits (increased production time, extended equipment life, reduced energy consumption). Most implementations achieve positive ROI within 12-24 months, with the most successful programs reporting 10:1 return ratios over five-year periods. According to Deloitte’s manufacturing analytics survey, companies should prioritize applications with clear financial impacts and reasonable implementation complexity when starting their predictive maintenance journey.
Cloud vs. Edge Computing for Maintenance Systems
The architectural decisions underlying predictive maintenance systems significantly impact their effectiveness and scalability. Cloud-based platforms offer virtually unlimited storage and processing capabilities, facilitating complex analytics across multiple facilities and enabling organizations to identify patterns across their entire equipment fleet. Conversely, edge computing brings processing power directly to the factory floor, reducing latency for time-critical applications and enabling operation in environments with limited connectivity. Many successful implementations utilize hybrid approaches—processing routine monitoring at the edge while sending aggregated data to cloud platforms for deeper analysis and cross-facility insights. This architectural flexibility allows organizations to balance immediate operational needs with strategic analytical capabilities, adapting as technologies and requirements evolve. As explored in IEEE Spectrum, advancing edge computing capabilities are increasingly enabling sophisticated analytics directly at the machine level, further expanding implementation options.
Predictive Maintenance for Critical Infrastructure
Beyond manufacturing, predictive maintenance plays an increasingly vital role in critical infrastructure protection. Power generation facilities use transformer oil analysis and generator vibration monitoring to prevent electrical grid failures. Water utilities deploy acoustic sensors to identify developing leaks before they cause service disruptions. Railway operators analyze track geometry measurements to schedule maintenance before safety risks develop. Bridge monitoring systems combine strain gauges, accelerometers, and visual inspections to track structural health. These applications share common challenges—aging infrastructure, public safety implications, and the need to maintain service continuity while performing maintenance. The American Society of Civil Engineers estimates that predictive approaches could reduce the national infrastructure maintenance backlog by 15-25% through more efficient resource allocation, demonstrating the technology’s potential beyond traditional industrial applications.
Machine Health Monitoring with Vibration Analysis
Vibration analysis represents one of the most established and reliable predictive maintenance techniques, particularly for rotating equipment. Every machine creates a unique vibration signature during normal operation—effectively a mechanical fingerprint that changes subtly as components wear or faults develop. Frequency spectrum analysis can distinguish between various failure modes: bearing defects, misalignment, imbalance, or looseness. Modern systems capture data through permanently mounted sensors or periodic measurements using portable devices, feeding information into specialized software that identifies developing issues weeks or months before audible or visible symptoms appear. This early detection window allows maintenance planning to occur during scheduled downtime rather than emergency situations. According to Plant Engineering magazine, organizations implementing comprehensive vibration monitoring typically reduce bearing-related failures by 65-75%, with each prevented emergency bearing replacement saving 8-12 hours of unplanned downtime.
Integration with Enterprise Asset Management (EAM)
The full potential of predictive maintenance emerges when seamlessly integrated with broader asset management systems. Enterprise Asset Management (EAM) platforms coordinate the entire asset lifecycle—from procurement through operation to eventual retirement—while predictive maintenance delivers the intelligence needed for optimal maintenance timing. This integration creates numerous operational advantages: work order automation that triggers maintenance requests based on predicted failures, inventory optimization that ensures parts availability without excessive stockpiling, and maintenance schedule optimization that balances workloads across technical teams. Leading organizations further extend these integrations to financial systems, automatically calculating maintenance impact on asset depreciation and capital planning. The Asset Leadership Network notes that organizations with mature integrations between predictive systems and asset management platforms typically reduce total asset management costs 15-20% while extending asset useful life by similar percentages.
Security Considerations for Connected Systems
As predictive maintenance systems become increasingly networked, cybersecurity considerations grow correspondingly important. Industrial networks once operated in isolation (air-gapped from external systems), but today’s connected architecture introduces new vulnerability vectors. Industrial control system security requires specialized approaches that balance operational technology (OT) requirements with information technology (IT) protection standards. Organizations must implement defense-in-depth strategies including network segmentation, access controls, firmware update management, and anomaly detection specifically tuned for maintenance systems. Security planning should address both data confidentiality (protecting proprietary manufacturing information) and system integrity (preventing manipulation of sensor data or maintenance schedules). The Industrial Control Systems Cyber Emergency Response Team (ICS-CERT) provides guidelines specifically addressing predictive maintenance security, emphasizing the importance of security-by-design principles rather than retrofitted protections.
