Understanding the B2B SaaS Analytics Landscape
In today’s data-driven business environment, B2B SaaS analytics has become a critical component for companies seeking sustainable growth and competitive advantage. Unlike traditional software models, SaaS platforms generate continuous streams of valuable user data, creating unprecedented opportunities for insight extraction.
The B2B SaaS analytics ecosystem encompasses a wide range of methodologies and tools specifically designed to track, measure, and interpret how businesses interact with software services. These analytics solutions differ significantly from B2C counterparts due to longer sales cycles, complex user hierarchies, and multi-stakeholder decision processes.
According to recent industry research, companies leveraging advanced B2B SaaS analytics experience 25-30% higher revenue growth compared to those using basic reporting tools. This stark difference highlights why understanding and implementing robust analytics frameworks is no longer optional but essential for SaaS business success.
The evolution of these analytical capabilities has accelerated dramatically in recent years, with artificial intelligence transforming sales operations and creating new possibilities for data interpretation beyond human capacity. As McKinsey reports, businesses that effectively harness these advanced analytics capabilities consistently outperform market averages by significant margins.
Key Performance Indicators for B2B SaaS Success
Tracking the right metrics is fundamental to understanding your SaaS business health and optimizing for growth. While numerous KPIs exist, certain metrics stand out as particularly crucial for B2B SaaS companies.
Customer Acquisition Cost (CAC) represents the total sales and marketing expense required to acquire a new customer. This figure must be carefully balanced against Customer Lifetime Value (CLV), which projects the total revenue a customer will generate before churning. The ideal CLV:CAC ratio should exceed 3:1 for sustainable growth.
Monthly Recurring Revenue (MRR) and its variations (including expansion MRR and contracted MRR) provide critical insights into revenue stability and growth trends. These metrics help forecast cash flow with greater accuracy than traditional sales models.
Churn rate—both user and revenue—deserves special attention as it directly impacts long-term viability. For enterprise-focused B2B SaaS, annual churn rates above 5-7% often signal serious product-market fit issues requiring immediate attention.
Implementing these metrics effectively often requires specialized tools that integrate with your existing tech stack. Many companies find success with platforms like Baremetrics or ChartMogul, while others develop custom dashboards using business intelligence tools like Tableau or Power BI. For companies already using advanced communication systems like AI-powered sales tools, integration with analytics platforms can provide even deeper customer insights.
Building Your SaaS Analytics Framework
Developing a comprehensive analytics framework requires thoughtful planning and execution. The process begins with identifying your specific business objectives and the questions you need to answer through data analysis. Without this clarity, even the most sophisticated analytics tools will fail to deliver actionable insights.
Start by mapping your customer journey—from initial awareness to purchase decision and ongoing usage patterns. Each stage offers valuable data collection opportunities that, when properly analyzed, reveal optimization potential. This approach aligns with modern conversational AI strategies that personalize customer interactions.
Your framework should include both quantitative metrics (like conversion rates and engagement scores) and qualitative feedback mechanisms. The combination provides a more complete picture of customer behavior and satisfaction than either approach alone.
Data integration represents a common challenge when building analytics frameworks. Many B2B SaaS companies operate with disparate systems—CRM, marketing automation, billing, and product usage tools—each generating valuable but isolated data. Solutions like Segment or Zapier can help create a unified data layer, while more advanced options include customer data platforms (CDPs) that create comprehensive user profiles from multiple sources.
When designing your framework, plan for scalability from the beginning. What works for analyzing 100 customers will likely break down at 1,000 or 10,000. Cloud-based analytics infrastructures like those offered by AWS or Google Cloud provide the necessary flexibility to grow with your business needs.
Product Analytics for SaaS Optimization
Product analytics reveals how users interact with your software, highlighting both successful features and improvement opportunities. Unlike marketing or sales analytics, product metrics focus specifically on in-app behavior patterns that directly influence retention and expansion revenue.
Feature adoption rates help identify which capabilities drive value for customers. Low adoption of key features often predicts future churn, while high engagement with specific tools can guide product development priorities and expansion opportunities.
