Understanding Customer Churn: The Silent Business Killer
Customer churn—the rate at which customers stop doing business with a company—is one of the most significant challenges facing businesses today. When customers leave, they take their revenue with them, creating gaps that are expensive and time-consuming to fill. According to research by Bain & Company, increasing customer retention by just 5% can boost profits by 25% to 95%. This stark reality makes churn prediction not just helpful but essential for business sustainability. Traditional methods of tracking customer departures are reactive rather than proactive, only identifying problems after customers have already walked away. This is where AI-powered churn prediction transforms the game, enabling businesses to spot warning signs before customers make the final decision to leave. By leveraging machine learning algorithms that analyze patterns in customer behavior, companies can intervene at critical moments with personalized retention strategies. Our AI voice assistants can help identify these patterns through conversational data analysis.
The True Cost of Customer Attrition
Many businesses underestimate what customer churn truly costs them. Beyond the immediate revenue loss, there are ripple effects that damage long-term business health. The acquisition cost for new customers is typically five times higher than retaining existing ones, according to research by Frederick Reichheld of Bain & Company. Additionally, loyal customers tend to spend more over time—up to 67% more in their third year compared to their first year with a business. When factoring in the negative word-of-mouth from dissatisfied ex-customers (with each telling approximately 9-15 people about their poor experience according to the White House Office of Consumer Affairs), the real cost of churn becomes alarmingly apparent. Companies also lose the investment they’ve made in customer relationships, including onboarding costs, support resources, and marketing expenditures. Using AI-driven prediction models allows businesses to calculate the lifetime value impact of retention, making the business case for churn prevention crystal clear. Our conversational AI solutions help businesses maintain meaningful customer relationships that discourage churn.
How AI Transforms Churn Prediction Accuracy
Traditional churn prediction methods rely on simplistic rules or basic statistical models that often miss the subtle indicators of customer dissatisfaction. AI solutions dramatically improve prediction accuracy by leveraging sophisticated machine learning algorithms that can identify complex patterns invisible to conventional analysis. For example, random forest algorithms can detect non-linear relationships between customer behaviors and churn probability, while deep learning neural networks can simultaneously process hundreds of variables to identify customers at risk. A study by McKinsey found that companies using AI for churn prediction increased their accuracy by 15-35% compared to traditional methods. This improved accuracy translates directly to more effective retention efforts and better resource allocation. Rather than treating all customers the same, businesses can prioritize high-value customers showing early warning signs. Our AI phone calls technology can detect sentiment changes in customer conversations that might indicate increased churn risk.
Key Data Points That Feed Churn Prediction Algorithms
The effectiveness of any AI churn prediction system depends heavily on the quality and breadth of data it analyzes. Successful models incorporate diverse data types from multiple sources to build a comprehensive view of customer health. Behavioral data tracks how customers interact with products or services, including login frequency, feature usage patterns, and engagement metrics. Transactional data examines purchase history, spending patterns, and account changes. Customer service interactions provide valuable insights through support ticket frequency, resolution times, and sentiment analysis of communications. Demographic information helps segment customers for more tailored predictions, while social media sentiment offers external validation of customer satisfaction. The most powerful churn prediction systems even incorporate market and competitive data to understand external factors affecting retention. When combined, these data points create a multidimensional profile that AI can analyze to detect subtle changes in customer behavior that human analysts might miss. Our AI call center solutions can gather and analyze many of these data points during customer interactions.
Machine Learning Models That Power Churn Prediction
Different machine learning approaches offer unique advantages for churn prediction, with the most effective solutions often combining multiple techniques. Logistic regression models provide easily interpretable results and work well with limited data, making them a good starting point. Decision trees excel at identifying clear decision paths that lead to churn, while random forests improve accuracy by combining multiple trees. For complex pattern recognition, gradient boosting machines like XGBoost and LightGBM have shown exceptional performance in churn prediction competitions. When dealing with unstructured data like customer service calls or emails, natural language processing (NLP) techniques can extract sentiment and intent. For extremely large datasets with many variables, deep learning neural networks can discover hidden patterns that simpler models might miss. The most sophisticated solutions implement ensemble approaches that combine predictions from multiple models to achieve greater accuracy than any single algorithm could provide. Our voice AI call center technology uses several of these models to analyze customer conversations for churn indicators.
