Understanding the Power of SaaS-Based Business Intelligence
Business Intelligence (BI) has transformed significantly over the past decade, with SaaS (Software as a Service) delivery models revolutionizing how companies access and leverage data analytics capabilities. This shift represents a fundamental change in how organizations approach data-driven decision making.
Traditional BI solutions required extensive on-premises infrastructure, significant upfront investment, and specialized IT teams for implementation and maintenance. These barriers often limited BI adoption to large enterprises with substantial resources.
The emergence of BI SaaS platforms has democratized access to sophisticated analytics, making powerful data tools available to businesses of all sizes. This cloud-based approach eliminates many of the traditional obstacles while providing enhanced flexibility and scalability.
Today’s business landscape demands agility and rapid insights from data. SaaS BI solutions meet these needs by offering quick deployment, regular updates, and accessibility from anywhere—creating opportunities for organizations to make faster, better-informed decisions without the traditional technical overhead.
Core Components of SaaS BI Solutions
Modern SaaS BI platforms integrate several essential components to deliver comprehensive analytics capabilities. These systems function through a multi-layered architecture designed for efficiency and scalability.
At the foundation lies data integration capabilities that connect to various sources including databases, applications, and cloud services. These connectors serve as the pipeline that feeds information into the BI system, often employing ETL (Extract, Transform, Load) processes to prepare data for analysis.
The data storage layer typically leverages cloud-based data warehouses or data lakes that efficiently manage vast information volumes while maintaining performance. This represents a significant advantage over traditional on-premises solutions that often struggled with scalability.
Advanced visualization tools transform raw data into interactive dashboards and reports, making complex information accessible and actionable. These visual interfaces allow users to explore data relationships and patterns that might otherwise remain hidden.
Many solutions incorporate AI and machine learning capabilities that can identify trends, anomalies, and potential opportunities automatically. This predictive functionality extends the value of BI beyond reporting on past performance to anticipating future outcomes.
The SaaS Delivery Model Advantage
The SaaS delivery model provides substantial benefits for organizations implementing business intelligence solutions. This approach fundamentally changes the economics and accessibility of advanced analytics.
With traditional BI systems, companies faced significant upfront capital expenditures for hardware, software licenses, and implementation services. SaaS BI transforms this into a predictable operational expense through subscription-based pricing models, typically charged monthly or annually per user.
Maintenance responsibilities shift from the customer to the service provider, who manages infrastructure, security updates, and performance optimization. This allows organizations to focus resources on extracting value from data rather than managing technical complexities.
The cloud-based architecture enables automatic updates and enhancements without disrupting operations. New features and capabilities become immediately available to all subscribers, ensuring organizations always have access to the latest innovations without complex upgrade processes.
According to a Gartner study, organizations using SaaS BI solutions typically achieve 40-60% lower total cost of ownership compared to on-premises alternatives when factoring in implementation, maintenance, and upgrade costs over a 3-5 year period.
Implementation Process for SaaS BI
Implementing a SaaS BI solution follows a structured process designed to ensure successful deployment and adoption. This typically begins with a thorough needs assessment to identify specific business requirements, key performance indicators, and desired outcomes.
The next stage involves data source identification and connection, where organizations map available data assets and establish integration pathways. This critical step determines what information will feed into the analytics system and may require addressing data quality or format issues.
Most vendors offer guided setup processes with templates and wizards to accelerate implementation. These tools help configure user permissions, create initial dashboards, and establish reporting frameworks aligned with business objectives.
User training and change management strategies are essential for driving adoption. The most successful implementations include hands-on workshops, role-specific training materials, and internal champions who can provide ongoing support.
According to a Forrester Research report, the average implementation timeline for SaaS BI platforms ranges from 2-8 weeks, compared to 6-12 months for traditional on-premises solutions—demonstrating the significant time-to-value advantage of the cloud-based approach.
Data Security and Compliance in SaaS BI
Data security represents a primary concern for organizations considering SaaS BI solutions. Reputable providers implement multiple protection layers, including encryption for data in transit and at rest, robust access controls, and regular security audits.
Many SaaS BI platforms now offer advanced security features such as single sign-on (SSO) integration, multi-factor authentication, and granular permission systems that control exactly what information each user can access. These capabilities help address concerns about housing sensitive business data in cloud environments.
Compliance certifications such as SOC 2, ISO 27001, and industry-specific standards (like HIPAA for healthcare or PCI DSS for payment processing) provide independent verification of security practices. Organizations should verify that their BI provider maintains relevant certifications for their industry.
