The Evolution of Sales Forecasting Through AI
Sales forecasting has transformed dramatically in recent years, moving beyond traditional spreadsheets and gut feelings to embrace sophisticated artificial intelligence solutions. Today’s AI-powered forecasting tools analyze vast amounts of historical data, customer interactions, and market trends to predict future sales with remarkable accuracy. Unlike conventional methods that relied heavily on manual calculations and subjective assessments, AI tools for sales forecasting leverage machine learning algorithms that continuously improve over time.
These intelligent forecasting systems can detect subtle patterns in sales data that human analysts might miss, identifying seasonality effects, customer behavior shifts, and emerging market trends. They excel at processing complex variables simultaneously, from macroeconomic indicators to granular customer engagement metrics.
The adoption of AI forecasting tools has accelerated particularly in high-velocity sales environments where rapid market changes demand equally swift adaptations to sales strategies. Organizations embracing these technologies are finding themselves better equipped to allocate resources efficiently, manage inventory proactively, and optimize their sales pipelines with precision.
Key Benefits of AI-Powered Sales Forecasting
Implementing AI for sales forecasting delivers multiple transformative advantages that directly impact a company’s bottom line. Unprecedented accuracy stands as the most significant benefit, with modern AI systems reducing forecast errors by 30-50% compared to traditional methods. This precision enables businesses to make confident decisions about inventory management, production scheduling, and resource allocation.
AI forecasting tools also provide real-time adaptability, continuously adjusting predictions as new data becomes available. This dynamic capability proves invaluable during market disruptions or unexpected events, allowing sales teams to pivot strategies quickly.
Another key advantage is time efficiency – tasks that once took sales teams days or weeks to complete can now be executed in minutes or hours. The automation of data collection, cleaning, and analysis frees sales professionals to focus on relationship-building and deal-closing activities.
AI forecasting also enables granular insights at multiple levels – from company-wide projections down to individual product performance and specific customer segments. These multi-dimensional forecasts help organizations identify high-potential opportunities and anticipate potential challenges before they materialize.
According to research by Gartner, companies using AI-powered sales forecasting have reported up to 20% increases in revenue and significant improvements in operational efficiency.
Essential Features in Top AI Forecasting Tools
When evaluating AI tools for sales, certain critical features distinguish exceptional forecasting solutions from merely adequate ones. Robust data integration capabilities should top your checklist – the best tools seamlessly connect with your CRM, ERP, market intelligence platforms, and other data sources to create comprehensive forecasting models.
Advanced analytics engines powered by machine learning algorithms represent another must-have feature. Look for tools that employ multiple algorithmic approaches, including time series analysis, regression modeling, and neural networks, to generate multi-scenario forecasts.
Intuitive visualization components transform complex data into actionable insights through customizable dashboards, interactive charts, and drill-down capabilities. These visual elements help stakeholders quickly grasp prediction trends and identify areas requiring attention.
Scenario planning functionality enables sales teams to test different assumptions and market conditions, creating "what-if" analyses that prepare organizations for various potential futures. This feature proves particularly valuable during uncertain economic times.
Automated anomaly detection identifies unusual patterns or outliers that might indicate emerging opportunities or potential problems, alerting teams to investigate further before these signals impact actual sales performance.
Leading solutions like Salesforce Einstein and Microsoft Dynamics 365 Sales Insights exemplify these essential features, providing comprehensive AI-powered forecasting capabilities.
Data Requirements for Effective AI Forecasting
The quality and comprehensiveness of your data foundation directly determine the accuracy of AI-based sales forecasts. At minimum, effective forecasting requires historical sales data spanning multiple business cycles – ideally 2-3 years worth – to identify seasonal patterns and long-term trends. This historical record should include transaction details, volumes, prices, and associated timestamps.
Customer data forms another critical component, encompassing demographic information, purchase history, engagement metrics, and behavioral signals. The more detailed these customer profiles, the better your AI system can segment and predict purchasing patterns.
Market intelligence provides essential context for forecasts, incorporating competitor activities, industry trends, and macroeconomic indicators that influence buying decisions. External datasets from sources like Bloomberg or industry-specific providers can significantly enhance forecast accuracy.