Real-Time Churn Risk Assessment: From Prediction to Action
Traditional churn analysis operates on historical data, often identifying risk factors too late for effective intervention. Modern AI solutions have shifted toward real-time monitoring that transforms churn prediction from a retrospective analysis into an actionable operational tool. These systems continuously evaluate customer interactions, monitoring behavioral triggers that might indicate dissatisfaction—such as reduced product usage, negative support interactions, or changes in purchasing patterns. When risk indicators reach certain thresholds, the system automatically alerts appropriate team members and may trigger customized retention workflows. For example, a subscription-based software company might receive an alert when a previously active user’s login frequency drops by 50%, prompting a targeted outreach before the customer considers cancellation. Real-time systems can also prioritize alerts based on customer value and churn probability, ensuring businesses focus retention efforts where they’ll have the greatest impact. Our AI phone agent can serve as a front-line tool for immediate response when churn risk is detected.
Predictive Analytics vs. Prescriptive Analytics in Retention Strategy
While predictive analytics tells you which customers are likely to leave, prescriptive analytics takes things a step further by recommending specific actions to prevent their departure. This crucial difference transforms churn prediction from an informational tool into an operational framework for customer retention. Predictive models identify at-risk customers and quantify their churn probability, but prescriptive systems analyze successful retention cases to recommend personalized interventions based on customer characteristics. For instance, a telecommunications company might discover through prescriptive analysis that offering a specific upgrade package retains high-value customers more effectively than a discount. The most advanced systems use reinforcement learning to continuously refine recommendations based on intervention outcomes, creating a feedback loop that progressively improves retention strategies. This approach not only increases retention rates but also optimizes the cost-effectiveness of retention efforts by recommending the minimum intervention necessary to keep each customer. Our AI calling business solutions can implement these prescriptive recommendations through automated outreach campaigns.
Industry-Specific Churn Prediction Applications
Churn prediction needs and methodologies vary significantly across industries, with each sector requiring tailored approaches. In subscription-based businesses like SaaS and streaming services, AI models focus on engagement metrics and feature adoption rates, with successful implementations reducing churn by 10-20% according to studies by Gainsight. The telecommunications industry benefits from analyzing call quality metrics, service interruptions, and competitor pricing, with Bain & Company reporting a 15% churn reduction through targeted retention programs. Financial services institutions leverage AI to track account activity patterns, life event indicators, and product utilization to predict attrition, while e-commerce businesses analyze browsing patterns, return rates, and customer service interactions to identify at-risk customers. Healthcare providers are increasingly using AI to predict patient churn by examining appointment adherence, treatment completion rates, and satisfaction scores. Each industry requires specific data inputs and model customizations, but all benefit from the precision that AI brings to customer retention efforts. Check out our AI conversational solutions for medical offices to see how healthcare providers are reducing patient churn.
Implementing Customer Segmentation for Targeted Retention
Not all customers have equal value or churn for the same reasons, making segmentation a critical component of effective retention strategies. AI-powered segmentation goes beyond traditional demographic groupings to create behavior-based microsegments that share similar usage patterns and churn risks. These sophisticated segmentation models consider factors like customer lifetime value (CLV), purchase frequency, product usage depth, and support interaction history to identify distinct customer groups. For example, a high-value segment showing early warning signs might receive proactive outreach from account managers, while a segment of infrequent users might receive re-engagement campaigns focused on product education. Research from Bain & Company suggests that companies implementing advanced segmentation in their retention strategies achieve 25-95% higher profitability compared to those using one-size-fits-all approaches. The most sophisticated systems implement dynamic segmentation that continuously reassigns customers based on changing behaviors and risk factors, ensuring retention strategies remain relevant as customer relationships evolve. Our AI appointment scheduler can help maintain engagement with different customer segments through personalized outreach.