Data residency options allow organizations to specify geographic locations where their information is stored, helping address regulatory requirements in regions with strict data sovereignty laws like the European Union’s GDPR or California’s CCPA.
Regular penetration testing and vulnerability assessments conducted by third-party security firms provide additional assurance that appropriate safeguards are in place. Leading vendors typically publish security whitepapers detailing their protection measures and compliance frameworks.
Key Features of Modern SaaS BI Platforms
Today’s SaaS BI solutions offer sophisticated capabilities that extend far beyond basic reporting. Self-service analytics tools empower business users to create customized visualizations and reports without requiring technical expertise or IT department assistance.
Interactive dashboards provide real-time visibility into key performance indicators with drill-down capabilities that allow users to explore the underlying data. These interfaces support both high-level overview metrics and detailed analysis of specific trends or anomalies.
Ad-hoc analysis tools enable users to ask spontaneous questions of their data through intuitive interfaces, often employing natural language processing so queries can be phrased in everyday business terminology rather than technical database language.
Mobile optimization ensures that insights are accessible across devices, allowing decision-makers to monitor performance metrics and respond to changing conditions even when away from their desks. This capability has become increasingly important as remote work becomes more prevalent.
Data storytelling features help transform raw analytics into compelling narratives that communicate insights effectively throughout the organization. These tools combine visualizations with contextual information to explain the significance of data trends and their business implications.
Integration Capabilities with Existing Systems
Effective SaaS BI platforms provide extensive integration options to connect with existing business systems and data sources. This connectivity is crucial for creating a comprehensive analytics environment.
Pre-built connectors for popular business applications such as Salesforce, SAP, Oracle, and Microsoft Dynamics allow for straightforward data extraction without complex custom development. These integrations typically refresh automatically on scheduled intervals to maintain current information.
API frameworks enable developers to create custom connections to proprietary or specialized systems when standard connectors aren’t available. These programmatic interfaces support bidirectional data flow, allowing analytics to both consume and provide information to other applications.
Many platforms support data blending capabilities that combine information from multiple sources into unified analyses. This feature allows organizations to correlate data across departmental boundaries—revealing relationships between marketing activities and sales outcomes, for example.
Webhook functionality enables event-driven integration scenarios where specific data changes or threshold violations can trigger actions in other systems. This automation helps organizations respond more quickly to emerging trends or issues identified through analytics.
Pricing Models and Cost Considerations
SaaS BI solutions typically employ several pricing structures that organizations should carefully evaluate against their specific needs. Understanding these models is essential for accurately forecasting costs and maximizing return on investment.
The most common approach is user-based pricing, where companies pay a monthly or annual fee per active user. This model often includes different user types (creators vs. viewers) with varying price points reflecting their access levels and capabilities.
Some vendors offer data-volume pricing based on the amount of information processed or stored, which may be more economical for organizations with few users but large datasets. This approach typically includes tiered pricing with increasing costs as data volumes grow.
Feature-based tiers provide different capability sets at various price points, allowing organizations to select packages aligned with their needs. Entry-level tiers might include basic reporting and visualization, while premium tiers add advanced analytics, AI capabilities, and greater customization options.
Hidden costs can significantly impact total expenditure. Organizations should consider potential charges for additional storage, premium support, API calls, custom development, and training when comparing solutions. Many vendors provide ROI calculators to help prospective customers estimate both costs and potential benefits.
Case Study: Retail Implementation Success
A mid-sized retail chain with 75 locations struggled with fragmented data across point-of-sale systems, inventory management software, and e-commerce platforms. This fragmentation prevented comprehensive analysis of customer behavior and operational efficiency.
By implementing a SaaS BI solution, the retailer consolidated these disparate data sources into a unified analytics environment. Store managers received daily dashboards showing sales performance, inventory levels, and staffing efficiency metrics, while executives gained cross-location comparisons and trend analysis.
The implementation process took only six weeks from contract signing to initial dashboard deployment, with the retailer citing the cloud-based approach as key to the rapid timeline. The subscription-based model required only $65,000 in first-year costs compared to a $380,000 estimate for an equivalent on-premises solution.
Within three months, the retailer identified inventory optimization opportunities that reduced carrying costs by 14%, while personalized marketing campaigns based on customer purchase patterns increased average transaction value by 8.5%. The company’s CEO noted, "We’ve moved from gut-based decisions to data-driven strategies, which has transformed our ability to respond to market changes."