Sales pipeline information – including prospect details, opportunity stages, and sales activities – helps the AI system evaluate near-term sales potential and conversion likelihoods. Integrating your conversational AI interactions with prospects can provide additional behavioral signals.
Data quality matters tremendously – inconsistent, incomplete, or inaccurate information will undermine even the most sophisticated forecasting algorithms. Implementing robust data governance practices and regular data cleansing processes ensures your AI tools have reliable information to work with.
Remember that AI forecasting systems improve over time as they ingest more data, so establishing comprehensive data collection practices today will yield increasingly accurate predictions tomorrow.
Implementation Process: Setting Up AI Forecasting
Successfully deploying AI for forecasting requires a structured approach that balances technical considerations with organizational factors. Begin with a clear objectives assessment – define exactly what you want your forecasting to achieve, whether that’s improving accuracy, speeding up the process, enhancing granularity, or some combination of these goals.
Data preparation represents the most time-consuming yet crucial step in implementation. This involves auditing existing data sources, identifying gaps, establishing integration pathways, and cleaning historical data to ensure quality. Many organizations underestimate this phase, which can require 50-70% of the total implementation effort.
Tool selection should align with your specific business requirements and existing technology ecosystem. Consider factors like integration capabilities with your current CRM, user interface complexity, customization options, and scalability needs. Many vendors offer proof-of-concept trials that allow you to test compatibility with your data and processes.
Phased rollout typically works better than an all-at-once approach. Start with a specific product line, sales team, or geographic region to validate the system before expanding. This controlled implementation helps identify and address issues with minimal disruption.
User training proves essential for adoption and effective utilization. Beyond technical training on the tool itself, focus on helping your team understand the forecasting methodology, interpret results correctly, and know when human judgment should override AI recommendations.
Organizations like Deloitte offer specialized consulting services for AI forecasting implementations, which can be valuable for complex deployments.
Integration with Existing Sales Tech Stack
For AI forecasting tools to deliver maximum value, they must communicate seamlessly with your current sales technology ecosystem. CRM integration forms the foundation of effective forecasting, with bidirectional data flows ensuring your AI tools have access to current pipeline information while pushing forecast insights back to where sales teams work daily.
Marketing automation platform connections provide critical top-of-funnel signals that help predict future pipeline development. By integrating data about campaign performance, lead scoring, and engagement metrics, your forecasting becomes more forward-looking rather than solely relying on existing opportunities.
ERP and financial system integration brings important historical transaction data, pricing information, and inventory status into your forecasting models. This integration helps align sales forecasts with operational realities and financial planning.
Business intelligence tools often serve as the visualization and reporting layer for AI forecasts, making integration between your forecasting engine and BI platforms essential for effective communication of insights.
API flexibility should be a key consideration when evaluating forecasting solutions. Open APIs allow for custom integrations with specialized tools in your stack and facilitate data sharing across platforms. Tools like Zapier or custom middleware can help bridge integration gaps when native connections aren’t available.
Many organizations benefit from working with integration specialists who understand both the technical requirements and business processes involved. Preserving data integrity across systems while enabling smooth information flow requires careful planning and ongoing management.
Accuracy Measurement and Continuous Improvement
Implementing robust accuracy metrics and feedback mechanisms ensures your AI forecasting system continuously improves over time. Forecast variance analysis should be conducted regularly, comparing predicted versus actual results across different time horizons, product categories, and customer segments to identify systematic biases or blind spots.
Mean Absolute Percentage Error (MAPE) provides a standardized accuracy measurement that works across different scales and time periods. Tracking MAPE trends over time helps quantify forecasting improvement and benchmark performance against industry standards.
Root cause analysis of significant forecasting misses yields valuable insights for system refinement. Was the error due to data quality issues, algorithm limitations, unexpected market events, or some combination of factors? Each analysis provides learning opportunities.
Model retraining schedules should align with your business cycles and data volatility. Some industries require weekly algorithm updates, while others can operate effectively with monthly or quarterly retraining. Your implementation partner can recommend appropriate intervals based on your specific situation.
Feedback loops with sales teams prove invaluable for improvement. Frontline salespeople often possess contextual knowledge about deals, customers, and market conditions that data alone can’t capture. Creating structured processes for incorporating this qualitative intelligence enhances model accuracy.
According to research by McKinsey & Company, organizations that implement systematic forecast improvement processes typically achieve 10-15% greater forecast accuracy year over year compared to those with static approaches.