Future Directions: AI and Autonomous Maintenance
The evolution of predictive maintenance continues toward increasingly autonomous systems that not only detect developing issues but independently initiate corrective actions. Advanced facilities are implementing self-healing systems that automatically adjust operational parameters to compensate for developing problems, extending equipment life while maintenance is scheduled. Artificial intelligence capabilities continue advancing beyond pattern detection into prescriptive recommendations, suggesting specific repair approaches optimized for each failure mode. Research at institutions like Carnegie Mellon’s Manufacturing Futures Initiative focuses on developing autonomous maintenance robots that can perform routine adjustments and repairs guided by predictive analytics. While complete maintenance automation remains years away for complex equipment, hybrid systems that combine human expertise with AI assistance represent the current frontier, allowing technicians to focus on complex issues while routine tasks become increasingly automated.
Environmental and Sustainability Impacts
Predictive maintenance delivers significant environmental benefits that often receive less attention than operational improvements. By keeping equipment operating at peak efficiency, these systems reduce energy consumption 5-15% compared to poorly maintained alternatives. More efficient operation directly translates to reduced carbon emissions across industrial facilities. Resource conservation improves through extended component lifespan and reduced material waste. Oil analysis enables condition-based lubrication changes rather than calendar-based replacement, reducing hazardous waste generation. Additionally, preventing catastrophic failures eliminates environmental incidents like coolant or hydraulic fluid releases. The United Nations Industrial Development Organization identifies predictive maintenance as an important tool for industrial sustainability, noting that optimized maintenance directly contributes to at least four Sustainable Development Goals through resource efficiency improvements and environmental impact reduction.
Mobile and Wearable Technologies for Maintenance Teams
Maintenance execution is transforming through mobile and wearable technologies that bridge the gap between analytical systems and field technicians. Augmented reality headsets overlay diagnostic information directly onto equipment being serviced, allowing technicians to visualize infrared heat signatures, ultrasonic readings, or historical performance data while conducting inspections. Mobile applications deliver real-time alerts, work instructions, and equipment history to maintenance staff anywhere in the facility. Wearable sensors monitor technician safety in hazardous environments while tracking maintenance progress. Voice-activated AI assistants allow hands-free documentation and information retrieval during complex repairs. These technologies significantly improve first-time-fix rates by ensuring technicians arrive properly prepared with correct parts and procedures. According to Field Service News, organizations deploying comprehensive mobile solutions typically reduce repair time 20-30% while improving repair quality through better procedural compliance.
Developing a Strategic Implementation Roadmap
For organizations beginning their predictive maintenance journey, developing a structured implementation roadmap proves essential for success. The process should begin with asset criticality assessment—identifying equipment where failures create the greatest operational impact. This analysis guides prioritization and resource allocation. Pilot programs should target high-value applications with clear success metrics, generating quick wins that build organizational confidence. Data infrastructure requirements must be assessed early, ensuring sufficient collection, storage, and processing capabilities. Change management strategies need equal attention, addressing skill development needs and workflow adjustments. Cross-functional teams combining maintenance expertise, IT capabilities, and production knowledge achieve the best results. A phased expansion approach allows organizations to refine methodologies based on initial learnings before broadening scope. The International Society of Automation recommends three-year implementation horizons for enterprise-wide deployments, allowing sufficient time for technology integration and organizational adaptation.
Transforming Your Operations with Callin.io’s Smart Solutions
As predictive maintenance continues revolutionizing industrial operations, communication systems play a crucial role in ensuring rapid response to developing issues. When your maintenance systems detect potential equipment problems, efficient communication becomes the difference between proactive resolution and costly downtime. Callin.io offers AI-powered phone agents that seamlessly integrate with your maintenance workflows, automatically notifying technicians about developing issues, scheduling urgent inspections, and coordinating parts delivery. These intelligent communication systems can prioritize alerts based on severity, ensure the right maintenance specialists are contacted, and provide detailed equipment information during calls.
Unlike traditional notification systems, Callin.io’s AI phone agents can engage in natural conversations, gathering additional information while providing maintenance personnel with real-time updates. The platform’s integration capabilities allow it to connect directly with your predictive maintenance systems, creating a closed-loop communication process that accelerates response times. With a free account option to explore the platform’s capabilities and affordable subscription plans starting at just 30USD monthly, Callin.io provides an accessible way to enhance your predictive maintenance program’s communication effectiveness. Discover how Callin.io’s AI voice agents can become an essential component of your maintenance transformation strategy.