This success demonstrates how SaaS BI can provide rapid time-to-value with minimal upfront investment, particularly for organizations with distributed operations and multiple data sources.
Advanced Analytics and AI Integration
Modern SaaS BI platforms increasingly incorporate artificial intelligence and machine learning capabilities that extend functionality beyond traditional analytics. These advanced features transform how organizations interact with and derive value from their data.
Predictive analytics algorithms can forecast future trends based on historical patterns, enabling proactive decision-making rather than reactive responses. For example, retailers can anticipate seasonal demand fluctuations with greater precision, optimizing inventory levels and staffing accordingly.
Natural language processing allows users to query data using conversational language rather than technical syntax. This capability significantly lowers the skills barrier, enabling even non-technical employees to extract insights by asking questions like "What were our top-selling products in the Northeast region last quarter?"
Anomaly detection systems automatically identify unusual patterns that might indicate opportunities or problems requiring attention. These algorithms continuously monitor data streams, alerting relevant stakeholders when metrics deviate significantly from expected values.
Automated insight generation tools can examine data relationships to discover meaningful patterns without human direction. Rather than requiring analysts to formulate specific questions, these systems proactively identify correlations and potential causal relationships that might otherwise remain undiscovered.
Scalability and Performance Considerations
SaaS BI platforms offer significant advantages in scalability compared to traditional on-premises solutions. Cloud-based architectures can dynamically adjust computing resources based on current processing demands, ensuring consistent performance even as data volumes or user numbers increase.
Most providers employ distributed processing frameworks like Hadoop or Spark that divide analytical workloads across multiple servers. This parallel processing approach enables complex calculations against massive datasets without the performance degradation typical in single-server environments.
In-memory analytics capabilities dramatically accelerate query response times by keeping frequently accessed data in RAM rather than reading from slower disk storage. This technology enables near-instantaneous dashboard updates and interactive data exploration even with large information sets.
Caching mechanisms store the results of common queries, further enhancing performance by eliminating redundant processing. These systems intelligently refresh cached results when underlying data changes, balancing performance optimization with accuracy requirements.
As organizations grow, SaaS solutions can easily accommodate expanding needs without hardware upgrades or complex reconfiguration. This elasticity represents a significant advantage over traditional systems that often require substantial infrastructure investments to handle increased scale.
User Adoption and Training Strategies
Successful BI implementation depends heavily on user adoption, which requires thoughtful strategy and ongoing support. The most sophisticated analytics tools deliver little value if employees don’t incorporate them into daily decision-making processes.
Effective organizations typically begin with executive sponsorship that clearly communicates the strategic importance of data-driven decision making. This top-down commitment establishes analytics as a priority and helps overcome resistance to changing established workflows.
Role-based training programs provide targeted instruction focused on specific use cases relevant to each user group. Marketing teams learn visualization techniques for campaign performance analysis, while finance departments focus on forecast modeling and variance reporting relevant to their responsibilities.
Internal champions or "power users" within each department can provide peer-level support and encouragement. These individuals receive advanced training and serve as resources for colleagues, helping troubleshoot issues and demonstrate practical applications relevant to their specific business context.
Many organizations implement gamification elements that recognize and reward active engagement with analytics tools. Leaderboards highlighting dashboard creation, insights shared, or decisions influenced by data analysis create positive reinforcement that drives continued adoption throughout the organization.
Future Trends in SaaS BI
The SaaS BI landscape continues to evolve rapidly, with several emerging trends shaping future capabilities. Understanding these developments helps organizations select platforms with long-term viability and competitive advantage potential.
Augmented analytics represents a significant advancement, using AI to automate data preparation, insight discovery, and visualization creation. These systems will increasingly function as intelligent assistants that guide users to relevant insights rather than requiring them to manually explore data relationships.
Edge analytics processing at data collection points rather than centralizing all information will become increasingly important as Internet of Things (IoT) deployments generate massive data volumes. This approach reduces latency for time-sensitive decisions and minimizes bandwidth requirements for data transmission.
Collaborative BI features are expanding beyond simple dashboard sharing to include annotation, discussion threads, and workflow integration. These capabilities transform analytics from isolated activities into team-based processes that incorporate diverse perspectives and expertise.
Embedded analytics integration directly into operational business applications will continue accelerating, bringing insights to users within their normal workflows rather than requiring them to switch to separate BI tools. This contextual approach significantly improves the likelihood that analytics will influence daily decisions.
According to Gartner research, by 2025, data storytelling will be the most widespread way of consuming analytics, with 75% of stories automatically generated by AI systems that identify the most relevant insights and present them in narrative form optimized for human understanding.