Overcoming Common Implementation Challenges
Even with careful planning, organizations often encounter hurdles when adopting AI forecasting tools. Data quality and accessibility issues frequently top the list of challenges. Historical data may be scattered across systems, contain inconsistencies, or lack the granularity needed for effective modeling. Addressing these foundational data problems before full implementation saves significant frustration later.
Stakeholder skepticism can derail even technically sound implementations. Sales leaders accustomed to traditional forecasting methods may resist trusting "black box" AI predictions. Overcoming this requires transparency about methodology, demonstrating clear accuracy improvements, and allowing for a transition period where both approaches run in parallel.
Integration complexity often exceeds initial expectations, particularly in organizations with numerous legacy systems or custom sales processes. Allocating sufficient IT resources and possibly engaging specialized integration partners helps navigate these technical challenges.
User adoption barriers typically emerge when forecasting tools feel disconnected from daily workflows or require substantial additional effort. Embedding AI forecasts directly into existing systems (like your CRM) and automating data collection wherever possible maximizes adoption.
Interpretation challenges arise when users don’t fully understand how to act on AI-generated forecasts. Effective training should cover not just tool operation but also how to translate forecasts into strategic decisions and tactical actions.
Organizations like Boston Consulting Group have found that companies addressing these challenges proactively achieve implementation success rates nearly three times higher than those taking a reactive approach.
Best Practices for Managing AI Sales Forecasts
Maximizing the value of your AI forecasting investment requires thoughtful management practices beyond initial implementation. Establish clear forecast governance with defined roles, responsibilities, and decision rights regarding forecast adjustments, override protocols, and model updates. This structure ensures accountability while preserving flexibility.
Implement a forecast review cadence that aligns with your sales cycles and business planning needs. These structured reviews should examine both aggregate accuracy and specific segments where predictions deviated significantly from actuals.
Develop scenario-based forecasting capabilities that allow your organization to prepare for multiple potential futures rather than relying on a single prediction. Leading companies typically maintain base, optimistic, and pessimistic scenarios with specific trigger events identified for each.
Balance AI and human judgment through clear guidelines about when manual adjustments are appropriate. While AI excels at processing historical patterns, humans bring valuable context about emerging market changes, competitive moves, and relationship dynamics that may not yet appear in the data.
Create actionable forecast presentations that translate complex predictions into clear business implications. The best forecast visualizations highlight not just the numbers but also key drivers, risks, and recommended actions for different stakeholders.
Maintain documentation of model changes and performance over time to build institutional knowledge about what works in your specific market context. This historical record proves invaluable when onboarding new team members or troubleshooting forecast issues.
Research by Forrester indicates that organizations following these management best practices realize 25-35% greater business value from their AI forecasting investments compared to those focused solely on technical implementation.
Case Study: Retail Success with AI Forecasting
A major North American retail chain provides an instructive example of AI forecasting’s transformative potential. Facing increasing competition from e-commerce giants and struggling with inventory imbalances across its 500+ locations, the retailer implemented an AI forecasting solution that dramatically improved its sales prediction accuracy.
Prior to implementation, the company relied on a combination of spreadsheet-based historical averages and store manager intuition, resulting in a 42% average forecast error rate. Store-level stock issues cost an estimated $15 million annually in lost sales and markdown requirements.
The retailer selected an AI forecasting platform that integrated with their existing POS system, ERP, and marketing automation tools. The implementation team prioritized three years of historical transaction data, enriched with local event calendars, weather patterns, demographic data, and competitor pricing information.
After a four-month implementation across a 50-store pilot group, forecast accuracy improved to an 18% error rate – a 57% improvement. More importantly, the granular nature of the AI forecasts allowed for store-specific inventory adjustments and marketing investments.
The most significant business impacts included:
- 22% reduction in out-of-stock situations
- 15% decrease in excess inventory carrying costs
- 8% increase in same-store sales compared to control group
- Store manager time spent on forecasting reduced from 5 hours to 1 hour weekly
The retailer has subsequently expanded the AI forecasting system enterprise-wide and integrated it with their AI phone agents for customer service, creating a closed-loop system where customer feedback directly informs future forecasts.