Balancing Prediction Accuracy with Explainability
While complex black-box AI models often deliver the highest prediction accuracy, they present challenges when businesses need to understand and act on their predictions. This creates a fundamental tension in churn prediction between accuracy and explainability that organizations must navigate. Highly complex models like deep neural networks and ensemble methods can capture subtle patterns in customer behavior, but their inner workings remain opaque to business users who need to implement retention strategies. More transparent approaches like decision trees and logistic regression provide clear explanations of which factors drive predictions, but may sacrifice some predictive power. The most effective solutions often implement hybrid approaches that use explainable models for day-to-day operations while leveraging more complex algorithms for periodic deep analysis. Some organizations are implementing local explainability techniques like LIME (Local Interpretable Model-agnostic Explanations) and SHAP (SHapley Additive exPlanations) that can provide insight into individual predictions from otherwise opaque models. This balance ensures that business teams can trust and act on AI-generated churn predictions. Our AI call assistant provides transparent reasoning behind its customer engagement recommendations.
Ethical Considerations in AI-Powered Customer Retention
As businesses deploy increasingly sophisticated AI tools to prevent customer churn, ethical considerations become paramount. These systems must balance effective retention with respect for customer autonomy and privacy. One key concern is data privacy compliance, as churn prediction systems process vast amounts of customer information that may be subject to regulations like GDPR and CCPA. Organizations must implement robust data governance frameworks that establish clear boundaries for how customer data can be used in prediction models. Another consideration is algorithmic bias, where AI systems might inadvertently discriminate against certain customer groups due to historical patterns in training data. Regular audits and fairness metrics help maintain ethical standards in model development. Companies must also consider intervention ethics—determining when retention efforts cross the line from helpful to manipulative. The most responsible approaches involve transparency with customers about data usage and providing genuine value in retention offers rather than exploiting behavioral weaknesses. Establishing an ethical AI framework with clear guidelines helps organizations navigate these complexities while maintaining customer trust. Our AI phone service adheres to strict ethical guidelines while helping businesses retain customers.
Integrating Churn Prediction with Customer Experience Platforms
For maximum impact, churn prediction shouldn’t exist in isolation but should integrate seamlessly with broader customer experience systems. This integration creates closed-loop processes where predictions trigger actions and outcomes inform future predictions. Successful implementations connect AI churn prediction tools with customer relationship management (CRM) platforms to provide service representatives with real-time risk scores and recommended actions during customer interactions. Integration with marketing automation systems enables triggered campaigns targeting at-risk segments with personalized retention offers. Customer success platforms use churn predictions to prioritize proactive outreach and guide account management strategies. Advanced organizations are creating unified customer data platforms (CDPs) that combine data from all customer touchpoints to provide a comprehensive view for churn prediction algorithms. The most sophisticated implementations use API-driven architectures that allow churn prediction models to exchange data with multiple systems in real-time, creating a dynamic ecosystem that continuously optimizes the customer experience. Consider using our Twilio AI assistants to establish these integrations with your existing communications infrastructure.
Measuring ROI: Quantifying the Impact of AI-Driven Retention
Demonstrating the business value of AI churn prediction requires robust measurement frameworks that capture both direct and indirect benefits. Organizations should establish clear baseline metrics before implementation to enable accurate before-and-after comparisons. Key performance indicators typically include retention rate improvements, extended customer lifetime value, and reduced acquisition costs to replace churned customers. Financial models should calculate the net present value (NPV) of retained customers to quantify long-term benefits, while also tracking intervention efficiency metrics that measure resources expended per customer saved. Companies often discover additional benefits like increased cross-sell opportunities and improved customer satisfaction scores that contribute to overall ROI. The most comprehensive measurement approaches use attribution modeling to distinguish the impact of AI-driven interventions from other retention initiatives. Regular A/B testing of retention strategies helps continuously refine both the prediction models and the interventions they trigger. A study by Forrester Research found that companies implementing AI for customer retention saw an average 10-25% reduction in churn and a 300% ROI over three years. Our AI phone consultant can help you track and measure these ROI metrics.