Session metrics—including frequency, duration, and flow patterns—reveal how deeply users engage with your platform. Many B2B SaaS companies find that Mixpanel or Amplitude provide excellent visualization of these user journeys, making pattern identification more intuitive for product teams.
User segmentation amplifies the value of product analytics by identifying different usage patterns across customer segments. Enterprise clients may leverage entirely different features than small business users, requiring tailored retention strategies for each segment. This approach parallels how AI tools for resellers adapt to different business contexts.
Implementing effective product analytics requires thoughtful instrumentation of your application. Each significant user action should trigger event tracking, creating a comprehensive dataset for analysis. While this process requires development resources upfront, the resulting insights typically deliver substantial ROI through improved retention and expansion revenue.
Customer Success Analytics and Retention Strategies
Customer success analytics focuses on identifying at-risk accounts before they churn and recognizing expansion opportunities within your existing customer base. This proactive approach transforms customer success from a reactive support function to a strategic growth driver.
Health scores aggregate multiple signals—including product usage, support interactions, NPS responses, and contract details—to predict account risk levels. Sophisticated models weight these factors differently based on their predictive power for your specific business context.
Engagement trends over time often provide early warning signals before traditional churn indicators appear. Declining login frequency, reduced feature usage, or decreasing active users within an account typically precede formal cancellation requests by weeks or months.
Expansion analytics identifies accounts ready for additional seats, features, or premium tiers. Signals may include consistently high usage, users bumping against current plan limitations, or positive sentiment in customer communications. Tools like Gainsight or CustomerSuccess.io help track these indicators systematically.
The predictive power of retention analytics has been significantly enhanced by machine learning algorithms that identify subtle churn patterns human analysts might miss. Companies that implement these advanced analytics approaches, similar to AI phone consultants, report identifying 35-40% more at-risk accounts than traditional methods, creating intervention opportunities that substantially improve overall retention rates.
Sales and Marketing Analytics for SaaS Growth
Sales and marketing analytics illuminate the effectiveness of your customer acquisition strategies, enabling more efficient resource allocation and continuous refinement of messaging and targeting. For B2B SaaS companies with limited marketing budgets, these insights can dramatically improve ROI on acquisition spending.
Attribution modeling tracks which channels and campaigns generate qualified leads and closed deals. Multi-touch attribution provides particular value for B2B SaaS companies with longer sales cycles involving numerous touchpoints. Tools like Bizible or Attributer help implement these sophisticated models without excessive technical complexity.
Funnel analytics measure conversion rates between pipeline stages, identifying specific points where prospects disengage. This granular view allows for targeted improvements rather than wholesale changes to acquisition strategies.
Lead scoring models use behavioral and demographic data to predict which prospects are most likely to convert, helping sales teams prioritize their outreach efforts. Advanced systems incorporate machine learning to continuously refine scoring accuracy based on conversion outcomes, similar to how AI calling agents adapt their approaches based on previous interactions.
Competitive intelligence analytics track market positioning and messaging shifts from key competitors, enabling proactive response to emerging threats or opportunities. Tools like Crayon or Klue automate much of this monitoring, allowing marketing teams to focus on strategic responses rather than manual data collection.
Financial Analytics for SaaS Business Models
Financial analytics adapted specifically for subscription-based models provides critical insights beyond traditional accounting metrics. These specialized measures help SaaS executives understand business performance through a lens that accounts for deferred revenue recognition and subscriber economics.
Unit economics analysis examines profitability at the individual customer level, accounting for acquisition costs, service expenses, and lifetime revenue. This granular view helps identify which customer segments generate the highest returns and which may actually destroy value despite contributing to topline growth.
Cohort analysis tracks how customer value evolves over time by grouping customers who joined during specific periods. This approach reveals whether your product and customer success initiatives are improving retention and expansion revenue over successive customer generations.
Cash flow projection models are particularly important for SaaS businesses due to the mismatch between upfront acquisition expenses and distributed subscription revenue. Accurate forecasting helps prevent cash constraints during periods of rapid growth when acquisition costs temporarily outpace recognized revenue.