Industry-Specific Applications of AI Forecasting
While AI forecasting offers benefits across sectors, implementation approaches and use cases vary significantly by industry. In pharmaceutical sales, forecasting tools incorporate prescription trends, physician adoption patterns, and regulatory changes to predict both new product launches and existing medication sales cycles. Companies like Pfizer have reported forecast improvements of 35-40% when using AI-enhanced methods.
The technology sector leverages AI forecasting to navigate rapid product lifecycles and complex channel dynamics. Hardware manufacturers particularly benefit from component-level forecasting that helps manage supply chain constraints while software companies use AI to predict subscription renewals and expansion opportunities.
Financial services organizations apply AI forecasting to predict everything from loan origination volumes to wealth management product adoption. The most sophisticated implementations incorporate economic indicators and regulatory environment assessments to adjust forecasts dynamically.
Manufacturing firms use AI sales forecasting to align production scheduling and raw material procurement with anticipated demand. This synchronization reduces carrying costs while ensuring product availability, with companies like Siemens reporting working capital improvements of 15-20% through improved forecast accuracy.
Hospitality and travel businesses face unique forecasting challenges with highly perishable inventory and extreme price elasticity. AI tools that incorporate competitive pricing data, search trends, and macroeconomic indicators have proven particularly valuable in these sectors.
Each industry requires specific data sources and modeling approaches, making specialized forecasting tools or customized implementations increasingly common. Industry-specific AI forecasting solutions like those from Anaplan have emerged to address these unique requirements.
The Future of AI Sales Forecasting
As AI forecasting technology continues to mature, several emerging trends promise to further revolutionize sales prediction capabilities. Real-time forecasting represents a significant evolution, with systems that continuously update predictions as new data arrives rather than generating periodic forecasts. This dynamic capability proves particularly valuable in fast-moving markets and crisis situations.
Prescriptive intelligence extends beyond prediction to recommend specific actions that optimize forecast outcomes. These advanced systems might suggest ideal customer targeting, pricing adjustments, or resource allocations to maximize sales potential based on current forecast trajectories.
Natural language interfaces will make sophisticated forecasting capabilities accessible to non-technical users through conversational queries. Sales leaders will simply ask questions like "How will Q3 performance affect our annual targets?" and receive instant, context-aware responses.
External data integration will expand dramatically, with forecasting systems automatically incorporating relevant signals from thousands of sources – from social media sentiment to satellite imagery of retail parking lots – to enhance prediction accuracy.
Explainable AI approaches will address the "black box" problem that creates trust issues with current systems. Next-generation forecasting tools will clearly articulate which factors most influenced a particular prediction and why certain outcomes are considered likely.
Autonomous forecasting represents perhaps the most transformative development – systems that not only generate predictions but independently take actions based on those forecasts, from adjusting digital advertising spend to modifying product pricing on e-commerce platforms.
Research from MIT Technology Review suggests that organizations investing in these advanced capabilities today will establish significant competitive advantages as AI forecasting becomes a standard business practice.
Security and Compliance Considerations
As organizations entrust sensitive sales data to AI forecasting systems, security and compliance issues demand careful attention. Data privacy regulations like GDPR, CCPA, and industry-specific requirements establish strict guidelines for handling customer information used in forecasting models. Ensuring your AI tools comply with applicable regulations requires thorough vendor assessment and proper data governance practices.
Intellectual property protection concerns arise when proprietary sales methodologies, pricing strategies, and customer insights are processed through external AI systems. Clear contractual provisions regarding data ownership and usage rights help mitigate these risks.
Access management within your organization determines who can view different forecast levels and underlying data. Implementing role-based permissions ensures sensitive information remains appropriately restricted while necessary insights reach decision-makers.
Audit trails provide essential documentation for both compliance verification and system accountability. Look for forecasting tools that maintain comprehensive logs of data inputs, model changes, manual adjustments, and user interactions.
Vendor security practices merit close examination during the selection process. Cloud-based forecasting solutions should employ robust encryption, regular security audits, and transparent incident response procedures. Request SOC 2 compliance documentation and ask about penetration testing protocols.
Data residency requirements in certain industries or regions may limit where your sales information can be stored and processed. Confirm that your chosen forecasting platform offers appropriate geographical options for data handling.