Case Study: Subscription-Based Streaming Service Transformation
A leading global streaming platform was struggling with subscriber retention despite offering competitive content. Their traditional retention methods relied on generic win-back offers after cancellation, resulting in only 15% customer recovery rates and significant revenue loss. After implementing an advanced AI churn prediction system, they transformed their approach to proactive retention. The solution integrated viewing data, customer support interactions, billing information, and external factors like competing service launches. Using a combination of gradient boosting machines and recurrent neural networks, the system identified at-risk subscribers with 87% accuracy 30 days before they would typically cancel. Each subscriber received a risk score and contributing factors, enabling personalized interventions. For users showing reduced engagement, the platform implemented personalized content recommendations based on historical preferences. When price sensitivity was the primary risk factor, subscribers received limited-time discount offers. The results were remarkable: a 31% reduction in overall churn rate within six months and a 22% increase in subscriber lifetime value. The company estimated a $43 million annual revenue impact from retained subscribers, with an implementation ROI exceeding 700%. Similar transformations are possible across industries using solutions like our AI voice agent.
Overcoming Implementation Challenges: From Concept to Deployment
Despite the clear benefits, many organizations struggle to move AI churn prediction from concept to operational reality. These implementation challenges can be overcome with proper planning and execution. Data integration barriers often present the first hurdle, as customer information typically resides in disconnected systems with inconsistent formats. Successful implementations begin with creating unified data pipelines that standardize and consolidate information from various sources. Organizational resistance can emerge when teams perceive AI as threatening their roles or question its reliability. Combatting this requires executive sponsorship, clear communication of benefits, and phased implementation that demonstrates value at each stage. Technical complexity challenges can be addressed by starting with simpler models that provide quick wins before progressing to more sophisticated algorithms. Organizations also face operational integration challenges when connecting prediction systems to customer-facing processes. Cross-functional implementation teams that include both data scientists and business users help bridge this gap. Finally, skills gaps can be addressed through a combination of training, strategic hiring, and partnerships with specialized vendors like Callin.io who provide ready-made AI solutions that require minimal technical expertise to deploy.
The Role of Human Intuition in AI-Augmented Retention
While AI excels at identifying patterns in vast datasets, human intuition and relationship management skills remain essential for effective customer retention. The most successful approaches combine algorithmic intelligence with human emotional intelligence in a collaborative framework. Customer-facing teams bring contextual understanding and relationship history that algorithms may miss, while AI provides data-driven insights that humans might overlook. Training staff to interpret and act on AI-generated churn predictions is crucial—they need to understand not just who is at risk but why, and how to tailor conversations accordingly. Some organizations implement human-in-the-loop systems where AI flags at-risk customers but humans make final decisions about intervention strategies. This approach leverages the strengths of both human and artificial intelligence while mitigating their respective weaknesses. Companies that strike this balance typically see 20-30% higher retention success rates than those relying exclusively on either human or AI-only approaches. As one customer success director put it: "Our AI tells us which customers need attention, but our team knows exactly what kind of attention they need." Find out how our AI call center white label solutions can help your team blend human touch with AI efficiency.
Future Trends: Where AI Churn Prediction is Heading
The field of AI-powered churn prediction continues to evolve rapidly, with several emerging trends poised to reshape how businesses approach customer retention. Predictive behavioral simulations are moving beyond simple probability scores to create detailed models of how customers might respond to different retention strategies, allowing for more precise intervention planning. Emotion AI and sentiment analysis are becoming increasingly sophisticated, enabling systems to detect subtle changes in customer satisfaction through voice patterns, text communications, and even facial expressions during video interactions. Federated learning techniques are addressing privacy concerns by allowing models to learn from customer data without that data ever leaving secure environments. Automated intervention optimization is gaining traction, with systems that can design and test thousands of retention offers to identify the most effective approach for each customer segment. Perhaps most transformative is the rise of preemptive experience design, where businesses use AI insights to reshape their entire customer journey to eliminate pain points before they trigger churn considerations. Companies that stay ahead of these trends will gain significant competitive advantages in customer retention. Our research into artificial intelligence for phone systems explores many of these emerging capabilities.