Advanced financial analytics platforms like ProfitWell or ChartMogul can automatically generate these subscription-specific financial metrics, integrating directly with billing systems to ensure accuracy without manual data entry. For companies implementing AI phone systems or similar technologies, these financial analytics tools help quantify the ROI of such investments.
Customer Journey Analytics for B2B SaaS
Customer journey analytics maps the entire relationship lifecycle from initial awareness through purchase, onboarding, regular usage, and renewal or expansion. This holistic view reveals friction points and opportunities that might be missed when examining individual interaction channels separately.
Touchpoint analysis identifies every significant interaction between customers and your company across marketing, sales, product usage, and support channels. Visualizing these interactions chronologically helps identify patterns that influence conversion and retention outcomes.
Time-to-value measurement tracks how quickly new customers achieve their first meaningful success with your product. Reducing this interval dramatically improves early retention, as customers who quickly experience value are significantly less likely to churn during the critical first 90 days.
Cross-channel experience consistency becomes increasingly important as customers interact with your brand through multiple touchpoints. Inconsistent messaging or service quality between channels often damages brand perception and reduces trust. Analytics platforms like Amplitude or Woopra can track these cross-channel journeys effectively.
For B2B SaaS companies serving enterprise clients, journey analytics must account for multiple stakeholders within each account, each with different priorities and usage patterns. This complexity requires more sophisticated tracking and analysis than typical B2C customer journeys, but delivers proportionally greater insights when implemented effectively.
Predictive Analytics and Machine Learning Applications
Predictive analytics leverages historical data patterns to forecast future outcomes, enabling proactive decision-making rather than reactive responses. The B2B SaaS context offers particularly rich opportunities for these techniques due to the wealth of structured data generated through normal business operations.
Churn prediction models identify accounts likely to cancel before explicit cancellation signals appear, creating intervention windows for customer success teams. Advanced models incorporate diverse signals including product usage patterns, support interactions, contract details, and even macroeconomic indicators for the client’s industry.
Upsell opportunity forecasting helps sales teams focus expansion efforts on accounts with the highest probability of converting. These models typically examine usage intensity, feature adoption patterns, and growth signals within the customer’s organization.
Lead scoring sophistication has increased dramatically through machine learning implementations that continuously refine predictive accuracy based on actual conversion outcomes. Modern systems can identify subtle interaction patterns that strongly correlate with purchase intent but might not be obvious to human analysts.
Implementing these predictive capabilities has become more accessible through platforms like DataRobot or Amazon SageMaker that provide user-friendly interfaces for developing machine learning models without requiring deep data science expertise. This democratization of advanced analytics parallels similar trends in AI voice assistants and other previously specialized technologies.
Real-Time Analytics and Operational Dashboards
Real-time analytics provides immediate feedback on key metrics, enabling rapid response to emerging issues or opportunities. While not all SaaS metrics require real-time monitoring, certain operational measures benefit tremendously from this immediacy.
System performance metrics should be monitored continuously to identify potential service degradation before it impacts customers. Tracking response times, error rates, and infrastructure utilization helps maintain the reliability that B2B customers particularly value.
User engagement patterns often reveal immediate feedback on new features or interface changes. Sudden drops in specific feature usage may indicate usability problems requiring quick intervention.
Conversion funnel activity benefits from real-time visibility during promotional campaigns or after significant product updates. This immediate feedback allows for quick adjustments if conversion rates differ significantly from expectations.
Implementing effective real-time dashboards requires careful consideration of which metrics truly benefit from immediate visibility versus those better viewed through longer timeframes. Tools like Datadog or New Relic provide excellent real-time monitoring capabilities specifically designed for SaaS applications, while business intelligence platforms like Looker or Power BI offer more customizable dashboard creation for business metrics.
Privacy and Compliance in SaaS Analytics
Privacy considerations and regulatory compliance have become increasingly important aspects of SaaS analytics implementation. With regulations like GDPR, CCPA, and industry-specific requirements like HIPAA, analytics strategies must balance insight generation with privacy protection.
Data collection policies should clearly specify what information is gathered and how it will be used. Transparency builds trust with customers and reduces regulatory risk. Many B2B SaaS companies now provide detailed analytics disclosure statements as part of their terms of service.