Selecting the Right SaaS BI Solution for Your Organization
Choosing the optimal SaaS BI platform requires systematic evaluation aligned with specific organizational needs. This decision-making process should consider both current requirements and anticipated future needs.
Begin by documenting key use cases and specific business questions the solution must address. This functional analysis provides evaluation criteria more meaningful than generic feature comparisons and helps prioritize capabilities based on actual business impact.
Technical compatibility with existing data sources and systems should be thoroughly assessed. Evaluate both standard connectors and API capabilities to ensure the platform can access all relevant information without costly custom development.
User experience significantly impacts adoption rates and should be evaluated from the perspective of different stakeholder groups. Request trial accounts that allow potential users to test the interface with actual business data rather than vendor-provided demonstrations.
Vendor stability and market position warrant careful consideration given the critical nature of BI infrastructure. Examine the provider’s financial health, investment in research and development, product roadmap, and customer retention metrics to assess long-term viability.
Total cost of ownership calculations should include subscription fees, implementation services, potential customization, training requirements, and internal administration costs. Request detailed pricing scenarios that reflect anticipated growth in users, data volume, and functional requirements.
Overcoming Common Implementation Challenges
Despite their advantages, SaaS BI deployment projects face several common obstacles that organizations should proactively address. Awareness of these challenges enables more effective planning and risk mitigation.
Data quality issues frequently surface during implementation as previously isolated information sources are consolidated. Establishing data governance procedures and cleansing processes before migration helps prevent analytics based on inaccurate or inconsistent information.
Integration complexities with legacy systems sometimes exceed initial estimates, particularly with older applications lacking modern API capabilities. Pilot projects connecting key data sources can validate assumptions and identify potential complications before full-scale implementation.
Change management resistance often emerges when implementing new analytics tools, particularly among users comfortable with existing reporting methods. Early involvement of end-users in the selection process and clear communication about how the new system addresses their specific pain points can reduce resistance.
Performance expectations sometimes create satisfaction issues when users with limited technical understanding expect instantaneous results from complex queries against massive datasets. Setting realistic expectations and optimizing high-priority reports can help manage perceptions during the transition period.
Skill gaps among internal teams frequently cause adoption challenges. Supplementing vendor training with internal knowledge-sharing sessions and easily accessible reference materials helps users develop confidence and proficiency with the new tools.
Maximizing Your Business Intelligence Investment
To derive maximum value from SaaS BI solutions, organizations should implement strategic approaches that extend beyond initial deployment. These practices help transform analytics from a reporting tool into a genuine competitive advantage.
Establish clear metrics for measuring success based on business outcomes rather than technical implementation milestones. These might include improvements in decision-making speed, operational efficiency, revenue growth, or cost reduction directly attributable to analytics insights.
Create a formal data strategy that identifies high-value information assets, establishes governance procedures, and prioritizes analytics initiatives based on potential business impact. This roadmap ensures resources focus on opportunities with the greatest return on investment.
Executive dashboards should connect analytics directly to strategic objectives, demonstrating how performance metrics relate to organizational goals. This alignment helps sustain leadership commitment and resource allocation for analytics initiatives.
Implement a continuous improvement cycle where user feedback regularly informs dashboard refinements and new report development. This iterative approach ensures analytics tools evolve alongside changing business needs and user sophistication.
Communities of practice bringing together analytics users from different departments create valuable cross-functional perspectives and accelerate knowledge sharing. These groups can identify new use cases, share successful techniques, and provide peer support that enhances the organization’s overall analytical capabilities.
Transform Your Business with SaaS BI and AI-Powered Communication
Business Intelligence SaaS solutions have fundamentally changed how organizations leverage data for strategic advantage, providing powerful analytics capabilities without the traditional barriers of complex infrastructure and specialized expertise. As we’ve explored throughout this article, these platforms offer remarkable flexibility, scalability, and rapid implementation advantages compared to legacy systems.
For businesses seeking to extend this data-driven approach to customer communications, Callin.io provides an innovative solution that complements your BI strategy. This advanced platform leverages artificial intelligence to transform phone interactions through intelligent automation that maintains the personal touch customers expect.
Callin.io’s AI phone agents can handle everything from answering frequently asked questions to scheduling appointments and qualifying leads—all while integrating with your existing systems to maintain data consistency. The platform offers affordable entry points with a free account option that includes test calls and basic features, making it accessible for organizations of any size.