Combining Churn Prediction with Customer Acquisition Strategies
Forward-thinking organizations are finding powerful synergies between AI-driven churn prediction and customer acquisition efforts. This integrated approach creates a closed loop that improves both retention and acquisition efficiency. By analyzing patterns from churned customers, companies can refine their ideal customer profiles and acquisition targeting to focus on prospects with higher retention probability. This approach typically reduces acquisition costs by 15-25% while improving long-term customer value. Churn prediction insights also inform product development priorities by identifying features that drive retention, creating a virtuous cycle where products evolve to naturally reduce churn. Companies can leverage retention data to create lookalike acquisition models that identify prospects with characteristics similar to their most loyal customers. Some organizations are implementing predictive lifetime value modeling at the acquisition stage, allowing them to adjust acquisition spending based on predicted long-term retention. The most sophisticated approaches use unified customer journey analytics that track from first touch to loyal customer (or eventual churn), providing a comprehensive view of the entire customer lifecycle. Implementing these combined strategies requires cross-functional collaboration between marketing, sales, product, and customer success teams. Our AI sales solutions can help bridge these departmental gaps with unified customer intelligence.
Building a Customer-Centric Retention Culture Using AI Insights
Technology alone cannot solve churn problems—organizations need to build cultures where customer retention is everyone’s responsibility, supported by AI-driven insights. Successful companies make churn metrics visible throughout the organization, not just in customer service departments. They implement cross-functional retention committees that regularly review churn data and coordinate retention initiatives across departments. Customer health scores generated by AI systems become key performance indicators for multiple teams, creating shared accountability for retention outcomes. Leading organizations conduct regular retention root cause analysis sessions where cross-functional teams use AI insights to identify and address systemic issues driving customer departures. Some companies are implementing retention-based compensation structures where bonuses and incentives are tied to customer retention metrics at all organizational levels. The most advanced approaches include customer advisory boards that provide direct feedback on retention initiatives identified through AI analysis. This culture shift transforms retention from a reactive customer service function to a proactive, company-wide priority driven by data-informed decision making. Learn how our AI voice conversation technology can help spread retention insights throughout your organization.
Maximizing Customer Lifetime Value Through Predictive Engagement
The ultimate goal of churn prediction isn’t just preventing departures but maximizing the total value of each customer relationship. AI solutions are increasingly focusing on this broader objective by predicting not just who might leave but also who has expansion potential. These systems analyze customer behavior patterns to identify expansion readiness indicators that signal opportunities for upselling and cross-selling. For example, a software company might recognize that certain usage patterns predict readiness for premium features, while a financial services firm might identify life events that indicate openness to new products. By combining churn risk scores with expansion potential scores, companies create sophisticated customer opportunity matrices that guide relationship management strategies across the entire customer base. The most advanced systems implement next-best-action recommendations that continuously suggest the optimal next step for each customer relationship—whether that’s a retention offer, an expansion opportunity, or a relationship-building interaction. This predictive engagement approach has been shown to increase average customer lifetime value by 25-50% compared to traditional retention-only strategies. Organizations implementing these comprehensive approaches recognize that the best retention strategy is often providing such remarkable value that customers never consider leaving. Our AI appointment setter can help schedule these value-building interactions at precisely the right moments.
Elevate Your Retention Strategy with Callin.io’s AI Solutions
Ready to transform your approach to customer retention? The insights we’ve explored about AI-powered churn prediction are just the beginning of what’s possible with today’s technology. At Callin.io, we’ve developed sophisticated AI communication tools that help businesses identify at-risk customers and engage them through personalized, timely interactions that dramatically improve retention rates. Our platform combines advanced churn prediction algorithms with automated outreach capabilities that make retention efforts both more effective and more efficient.
If you’re looking to implement these strategies in your business, Callin.io offers a comprehensive solution for managing your business communications with AI-powered intelligence. Our platform enables you to deploy AI phone agents that can handle both inbound and outbound calls autonomously, from scheduling appointments to answering common questions and even closing sales—all while gathering valuable data for churn prediction. With our intuitive interface, you can quickly set up your AI agent, enjoy included test calls, and track all interactions through our task dashboard.
Start with a free account to explore the basics, or subscribe to one of our plans starting at just $30 per month for advanced features like Google Calendar integration and built-in CRM functionality. Don’t let customer churn continue to drain your business resources—discover how Callin.io’s AI solutions can help you build stronger, longer-lasting customer relationships today.