Organizations like IBM Security provide specialized assessment services for AI implementations that help identify and address potential vulnerabilities before they lead to data breaches or compliance violations.
Cost-Benefit Analysis of AI Forecasting Solutions
Evaluating the financial implications of AI forecasting investments requires examining both direct costs and expected returns. Implementation expenses typically include software licensing, professional services, data preparation, integration work, and training costs. Depending on your organization’s size and complexity, these initial investments range from $50,000 for midsize implementations to several million for enterprise-wide deployments.
Ongoing operational costs encompass subscription fees (typically $50-200 monthly per user for cloud-based solutions), internal administration, periodic model tuning, and possible data acquisition expenses. Budget for approximately 20-30% of initial implementation cost as annual maintenance.
Against these investments, organizations typically measure returns in several categories. Inventory optimization often delivers the most quantifiable benefits, with companies reporting 15-30% reductions in excess stock and 20-40% decreases in stockout situations. For a business with $10 million in inventory, this optimization can free $1.5-3 million in working capital.
Resource allocation improvements generate significant returns through better sales territory design, more effective marketing spend, and optimized sales team deployment. Companies implementing AI forecasting report 10-15% greater sales productivity from the same resources.
Strategic decision quality improves when leaders have accurate forecasts for evaluating new market entries, acquisition opportunities, and investment timing. While harder to quantify, the competitive advantage from better-informed decisions often exceeds all other benefits combined.
According to Harvard Business Review, organizations typically achieve positive ROI from AI forecasting implementations within 9-15 months, with mature deployments generating 300-500% returns over three years.
Transforming Your Sales Operations with AI-Powered Insights
Harnessing the full potential of AI forecasting requires rethinking key aspects of your sales operations beyond the forecasting process itself. Sales territory design can be dynamically optimized based on AI-driven opportunity predictions rather than simple geographic divisions or historical performance. This data-driven approach typically improves territory balance by 25-35%.
Quota setting becomes more scientific and equitable when based on AI forecasts that consider market potential, historical patterns, and account-specific opportunities. Sales teams perceive quotas derived from sophisticated models as fairer and more achievable than traditional top-down allocations.
Incentive compensation structures can align more precisely with strategic priorities when informed by granular forecasts. Organizations increasingly use AI predictions to create targeted SPIFs (Sales Performance Incentive Funds) that accelerate growth in high-potential segments.
Sales coaching gains precision when managers can focus on specific opportunities where forecast models identify the greatest swing potential. Rather than general skill development, coaching becomes targeted to deals where intervention will most impact overall performance.
Pipeline management transforms when AI tools highlight which opportunities deserve additional attention based on forecast importance and conversion risk. This prioritization helps sales teams focus on the right deals at the right time.
Collaborative selling improves when all team members share the same data-driven forecast view, eliminating disagreements about priorities and resource allocation. This alignment speeds decision-making and reduces internal friction.
Organizations like Salesforce have documented how these operational transformations, powered by accurate AI forecasting, can increase overall sales productivity by 20-30% while simultaneously improving forecast accuracy.
Your Journey to Enhanced Forecasting Begins Today
Implementing AI-powered sales forecasting represents a significant opportunity to transform your business with data-driven predictions that increase accuracy, save time, and drive better decision-making throughout your organization. As you’ve seen throughout this article, the benefits extend far beyond simply knowing your numbers better – they touch every aspect of sales operations and strategy execution.
Begin your journey by assessing your current forecasting maturity and identifying specific pain points that AI tools could address. Consider starting with a focused pilot in a single product line or region to demonstrate value quickly before expanding.
Remember that successful implementation requires both technical excellence and organizational change management. The most sophisticated AI models deliver limited value if your team doesn’t trust or understand how to act on the insights they provide.
If you’re ready to enhance your sales operations with innovative technology, Callin.io offers powerful AI solutions that can transform how your business communicates with customers. Our AI phone agents can complement your forecasting efforts by capturing valuable customer insights during calls while delivering consistent, high-quality interactions that drive sales performance.
With a free account, you can explore how our intuitive interface makes it easy to configure AI agents that handle appointments, answer FAQs, and even close deals through natural conversations. For more advanced needs, our subscription plans starting at just $30 USD monthly provide Google Calendar integration and CRM capabilities to further enhance your sales ecosystem. Discover more about Callin.io today and take the next step toward data-driven sales excellence.