By combining robust business intelligence with AI-powered communication through Callin.io, you create a comprehensive ecosystem where data-driven insights directly inform and enhance customer interactions. Visit Callin.io today to discover how intelligent communication tools can complement your business intelligence strategy and drive measurable improvements in customer experience and operational efficiency.
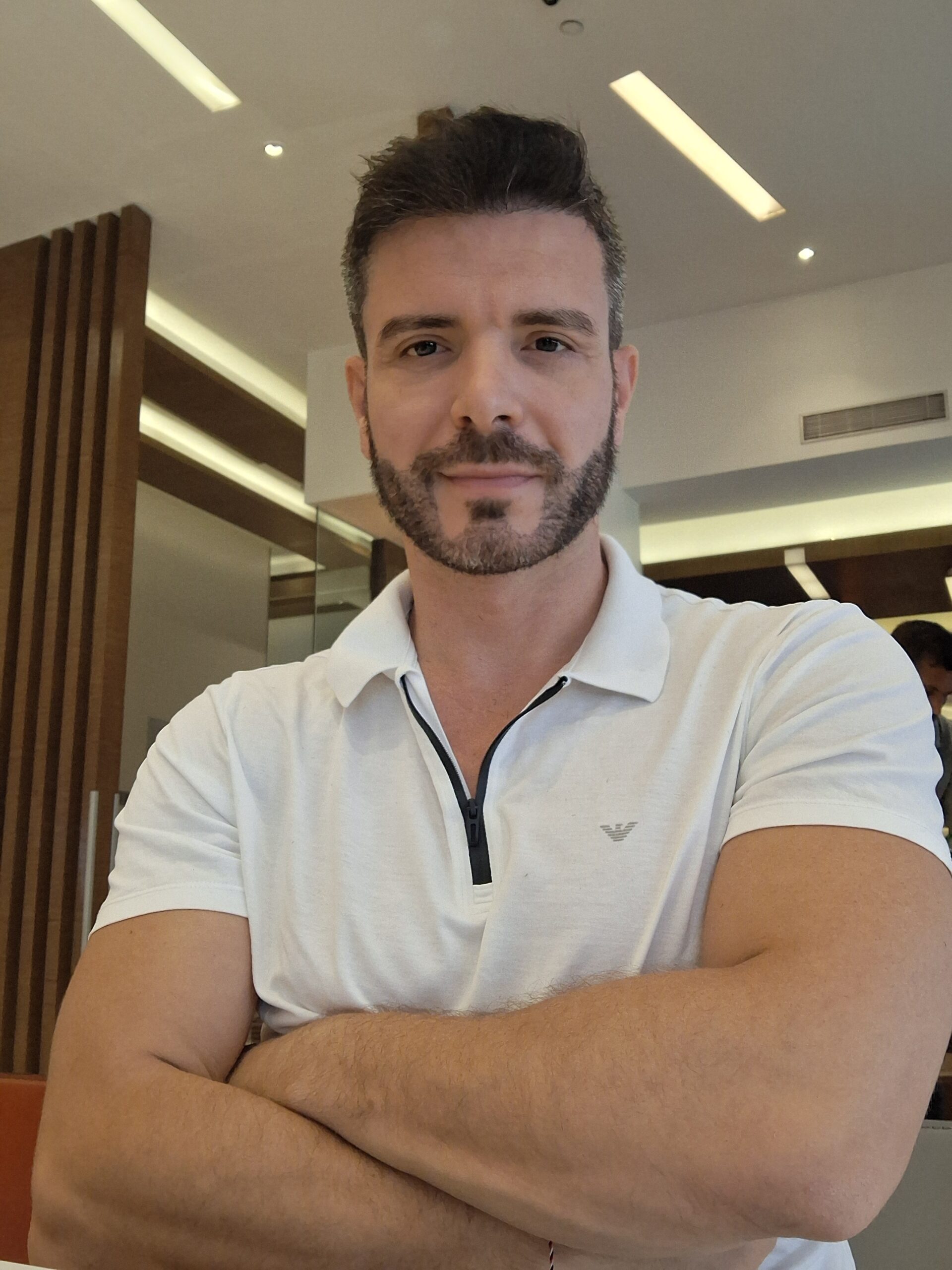
Helping businesses grow faster with AI. 🚀 At Callin.io, we make it easy for companies close more deals, engage customers more effectively, and scale their growth with smart AI voice assistants. Ready to transform your business with AI? 📅 Let’s talk!
Vincenzo Piccolo
Chief Executive Officer and Co Founder