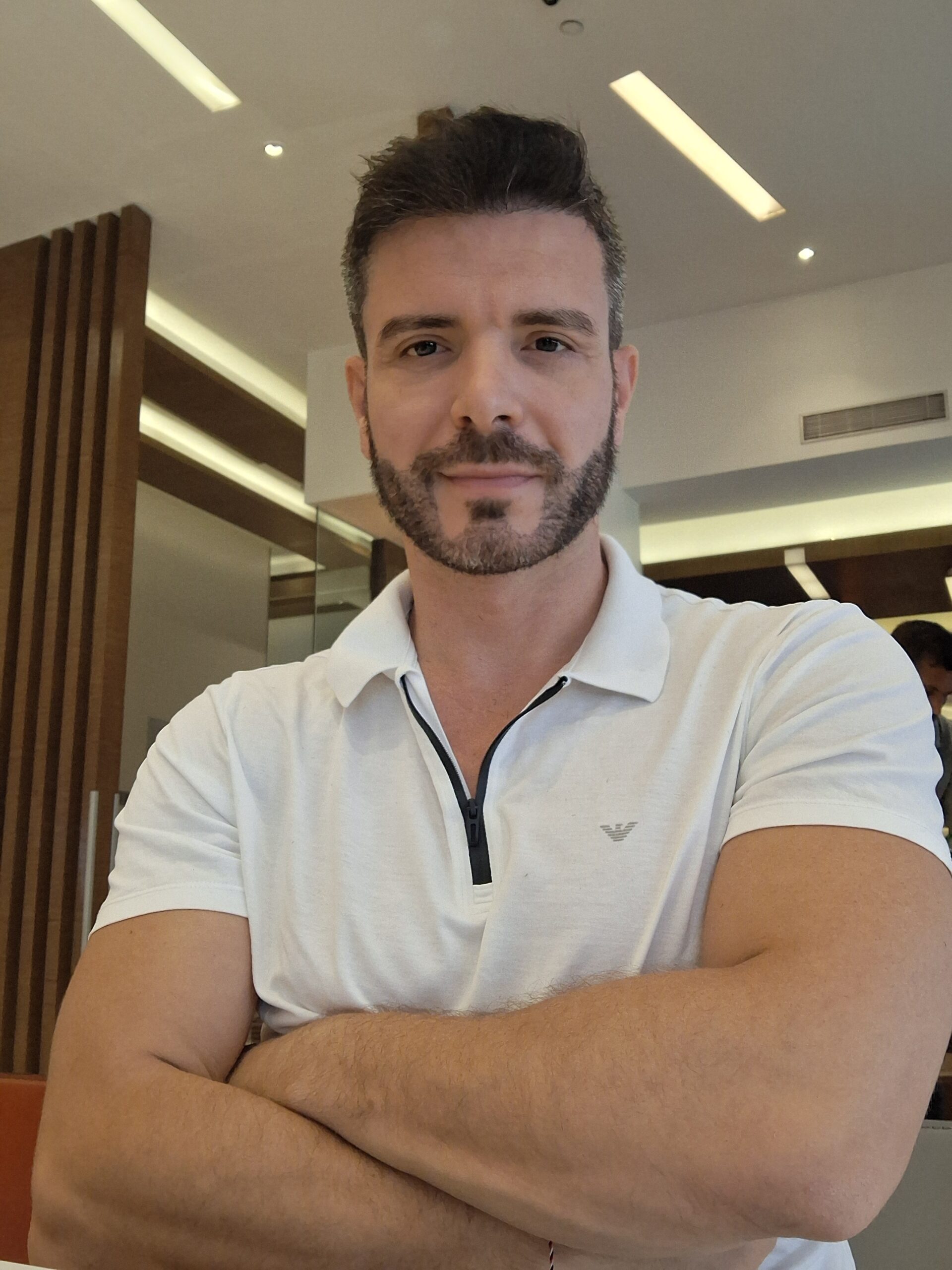
Helping businesses grow faster with AI. 🚀 At Callin.io, we make it easy for companies close more deals, engage customers more effectively, and scale their growth with smart AI voice assistants. Ready to transform your business with AI? 📅 Let’s talk!
Vincenzo Piccolo
Chief Executive Officer and Co Founder