Data minimization principles suggest collecting only information with clear analytical value rather than accumulating data without specific purposes. This approach reduces both compliance risk and storage costs.
Anonymization and aggregation techniques help protect individual privacy while still enabling valuable analysis. For example, user flows can be analyzed in aggregate without maintaining individually identifiable records of specific users’ actions.
International data transfers require particular attention for companies serving global markets. Analytics architectures may need region-specific data storage and processing to comply with local regulations, similar to how SIP trunking solutions must address regional telecommunications regulations.
Data Visualization for Decision Making
Effective data visualization transforms complex analytics into accessible insights that drive action. For B2B SaaS companies, the right visualizations can dramatically improve how teams interpret and respond to performance metrics.
Executive dashboards should present high-level KPIs with clear trending information, enabling quick assessment of business health. These interfaces typically focus on financial metrics, growth indicators, and retention measures relevant to strategic decision-making.
Team-specific visualizations deliver more granular metrics relevant to each functional area. Product teams benefit from detailed feature usage and engagement patterns, while marketing requires campaign performance and attribution visualizations.
Interactive exploration tools allow users to investigate data patterns beyond predefined reports. This capability is particularly valuable for answering emerging questions without requiring analyst intervention. Modern tools like Tableau or Power BI provide sophisticated drill-down capabilities accessible to business users.
Narrative visualization approaches that combine data with explanatory context often prove most effective for driving organizational change. Rather than presenting isolated metrics, these approaches tell coherent stories about customer behavior or business performance that motivate specific actions.
Implementing A/B Testing in SaaS Products
A/B testing (or split testing) methodologies enable data-driven decision making by comparing how different versions of features, interfaces, or workflows perform with actual users. This empirical approach reduces subjective debates about product changes by providing concrete performance data.
Feature testing compares different implementations of product capabilities to determine which delivers better engagement or outcome metrics. This approach is particularly valuable for core features where small efficiency improvements can significantly impact overall product value.
Pricing and packaging experiments help optimize revenue by testing how different offerings impact conversion rates and average contract values. For B2B SaaS with tiered pricing models, these tests can reveal surprising price elasticity patterns across different market segments.
Onboarding flow optimization through systematic testing often yields dramatic improvements in activation rates and time-to-value. Since onboarding represents a critical moment in the customer journey, even modest improvements can substantially impact overall business performance.
Implementing effective testing programs requires both technical infrastructure and organizational discipline. Tools like Optimizely or LaunchDarkly provide the necessary technical capabilities, while clear statistical standards and decision protocols ensure test results drive actual product decisions.
Building an Analytics-Driven Culture
Creating an organization that consistently leverages analytics for decision making requires more than just implementing the right tools. Cultural transformation is needed to ensure data actually influences strategic and operational choices.
Leadership alignment on the value of data-driven decisions provides essential foundation for analytics adoption. When executives consistently request data to support recommendations and visibly base their own decisions on analytics, the approach cascades throughout the organization.
Accessibility of insights to all relevant stakeholders democratizes data-informed decision making. Modern analytics platforms offer role-appropriate dashboards and self-service capabilities that eliminate bottlenecks created when all analytics requests must flow through dedicated analysts.
Training programs to develop analytical thinking across functions help employees effectively interpret and apply data to their specific responsibilities. These skills are increasingly considered core competencies rather than specialized technical abilities.
Celebration of data-driven successes reinforces the value of analytics approaches. Recognizing teams that leverage data effectively to improve outcomes creates positive examples that encourage wider adoption, similar to how success stories help drive adoption of AI phone agents and other innovative technologies.
Analytics Integration with Your SaaS Tech Stack
Effective analytics implementation requires thoughtful integration with your existing technology infrastructure. Isolated analytics solutions that don’t connect with other business systems create data silos that limit insight potential.
CRM integration ensures customer interaction data flows seamlessly into your analytics platform, enabling correlation between sales activities and outcomes. Popular CRM systems like Salesforce or HubSpot offer robust API connections to most analytics platforms.