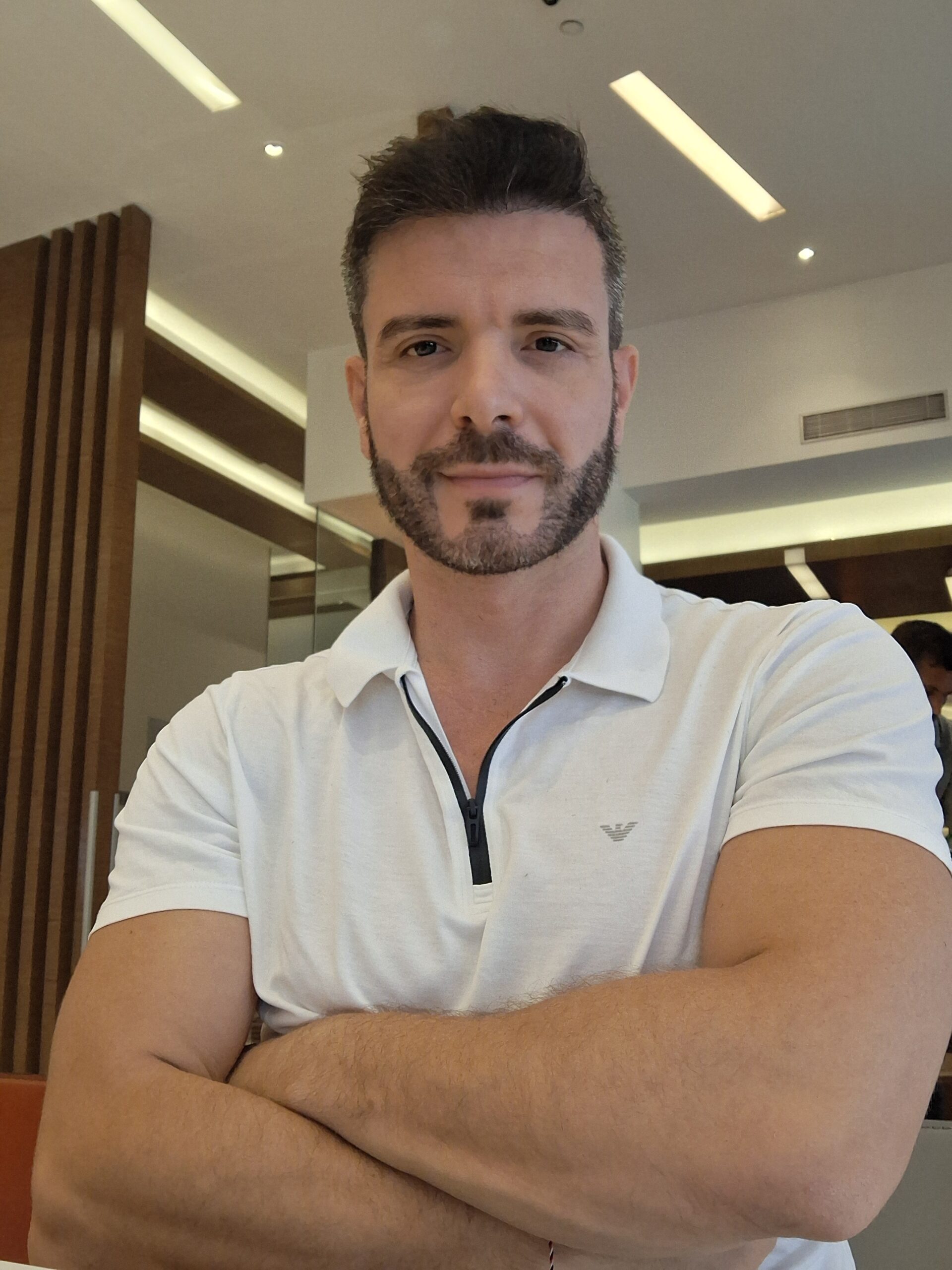
Helping businesses grow faster with AI. 🚀 At Callin.io, we make it easy for companies close more deals, engage customers more effectively, and scale their growth with smart AI voice assistants. Ready to transform your business with AI? 📅 Let’s talk!
Vincenzo Piccolo
Chief Executive Officer and Co Founder