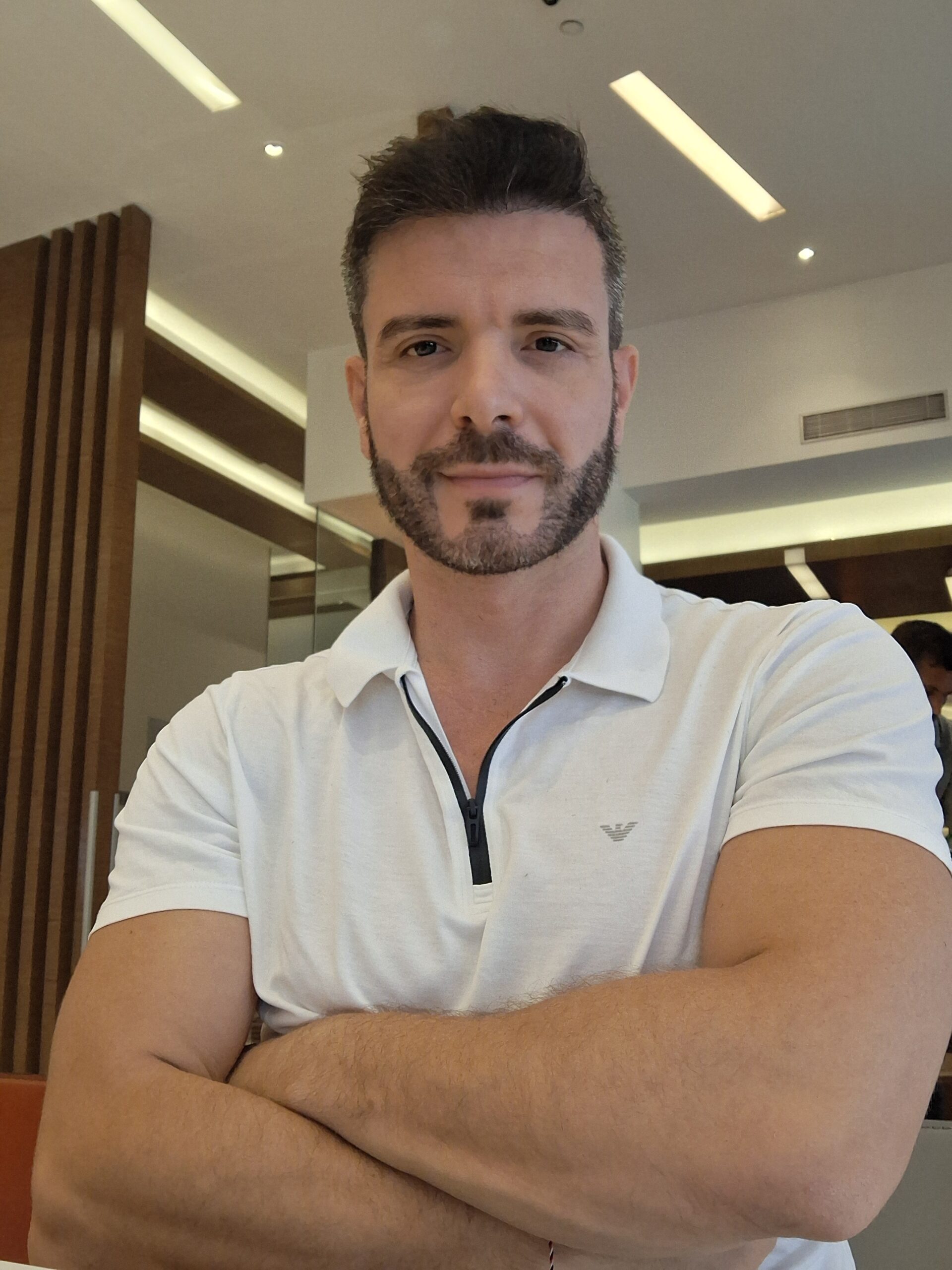
Helping businesses grow faster with AI. π At Callin.io, we make it easy for companies close more deals, engage customers more effectively, and scale their growth with smart AI voice assistants. Ready to transform your business with AI? π Β Letβs talk!
Vincenzo Piccolo
Chief Executive Officer and Co Founder