Product telemetry systems capture detailed usage data directly from your application, providing visibility into feature adoption and engagement patterns. These implementations often leverage event-tracking libraries like Segment or Snowplow that standardize data collection.
Marketing automation platform connections unify campaign performance data with downstream customer behaviors, enabling true multi-touch attribution. These integrations help marketing teams understand which efforts genuinely influence pipeline development and closed deals.
Data warehouse implementation provides the foundation for comprehensive analytics by centralizing information from disparate sources. Modern cloud data warehouses like Snowflake or BigQuery offer scalable performance that grows with your data volume needs.
Advanced Analytics Techniques for SaaS Growth
As your analytics capabilities mature, advanced techniques can unlock deeper insights and competitive advantages. These sophisticated approaches build upon foundational analytics to reveal patterns and opportunities invisible to basic reporting.
Cohort analysis beyond simple retention metrics examines how customer behaviors evolve across different acquisition groups. This technique reveals whether product improvements are actually increasing engagement and value for newer customers compared to earlier cohorts.
Multivariate testing expands beyond simple A/B comparisons to examine interactions between multiple variables simultaneously. This approach is particularly valuable for complex product experiences where numerous elements influence user success.
Customer segmentation using machine learning identifies natural groupings based on behavior patterns rather than predetermined characteristics. These emergent segments often reveal surprising customer types that wouldn’t be discovered through traditional demographic or firmographic segmentation.
Sentiment analysis applied to support interactions, NPS comments, and other qualitative customer feedback helps quantify customer attitudes that influence retention and expansion. Modern natural language processing techniques achieve increasingly accurate sentiment classification, enabling large-scale analysis previously impossible with manual review.
Transforming Your B2B SaaS with Intelligence-Driven Strategies
Transforming your SaaS business through analytics requires systematic application of insights to strategic and operational decisions. The most successful companies establish clear processes for translating data into action.
Growth experimentation frameworks create structured approaches to testing new acquisition, activation, and retention strategies based on analytical insights. These frameworks typically include hypothesis formation, experiment design, implementation, and result analysis phases.
Product roadmap prioritization informed by usage analytics ensures development resources focus on features with the highest potential impact on customer value and retention. This data-driven approach often challenges assumptions about what customers actually value most.
Customer success intervention protocols triggered by analytical risk indicators create consistent responses to potential churn signals. These systematic approaches ensure timely action when analytics identify accounts requiring attention.
The most sophisticated analytics implementations ultimately move beyond descriptive measures to prescriptive guidance—not just reporting what has happened but recommending specific actions based on predictive models and business rules. This evolution parallels how conversational AI has progressed from simple response systems to proactive engagement tools.
Elevate Your SaaS Analytics with Integrated Communication Solutions
As you develop your B2B SaaS analytics strategy, consider how enhanced communication capabilities can both generate valuable data and help you act on analytical insights. Modern business requires seamless integration between analytics systems and customer interaction channels.
For organizations seeking to maximize the value of customer conversations, Callin.io offers AI-powered phone agents that not only handle calls autonomously but also generate rich interaction data that feeds directly into your analytics ecosystem. This integration provides unprecedented visibility into customer needs, concerns, and satisfaction levels expressed through voice conversations.
With Callin.io’s AI phone agents, you can automatically schedule appointments, answer common questions, and even close sales—all while capturing structured data from every interaction. The platform’s natural language processing capabilities ensure conversations flow naturally while simultaneously extracting actionable insights.
Getting started is straightforward with Callin.io’s free account, which includes a user-friendly interface, test calls, and a comprehensive task dashboard for monitoring interactions. For businesses requiring advanced features like Google Calendar integration and built-in CRM functionality, paid plans start at just $30 monthly.
By combining robust analytics with intelligent communication systems, you create a powerful feedback loop: analytics guide your customer engagement strategy, while AI-powered communications generate new data that further refines your analytics. Explore Callin.io today to see how intelligent communication can enhance your B2B SaaS analytics capabilities.
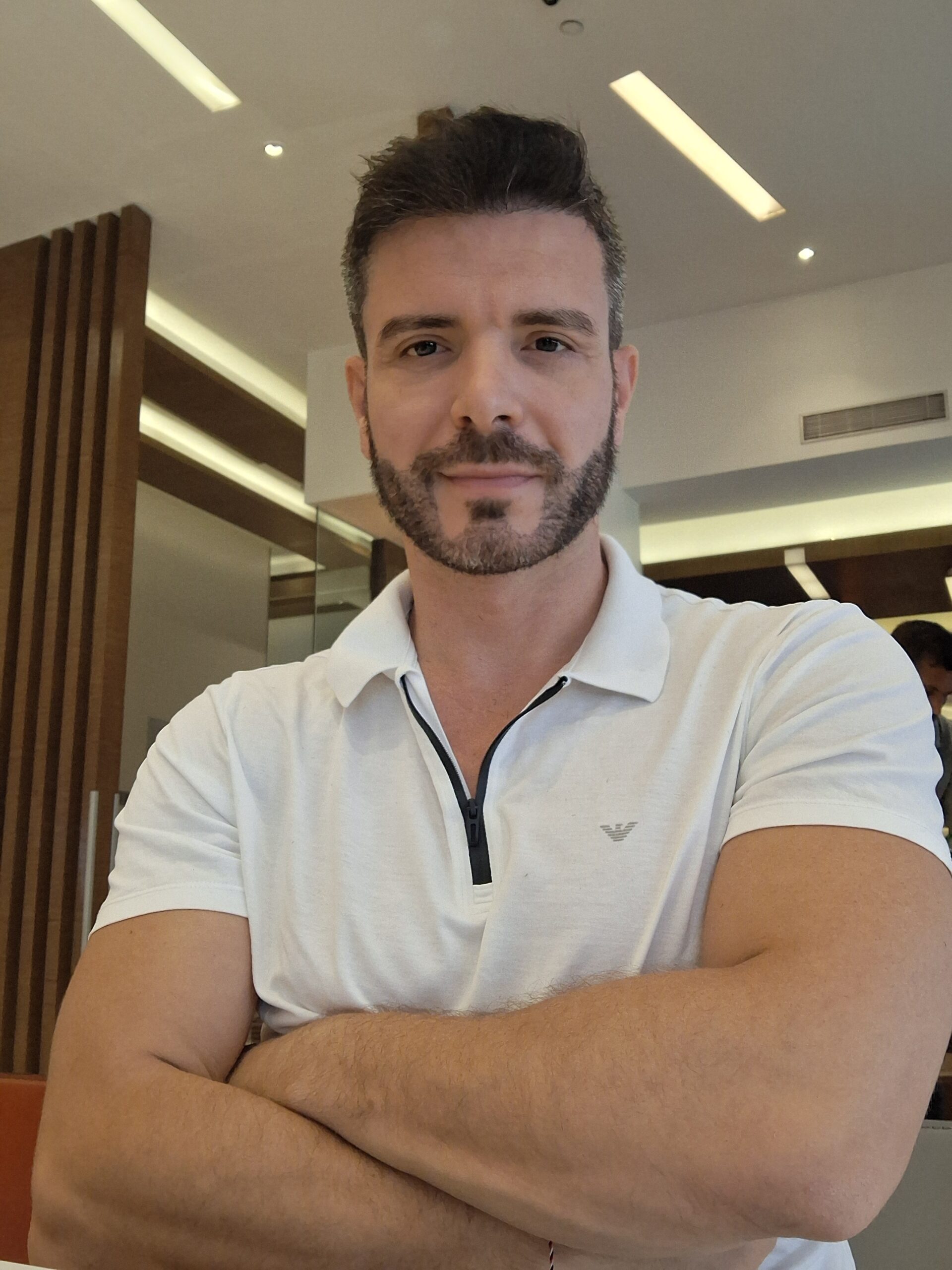
Helping businesses grow faster with AI. 🚀 At Callin.io, we make it easy for companies close more deals, engage customers more effectively, and scale their growth with smart AI voice assistants. Ready to transform your business with AI? 📅 Let’s talk!
Vincenzo Piccolo
Chief Executive Officer and Co Founder